Evolution of the Sérsic Index Up to Z=2.5 from JWST and HST
arXiv · Astrophysics of Galaxies(2025)
Abstract
The James Webb Space Telescope (JWST) is unveiling the rest-frame near-IR structure of galaxies. We measure the evolution with redshift of the rest-frame optical and near-IR Sérsic index (n), and examine the dependence on stellar mass and star-formation activity across the redshift range 0.5≤ z≤2.5. We infer rest-frame near-IR Sérsic profiles for ≈ 15.000 galaxies in publicly available NIRCam imaging mosaics from the COSMOS-Web and PRIMER surveys. We augment these with rest-frame optical Sérsic indices, previously measured from HST imaging mosaics. The median Sérsic index evolves slowly or not at all with redshift, except for very high-mass galaxies (M_⋆ > 10^11 M_⊙), which show an increase from n≈ 2.5 to n≈ 4 at z<1. High-mass galaxies have higher n than lower-mass galaxies (M_⋆=10^9.5 M_⊙) at all redshifts, with a stronger dependence in the rest-frame near-IR than in the rest-frame optical at z>1. This wavelength dependence is caused by star-forming galaxies that have lower optical than near-IR n at z>1 (but not at z<1). Both at optical and near-IR wavelengths, star-forming galaxies have lower n than quiescent galaxies, fortifying the connection between star-formation activity and radial stellar mass distribution. At z>1 the median near-IR n varies strongly with star formation activity, but not with stellar mass. The scatter in near-IR n is higher in the green valley (0.25 dex) than on the star-forming sequence and among quiescent galaxies (0.18 dex) – this trend is not seen in the optical because dust and young stars contribute to the variety in optical light profiles. Our newly measured rest-frame near-IR radial light profiles motivate future comparisons with radial stellar mass profiles of simulated galaxies as a stringent constraint on processes that govern galaxy formation.
MoreTranslated text
PDF
View via Publisher
AI Read Science
AI Summary
AI Summary is the key point extracted automatically understanding the full text of the paper, including the background, methods, results, conclusions, icons and other key content, so that you can get the outline of the paper at a glance.
Example
Background
Key content
Introduction
Methods
Results
Related work
Fund
Key content
- Pretraining has recently greatly promoted the development of natural language processing (NLP)
- We show that M6 outperforms the baselines in multimodal downstream tasks, and the large M6 with 10 parameters can reach a better performance
- We propose a method called M6 that is able to process information of multiple modalities and perform both single-modal and cross-modal understanding and generation
- The model is scaled to large model with 10 billion parameters with sophisticated deployment, and the 10 -parameter M6-large is the largest pretrained model in Chinese
- Experimental results show that our proposed M6 outperforms the baseline in a number of downstream tasks concerning both single modality and multiple modalities We will continue the pretraining of extremely large models by increasing data to explore the limit of its performance
Try using models to generate summary,it takes about 60s
Must-Reading Tree
Example
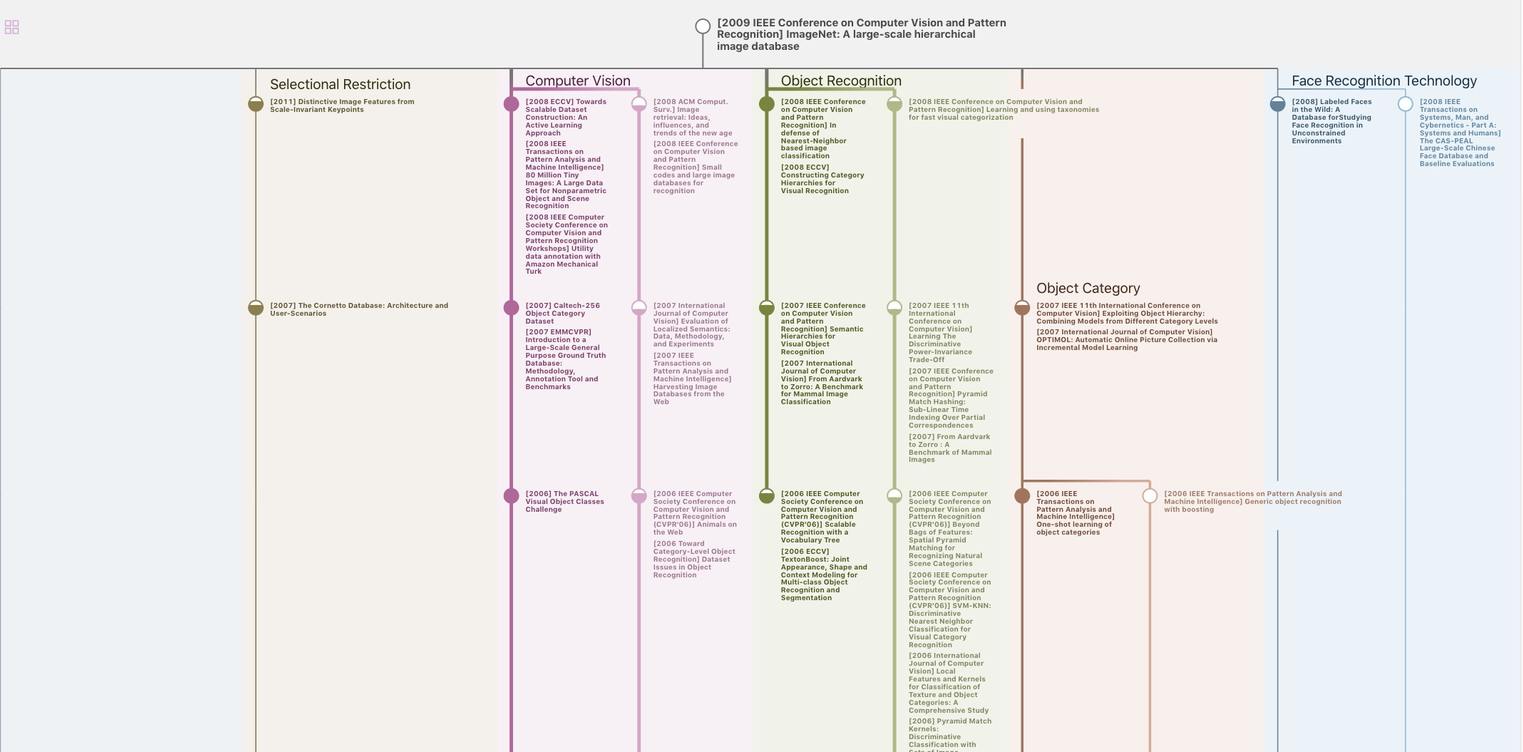
Generate MRT to find the research sequence of this paper
Data Disclaimer
The page data are from open Internet sources, cooperative publishers and automatic analysis results through AI technology. We do not make any commitments and guarantees for the validity, accuracy, correctness, reliability, completeness and timeliness of the page data. If you have any questions, please contact us by email: report@aminer.cn
Chat Paper
去 AI 文献库 对话