Associations Between Long-Term Exposure to Fine Particulate Matter and Its Constituents with Lung Cancer Incidence: Evidence from a Prospective Cohort Study in Beijing, China
ENVIRONMENTAL POLLUTION(2025)
Peking Univ | Advanced Institute of Information Technology
Abstract
Association between long-term exposure to ambient fine particulate matter (PM2.5) and lung cancer incidence is well-documented. However, the role of different PM 2.5 constituents [black carbon (BC), ammonium (NH4+), nitrate (NO3-), organic matter (OM), and inorganic sulfate (SO42- )] remain unclear. The study aimed to specify the associations between PM 2.5 constituents and lung cancer incidence. Based on a prospective cohort of 130,860 participants in Beijing, the present study utilized Cox model to explore the associations between PM 2.5 constituents and lung cancer incidence. We further used mixed exposure models [weighted quantile sum (WQS) and quantile-based g-computation (Qgcomp)] and machine learning model [random forest model with SHapley Additive exPlanations (SHAP)] to specify the importance of each constituent. Results indicated that PM 2.5 mass and its constituents were significantly associated with increased lung cancer incidence. The hazard ratios (HRs) and 95% confidence intervals (CIs) of 1-mu g/m3 increase in the 5-year average concentrations were 1.01 (95% CI: 1.00, 1.02) for PM 2.5 mass, 1.23 (95% CI: 1.06, 1.42) for BC, 1.15 (95% CI: 1.04, 1.27) for NH4+, 1.08 (95% CI: 1.02, 1.16) for NO3-, 1.04 (95% CI: 1.01, 1.06) for OM, and 1.08 (95% CI: 1.03, 1.15) for SO42-.Both the WQS and Qgcomp models assigned the two highest positive weights to BC and SO42-. SHAP analysis identified SO42-and BC as the first and third most important contributors, respectively. Our results indicated that PM 2.5 mass and its constituents were significantly associated with lung cancer incidence, and BC and SO42-were the key constituents in these associations.
MoreTranslated text
Key words
PM 2.5 constituents,Lung cancer incidence,Mixed exposure,Explainable machine learning,Prospective cohort
求助PDF
上传PDF
View via Publisher
AI Read Science
AI Summary
AI Summary is the key point extracted automatically understanding the full text of the paper, including the background, methods, results, conclusions, icons and other key content, so that you can get the outline of the paper at a glance.
Example
Background
Key content
Introduction
Methods
Results
Related work
Fund
Key content
- Pretraining has recently greatly promoted the development of natural language processing (NLP)
- We show that M6 outperforms the baselines in multimodal downstream tasks, and the large M6 with 10 parameters can reach a better performance
- We propose a method called M6 that is able to process information of multiple modalities and perform both single-modal and cross-modal understanding and generation
- The model is scaled to large model with 10 billion parameters with sophisticated deployment, and the 10 -parameter M6-large is the largest pretrained model in Chinese
- Experimental results show that our proposed M6 outperforms the baseline in a number of downstream tasks concerning both single modality and multiple modalities We will continue the pretraining of extremely large models by increasing data to explore the limit of its performance
Upload PDF to Generate Summary
Must-Reading Tree
Example
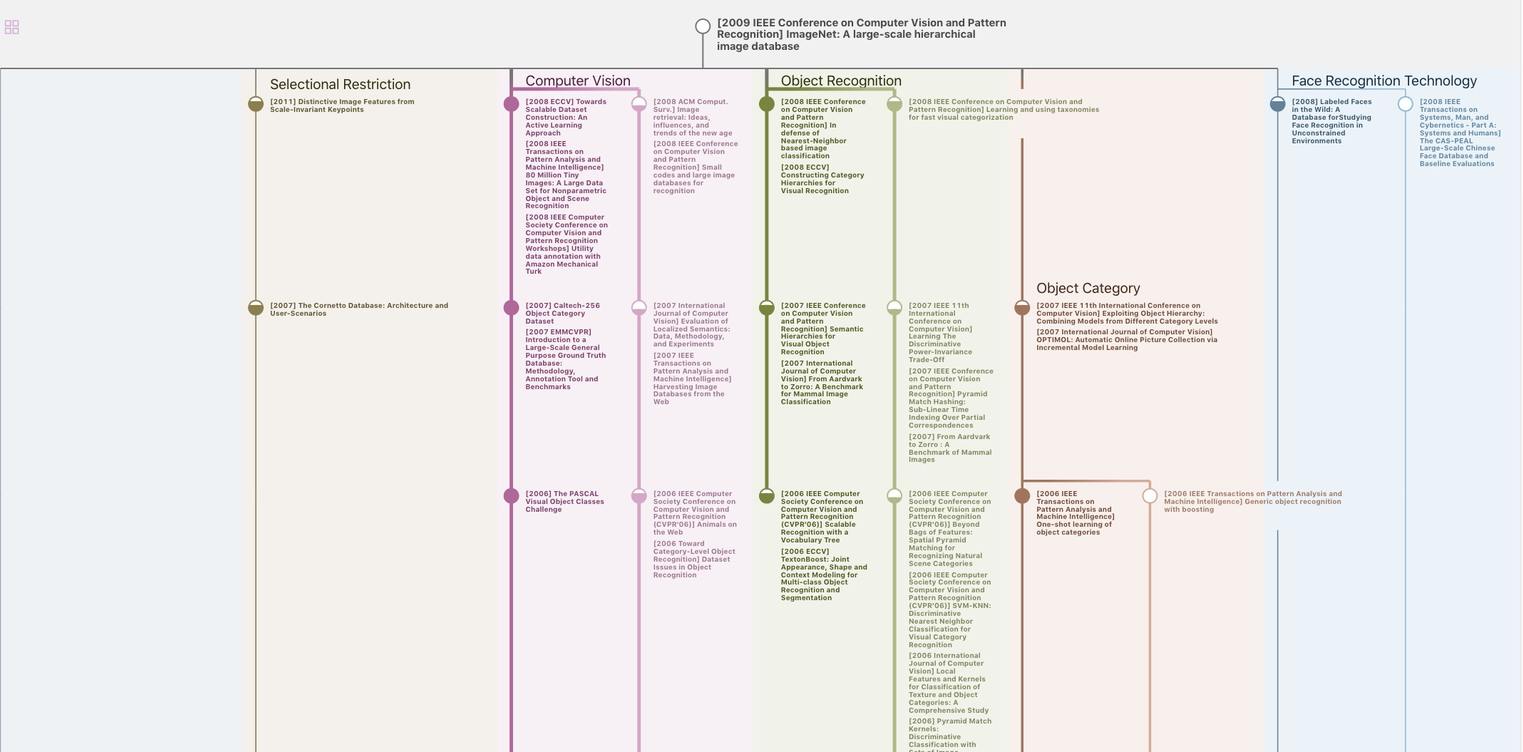
Generate MRT to find the research sequence of this paper
Data Disclaimer
The page data are from open Internet sources, cooperative publishers and automatic analysis results through AI technology. We do not make any commitments and guarantees for the validity, accuracy, correctness, reliability, completeness and timeliness of the page data. If you have any questions, please contact us by email: report@aminer.cn
Chat Paper