Comparative Effectiveness of Risankizumab Vs. Ustekinumab in Bio-Naïve Crohn's Disease Patients: A Real-World Multicentre Retrospective Cohort Study
JOURNAL OF CROHNS & COLITIS(2025)
Washington Univ St Louis | Cedars Sinai Med Ctr | Nancy Univ Hosp | Mayo Clin | Icahn School of Medicine at Mt. Sinai | Washington Univ
Abstract
Abstract Background The SEQUENCE trial, in bio-exposed Crohn's disease (CD) patients, demonstrated non-inferiority for clinical remission at 24 weeks and superiority for endoscopic remission at week 48 for risankizumab (RZB) compared to ustekinumab (UST). However, this comparison has not been adequately explored in bio-naive CD patients. Our study aimed to compare the clinical effectiveness among bio-naive CD patients treated with RZB vs. UST in a real-world setting, using data from a US-based multicenter cohort. Methods This retrospective cohort study utilized data from the TriNetX US Collaborative Network, comprising sixty-eight tertiary institutions, to examine adult (≥18 years) bio-naive CD patients initiated on RZB or UST from June 17th, 2022 (FDA approval date) to October 31st, 2024. Patients with prior advanced therapy usage and those with approved rheumatologic disease indications for RZB/UST were excluded. A 1:1 propensity score matching (PSM) analysis was conducted to balance cohorts on demographics, baseline comorbidities, and indicators of CD severity, including CD phenotype, extraintestinal manifestations, concurrent corticosteroid use, hemoglobin, and body weight. Cox proportional hazards models estimated hazard ratios (HRs) in matched cohorts for one-year post-treatment composite outcomes (need for corticosteroids, hospitalizations, emergency room visits, surgeries) with a priori significance threshold of p < 0.05. Results The study sample included 1,630 bio-naive CD patients treated with RZB and 3,105 with UST. Among patients on UST, 658 (21%) were dosed every four to six weeks, after the first dose. Baseline characteristics of the PSM RZB and UST cohorts are shown in Table 1 with 1,568 patients in each cohort. After PSM (Figure 1), there were no significant differences in composite outcome (HR 1.077, p = 0.170), corticosteroid use (HR 1.099, p = 0.110), CD-related surgery (HR 1.231, p = 0.284), hospitalization (HR 0.947, p = 0.615) or ER visits (HR 1.041, p = 0.687). Additionally, no significant differences were observed in opioid use (HR 1.062, p = 0.366), switch to different advanced therapy (HR 1.097, p = 0.468) or elevated disease biomarkers, including CRP (>10mg/l, HR 1.186 p = 0.101) or fecal calprotectin (>150 µg/g; HR 1.109, p = 0.407) at 1-year follow-up. Conclusion In this real-world experience allowing for drug optimization, no difference was observed in bio naïve patients between UST and RZB as far as clinical remission and progression of CD.
MoreTranslated text
求助PDF
上传PDF
View via Publisher
AI Read Science
AI Summary
AI Summary is the key point extracted automatically understanding the full text of the paper, including the background, methods, results, conclusions, icons and other key content, so that you can get the outline of the paper at a glance.
Example
Background
Key content
Introduction
Methods
Results
Related work
Fund
Key content
- Pretraining has recently greatly promoted the development of natural language processing (NLP)
- We show that M6 outperforms the baselines in multimodal downstream tasks, and the large M6 with 10 parameters can reach a better performance
- We propose a method called M6 that is able to process information of multiple modalities and perform both single-modal and cross-modal understanding and generation
- The model is scaled to large model with 10 billion parameters with sophisticated deployment, and the 10 -parameter M6-large is the largest pretrained model in Chinese
- Experimental results show that our proposed M6 outperforms the baseline in a number of downstream tasks concerning both single modality and multiple modalities We will continue the pretraining of extremely large models by increasing data to explore the limit of its performance
Upload PDF to Generate Summary
Must-Reading Tree
Example
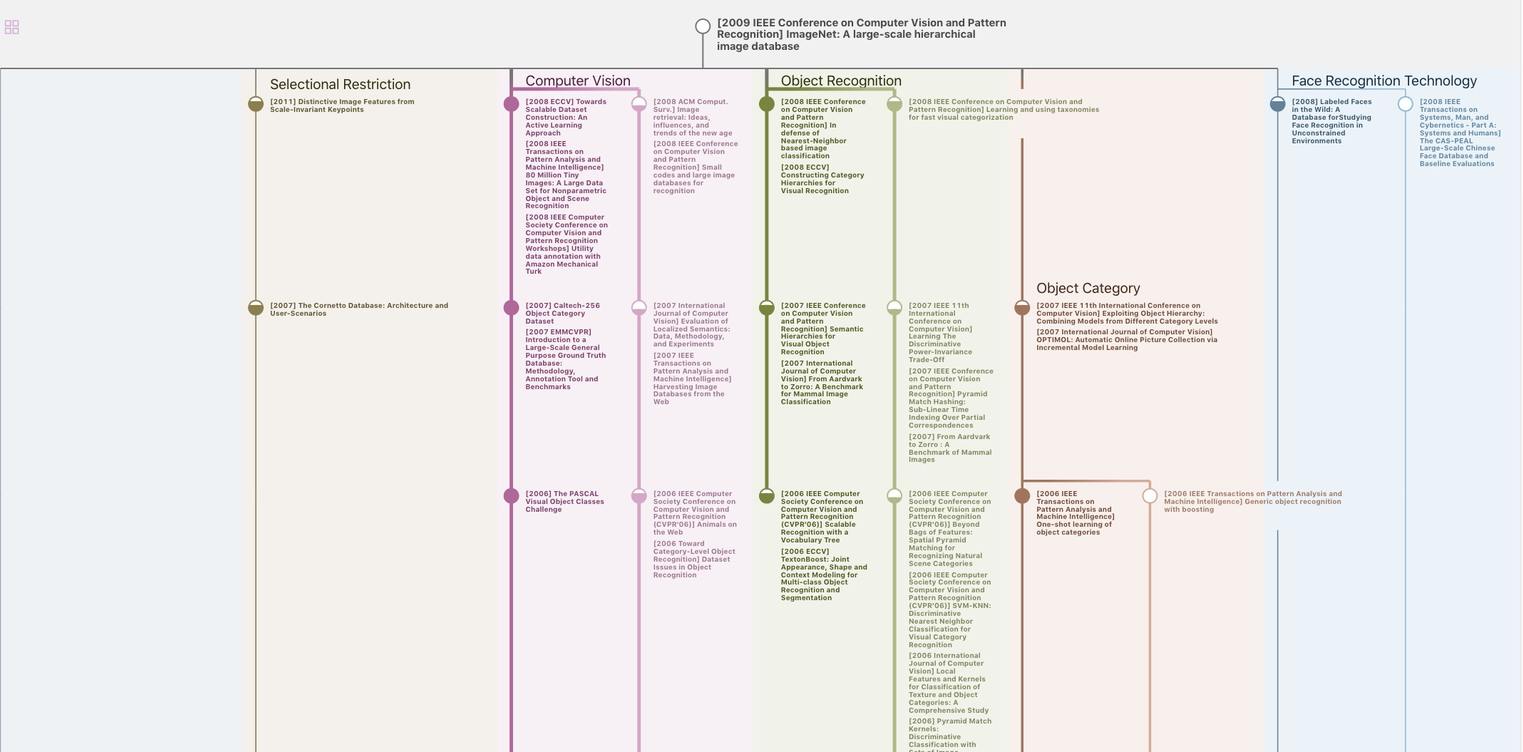
Generate MRT to find the research sequence of this paper
Data Disclaimer
The page data are from open Internet sources, cooperative publishers and automatic analysis results through AI technology. We do not make any commitments and guarantees for the validity, accuracy, correctness, reliability, completeness and timeliness of the page data. If you have any questions, please contact us by email: report@aminer.cn
Chat Paper
GPU is busy, summary generation fails
Rerequest