From Multifragmentation to Neck Fragmentation: Mass, Isospin, and Velocity Correlations
Physical review. C, Nuclear physics(2012)
Univ Bucharest | Ist Nazl Fis Nucl
Abstract
We present new features of fragmentation dynamics at the transition from semicentral to semiperipheral heavy-ion collisions at Fermi energies, as obtained within a microscopic transport model, the stochastic mean field. We show that, along this transition, specific hierarchy phenomena of some kinematic observables associated with the intermediate mass fragments develop. Their correlations with the dynamics of the isospin degree of freedom, predicted by our calculations, open new possibilities to learn about the density dependence of nuclear symmetry energy below saturation, as well as about the relevant fragmentation mechanisms. Detailed results are presented for mass symmetric Sn + Sn reactions with different isospin content at 50 MeV/nucleon.
MoreTranslated text
PDF
View via Publisher
AI Read Science
AI Summary
AI Summary is the key point extracted automatically understanding the full text of the paper, including the background, methods, results, conclusions, icons and other key content, so that you can get the outline of the paper at a glance.
Example
Background
Key content
Introduction
Methods
Results
Related work
Fund
Key content
- Pretraining has recently greatly promoted the development of natural language processing (NLP)
- We show that M6 outperforms the baselines in multimodal downstream tasks, and the large M6 with 10 parameters can reach a better performance
- We propose a method called M6 that is able to process information of multiple modalities and perform both single-modal and cross-modal understanding and generation
- The model is scaled to large model with 10 billion parameters with sophisticated deployment, and the 10 -parameter M6-large is the largest pretrained model in Chinese
- Experimental results show that our proposed M6 outperforms the baseline in a number of downstream tasks concerning both single modality and multiple modalities We will continue the pretraining of extremely large models by increasing data to explore the limit of its performance
Try using models to generate summary,it takes about 60s
Must-Reading Tree
Example
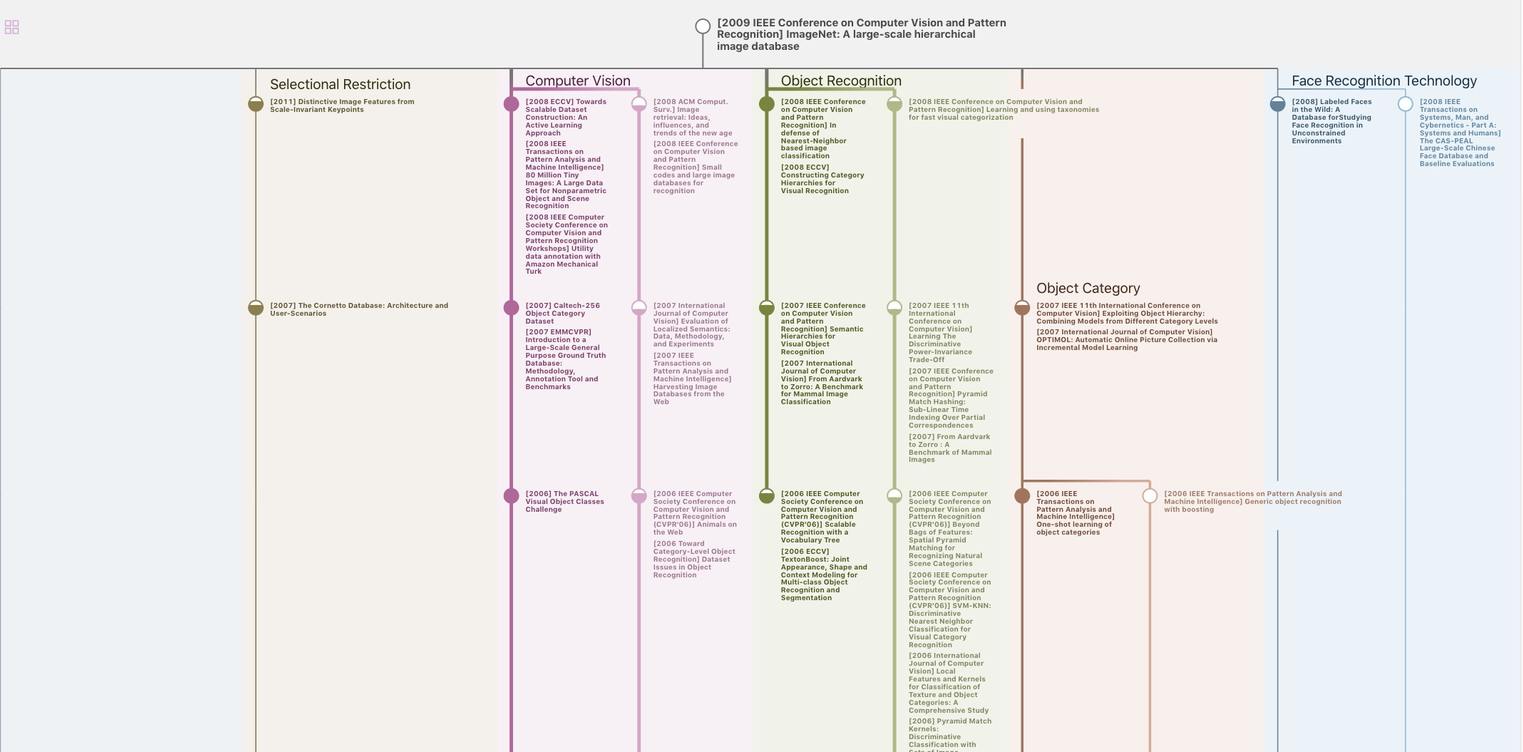
Generate MRT to find the research sequence of this paper
Related Papers
2014
被引用77 | 浏览
2013
被引用0 | 浏览
2014
被引用27 | 浏览
2014
被引用2 | 浏览
2018
被引用6 | 浏览
2019
被引用4 | 浏览
2018
被引用6 | 浏览
2014
被引用68 | 浏览
Data Disclaimer
The page data are from open Internet sources, cooperative publishers and automatic analysis results through AI technology. We do not make any commitments and guarantees for the validity, accuracy, correctness, reliability, completeness and timeliness of the page data. If you have any questions, please contact us by email: report@aminer.cn
Chat Paper
去 AI 文献库 对话