BANNMDA: a Computational Model for Predicting Potential Microbe-Drug Associations Based on Bilinear Attention Networks and Nuclear Norm Minimization
FRONTIERS IN MICROBIOLOGY(2025)
Hunan Vocat Coll Sci & Technol | Hunan Vocat Coll Elect & Technol | Changsha Univ
Abstract
IntroductionPredicting potential associations between microbes and drugs is crucial for advancing pharmaceutical research and development. In this manuscript, we introduced an innovative computational model named BANNMDA by integrating Bilinear Attention Networks(BAN) with the Nuclear Norm Minimization (NNM) to uncover hidden connections between microbes and drugs.MethodsIn BANNMDA, we initially constructed a heterogeneous microbe-drug network by combining multiple drug and microbe similarity metrics with known microbe-drug relationships. Subsequently, we applied both BAN and NNM to compute predicted scores of potential microbe-drug associations. Finally, we implemented 5-fold cross-validation frameworks to evaluate the prediction performance of BANNMDA.Results and discussionThe experimental results indicated that BANNMDA outperformed state-of-the-art competitive methods. We conducted case studies on well-known drugs such as the Amoxicillin and Ceftazidime, as well as on pathogens such as Bacillus cereus and Influenza A virus, to further evaluate the efficacy of BANNMDA, and experimental outcomes showed that there were 9 out of the top 10 predicted drugs, along with 8 and 9 out of the top 10 predicted microbes having been corroborated by relevant literatures. These findings underscored the capability of BANNMDA to achieve commendable predictive accuracy.
MoreTranslated text
Key words
computational model,microbe-drug associations,bilinear attention networks,nuclear norm minimization,prediction
求助PDF
上传PDF
View via Publisher
AI Read Science
AI Summary
AI Summary is the key point extracted automatically understanding the full text of the paper, including the background, methods, results, conclusions, icons and other key content, so that you can get the outline of the paper at a glance.
Example
Background
Key content
Introduction
Methods
Results
Related work
Fund
Key content
- Pretraining has recently greatly promoted the development of natural language processing (NLP)
- We show that M6 outperforms the baselines in multimodal downstream tasks, and the large M6 with 10 parameters can reach a better performance
- We propose a method called M6 that is able to process information of multiple modalities and perform both single-modal and cross-modal understanding and generation
- The model is scaled to large model with 10 billion parameters with sophisticated deployment, and the 10 -parameter M6-large is the largest pretrained model in Chinese
- Experimental results show that our proposed M6 outperforms the baseline in a number of downstream tasks concerning both single modality and multiple modalities We will continue the pretraining of extremely large models by increasing data to explore the limit of its performance
Upload PDF to Generate Summary
Must-Reading Tree
Example
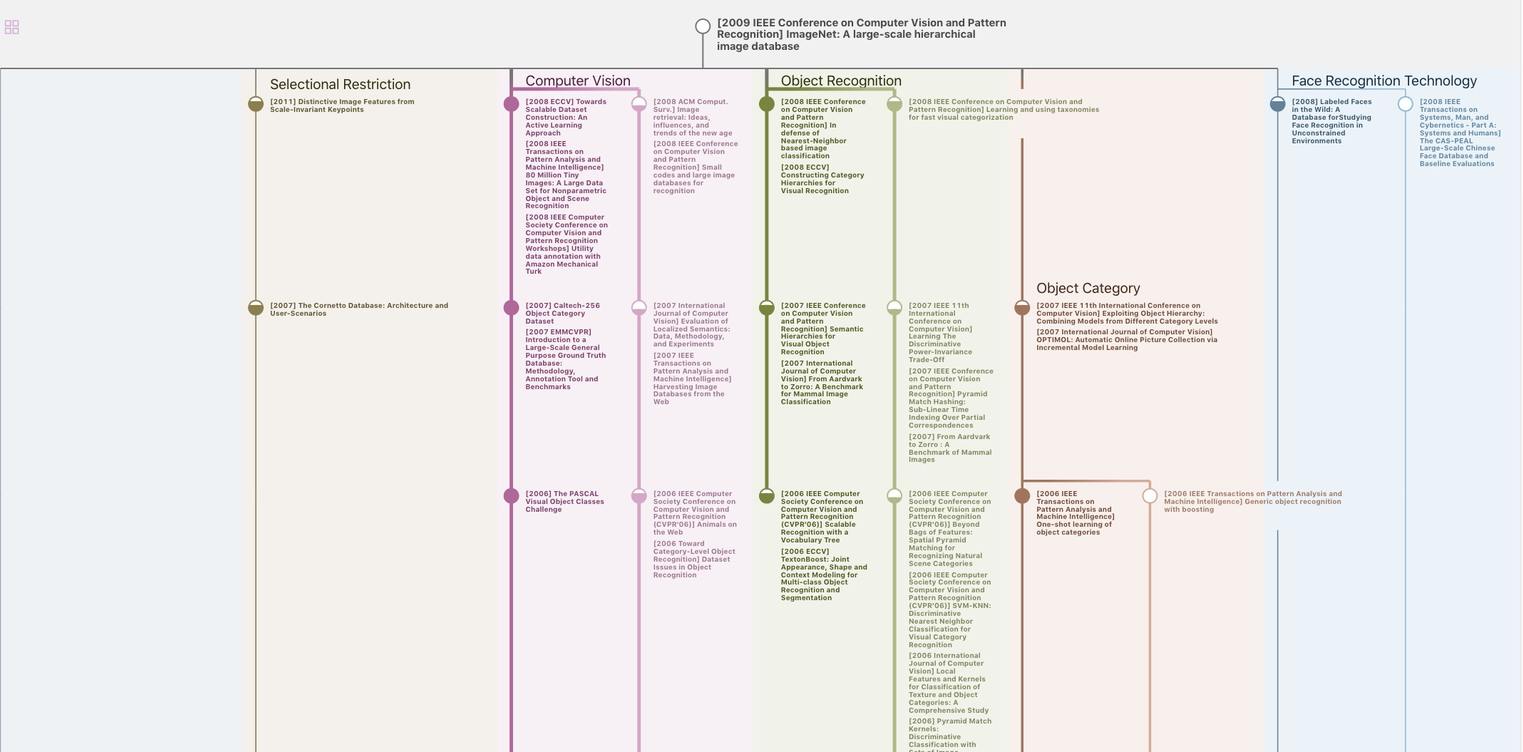
Generate MRT to find the research sequence of this paper
Data Disclaimer
The page data are from open Internet sources, cooperative publishers and automatic analysis results through AI technology. We do not make any commitments and guarantees for the validity, accuracy, correctness, reliability, completeness and timeliness of the page data. If you have any questions, please contact us by email: report@aminer.cn
Chat Paper