Spatial Variability of Hydrochemistry in Coal-Bearing Karst Areas Considering Sulfur Pollution and Underground Engineering Effects
Environmental pollution (Barking, Essex 1987)(2025)
AI 理解论文
溯源树
样例
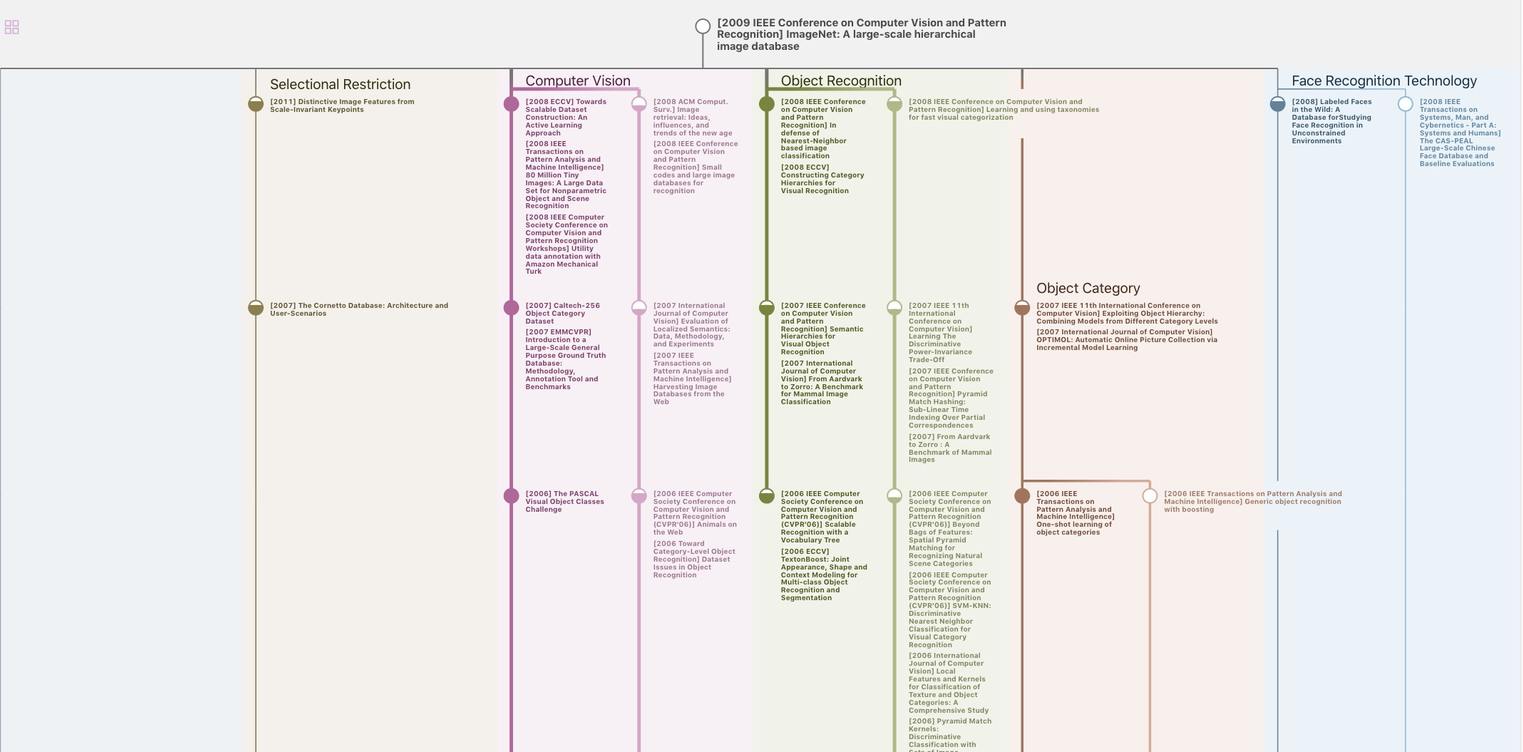
生成溯源树,研究论文发展脉络
Chat Paper
正在生成论文摘要
Environmental pollution (Barking, Essex 1987)(2025)