Supplementary Figures from Early Detection of Ovarian Cancer Using Cell-Free DNA Fragmentomes and Protein Biomarkers
crossref(2025)
Abstract
Supplementary Figure S1. Evaluation of screening model DELFI-Pro scores and comorbidities in individuals without cancer. Supplementary Figure S2. DELFI-Pro score evaluation in available clinical characteristics of patients with cancer. Supplementary Figure S3. Stability analysis across fold repeats and collection source. Supplementary Figure S4. Detection of ovarian cancer subtypes using DELFI-Pro screening model. Supplementary Figure S5. ROC analyses of asymptomatic individuals in the using screening or diagnostic models in the Discovery Cohort. Supplementary Figure S6. Performance of ichorCNA and median cfDNA fragment lengths in the Discovery Cohorts. Supplementary Figure S7. Detection of ovarian cancer subtypes using DELFI-Pro at high specificity. Supplementary Figure S8. Genome-wide fragmentation profiles are altered in patients in the Validation Cohort with ovarian cancer. Supplementary Figure S9. Analyses of chromosomal changes in Discovery and Validation cohorts. Supplementary Figure S10. Performance of DELFI-Pro for detection of ovarian cancer in the Validation Cohort. Supplementary Figure S11. Correlation of rank ordered DELFI-Pro scores for the Screening and Diagnostic models. Supplementary Figure S12. Assessment of DELFI-Pro in women with benign lesions. Supplementary Figure S13. Performance of DELFI-Pro for distinguishing ovarian cancer from benign masses. Supplementary Figure S14. Performance of DELFI-Pro for distinguishing ovarian cancer subtypes from benign masses. Supplementary Figure S15. Performance of DELFI-Pro for distinguishing ovarian cancer subtypes from benign masses in Validation Cohort. Supplementary Figure S16. Evaluation of overall tumor burden using the sum of reported lesion diameters. Supplementary Figure S17. Evaluation of CA1-25 and HE4 blood concentrations measured at different centers.
MoreTranslated text
求助PDF
上传PDF
View via Publisher
AI Read Science
AI Summary
AI Summary is the key point extracted automatically understanding the full text of the paper, including the background, methods, results, conclusions, icons and other key content, so that you can get the outline of the paper at a glance.
Example
Background
Key content
Introduction
Methods
Results
Related work
Fund
Key content
- Pretraining has recently greatly promoted the development of natural language processing (NLP)
- We show that M6 outperforms the baselines in multimodal downstream tasks, and the large M6 with 10 parameters can reach a better performance
- We propose a method called M6 that is able to process information of multiple modalities and perform both single-modal and cross-modal understanding and generation
- The model is scaled to large model with 10 billion parameters with sophisticated deployment, and the 10 -parameter M6-large is the largest pretrained model in Chinese
- Experimental results show that our proposed M6 outperforms the baseline in a number of downstream tasks concerning both single modality and multiple modalities We will continue the pretraining of extremely large models by increasing data to explore the limit of its performance
Upload PDF to Generate Summary
Must-Reading Tree
Example
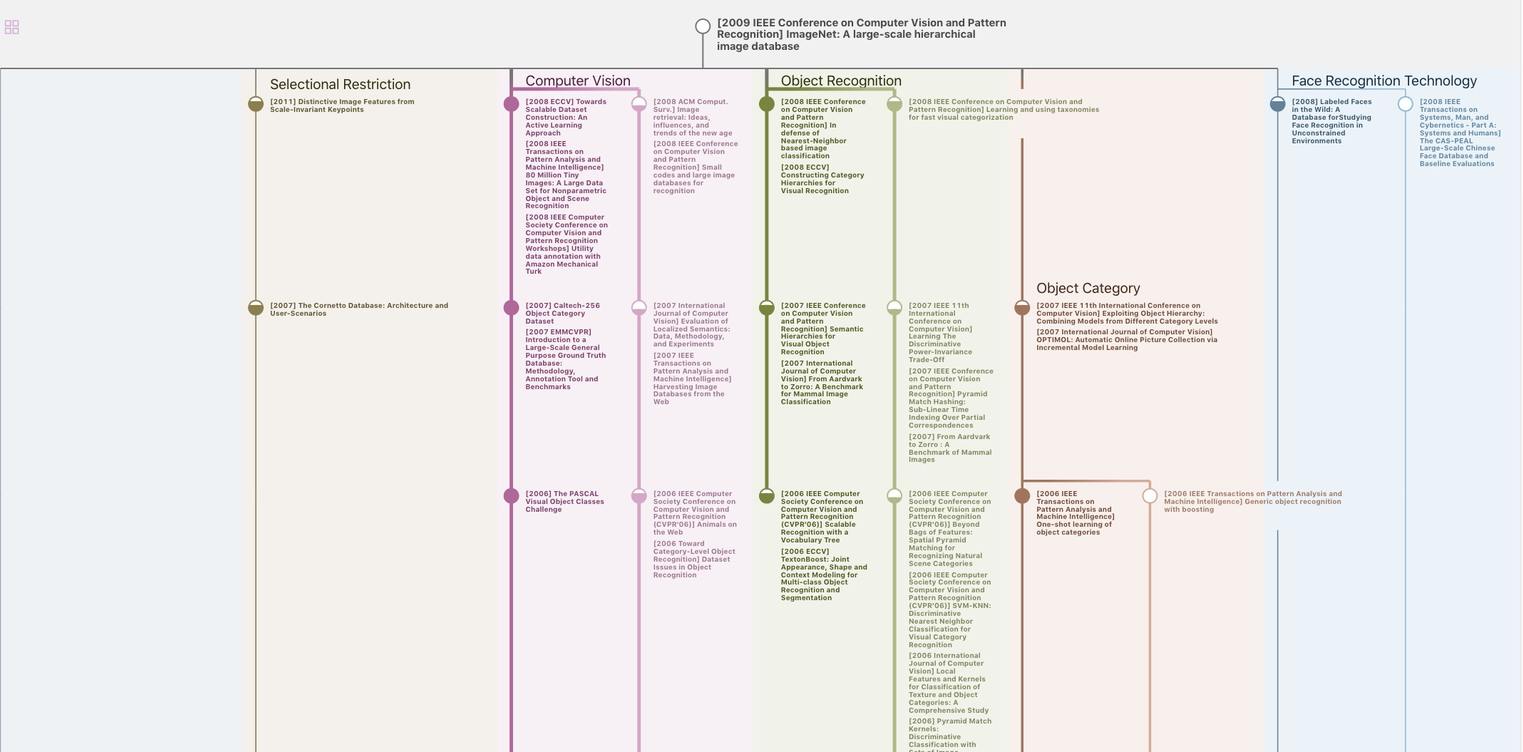
Generate MRT to find the research sequence of this paper
Data Disclaimer
The page data are from open Internet sources, cooperative publishers and automatic analysis results through AI technology. We do not make any commitments and guarantees for the validity, accuracy, correctness, reliability, completeness and timeliness of the page data. If you have any questions, please contact us by email: report@aminer.cn
Chat Paper
GPU is busy, summary generation fails
Rerequest