The AGN Optical Variability Fundamental Plane
arXiv · Astrophysics of Galaxies(2025)
Abstract
We investigate the relationship between AGN optical variability timescales, amplitudes, and supermassive black hole (SMBH) masses using homogeneous light curves from the All-Sky Automated Survey for SuperNovae (ASAS-SN). We fit a damped random walk (DRW) model to high-cadence, long-baseline ASAS-SN light curves to estimate the characteristic variability timescale (τ_DRW) and amplitude (σ) for 57 AGN with precise SMBH mass measurements from reverberation mapping and dynamical methods. We confirm a significant correlation between τ_DRW and SMBH mass, and find: log_10(M_BH/ M_⊙) = (1.85±0.20)×log_10 (τ_DRW/200 days)+7.59±0.08. Incorporating σ̂^2 = 2σ^2/τ_DRW in a plane model significantly improves residuals, and we find: log_10(M_BH/ M_⊙) = (2.27±0.20)×log_10 (τ_DRW/200 days)+(1.20±0.20)×log_10(σ̂/1 mJy/days^1/2)+7.68±0.08 with a scatter of 0.39 dex. We calculate τ_DRW, σ̂, and estimate SMBH masses for 203 bright (V<16 mag) AGN from the Milliquas catalog and compare these estimates with measurements from the BAT AGN Spectroscopic Survey for 42 overlapping AGN. In 10 years, LSST could extend this method to survey 7≲log_10(M_BH/M_⊙)≲9 SMBHs out to z∼1 and log_10(M_BH/M_⊙)∼8.0 out to z∼4, and ASAS-SN could probe 5≲log_10(M_BH/M_⊙)≲10.5 SMBHs in the local universe and log_10(M_BH/M_⊙)∼9.0 out to z∼2. Measuring AGN variability with these datasets will provide a unique probe of SMBH evolution by making estimates of M_BH spanning several orders of magnitude with photometric observations alone.
MoreTranslated text
PDF
View via Publisher
AI Read Science
AI Summary
AI Summary is the key point extracted automatically understanding the full text of the paper, including the background, methods, results, conclusions, icons and other key content, so that you can get the outline of the paper at a glance.
Example
Background
Key content
Introduction
Methods
Results
Related work
Fund
Key content
- Pretraining has recently greatly promoted the development of natural language processing (NLP)
- We show that M6 outperforms the baselines in multimodal downstream tasks, and the large M6 with 10 parameters can reach a better performance
- We propose a method called M6 that is able to process information of multiple modalities and perform both single-modal and cross-modal understanding and generation
- The model is scaled to large model with 10 billion parameters with sophisticated deployment, and the 10 -parameter M6-large is the largest pretrained model in Chinese
- Experimental results show that our proposed M6 outperforms the baseline in a number of downstream tasks concerning both single modality and multiple modalities We will continue the pretraining of extremely large models by increasing data to explore the limit of its performance
Try using models to generate summary,it takes about 60s
Must-Reading Tree
Example
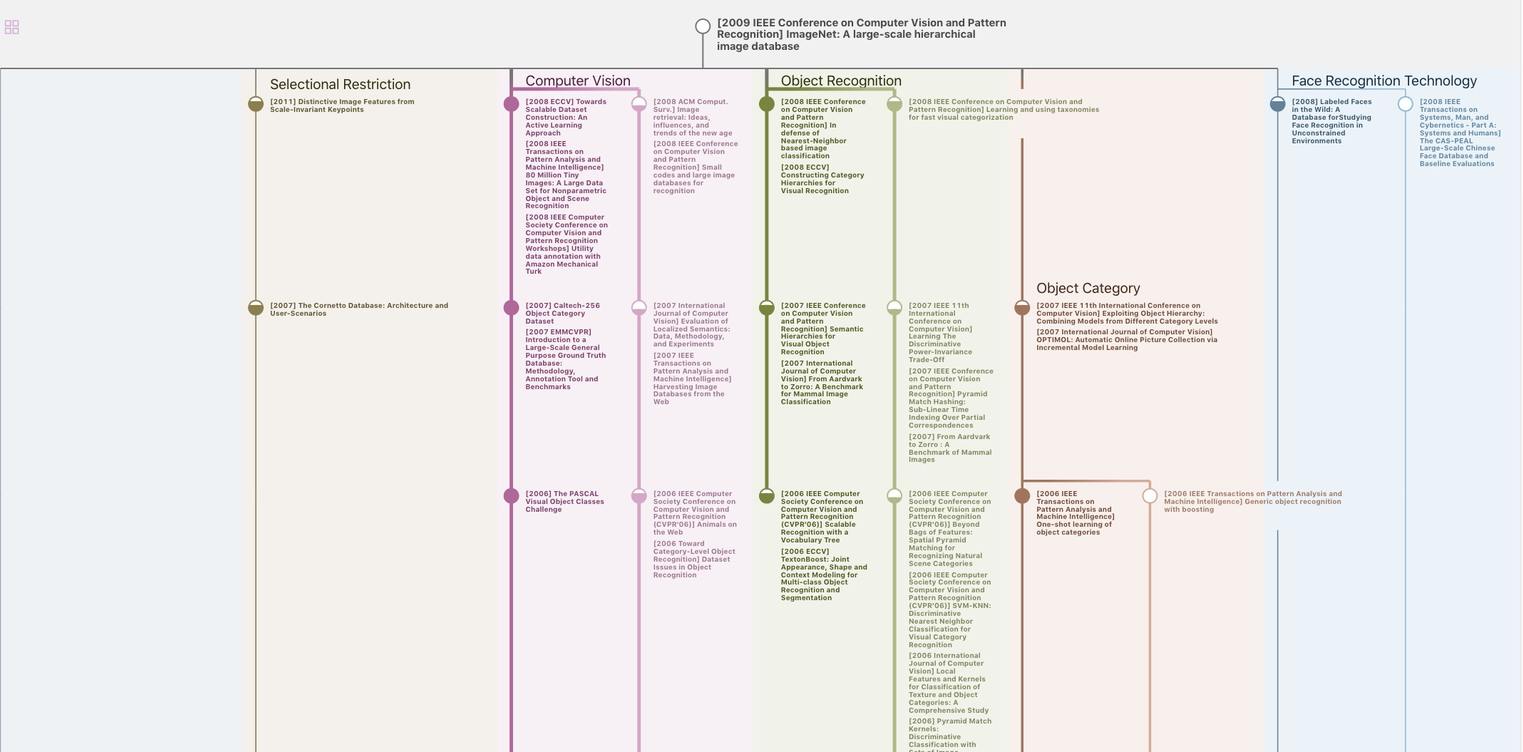
Generate MRT to find the research sequence of this paper
Data Disclaimer
The page data are from open Internet sources, cooperative publishers and automatic analysis results through AI technology. We do not make any commitments and guarantees for the validity, accuracy, correctness, reliability, completeness and timeliness of the page data. If you have any questions, please contact us by email: report@aminer.cn
Chat Paper
GPU is busy, summary generation fails
Rerequest