Use of Mean Apparent Propagator (MAP) MRI in Patients with Acute Ischemic Stroke: A Comparative Study with DTI and NODDI
MAGNETIC RESONANCE IMAGING(2025)
Thomas Jefferson Univ Hosp | Thomas Jefferson Univ
Abstract
Purpose: To evaluate the Mean Apparent Propagator (MAP) MRI for processing multi-shell diffusion imaging in patients with acute ischemic stroke (AIS) and correlate to diffusion tensor imaging (DTI) and neurite orientation and dispersion density imaging (NODDI). Methods: We enrolled patients with AIS from 1/2022 to 4/2024 who underwent multi-shell diffusion imaging on a 3.0-Tesla scanner to generate DTI, NODDI and MAP measures. Mean intensity and standard deviation (SD) were calculated for the infarcted regions-of-interest in b0, fractional anisotropy (FA), mean diffusivity (MD), intra-cellular volume fraction (ICVF), free water fraction (FWF), and orientation dispersion index (ODI), return to the origin probability (RTOP), return to the plane probability (RTPP), return to the axis probability (RTAP), propagator anisotropy (PA), q-space Mean Square Displacement (QMSD), and non-Gaussianity (NG). Results: Twenty-two patients were included with an average age of 69.5 +/- 13.5, mean NIHSS of 12.4 +/- 7.7, and median infarct of 73.3 +/- 10.1 ml. ICVF was correlated with RTPP (rho = 0.82, p < 0.01), RTAP (rho = 0.76, p < 0.01) and RTOP (rho = 0.79, p < 0.01), ODI with PA (rho =-0.83, p < 0.01), FWF with RTOP (rho =-0.73, p < 0.01), RTAP (rho =-0.69, p < 0.01), and RTPP (rho =-0.73, p < 0.01), MD with RTPP (rho =-0.80, p < 0.01), RTOP (rho =-0.79, p < 0.01), and RTAP (rho =-0.77, p < 0.01), FA with RTAP (rho = 0.77, p < 0.01), RTOP (rho = 0.67, p = 0.01), PA (rho = 0.74, p < 0.01), and SD PA (rho = 0.85, p < 0.01). Multivariable linear regression identified the SD QMSD ((3 = 0.406, p = 0.008), thrombectomy ((3 = 0.481, p = 0.002), and infarct volume ((3 = 0.292, p = 0.051) as predictive of stroke severity based on NIHSS. Conclusions: Given its short processing time, MAP MRI is a valuable alternative with potential for clinical use in AIS.
MoreTranslated text
Key words
NODDI,Diffusion MRI,MAP,Stroke
求助PDF
上传PDF
View via Publisher
AI Read Science
AI Summary
AI Summary is the key point extracted automatically understanding the full text of the paper, including the background, methods, results, conclusions, icons and other key content, so that you can get the outline of the paper at a glance.
Example
Background
Key content
Introduction
Methods
Results
Related work
Fund
Key content
- Pretraining has recently greatly promoted the development of natural language processing (NLP)
- We show that M6 outperforms the baselines in multimodal downstream tasks, and the large M6 with 10 parameters can reach a better performance
- We propose a method called M6 that is able to process information of multiple modalities and perform both single-modal and cross-modal understanding and generation
- The model is scaled to large model with 10 billion parameters with sophisticated deployment, and the 10 -parameter M6-large is the largest pretrained model in Chinese
- Experimental results show that our proposed M6 outperforms the baseline in a number of downstream tasks concerning both single modality and multiple modalities We will continue the pretraining of extremely large models by increasing data to explore the limit of its performance
Upload PDF to Generate Summary
Must-Reading Tree
Example
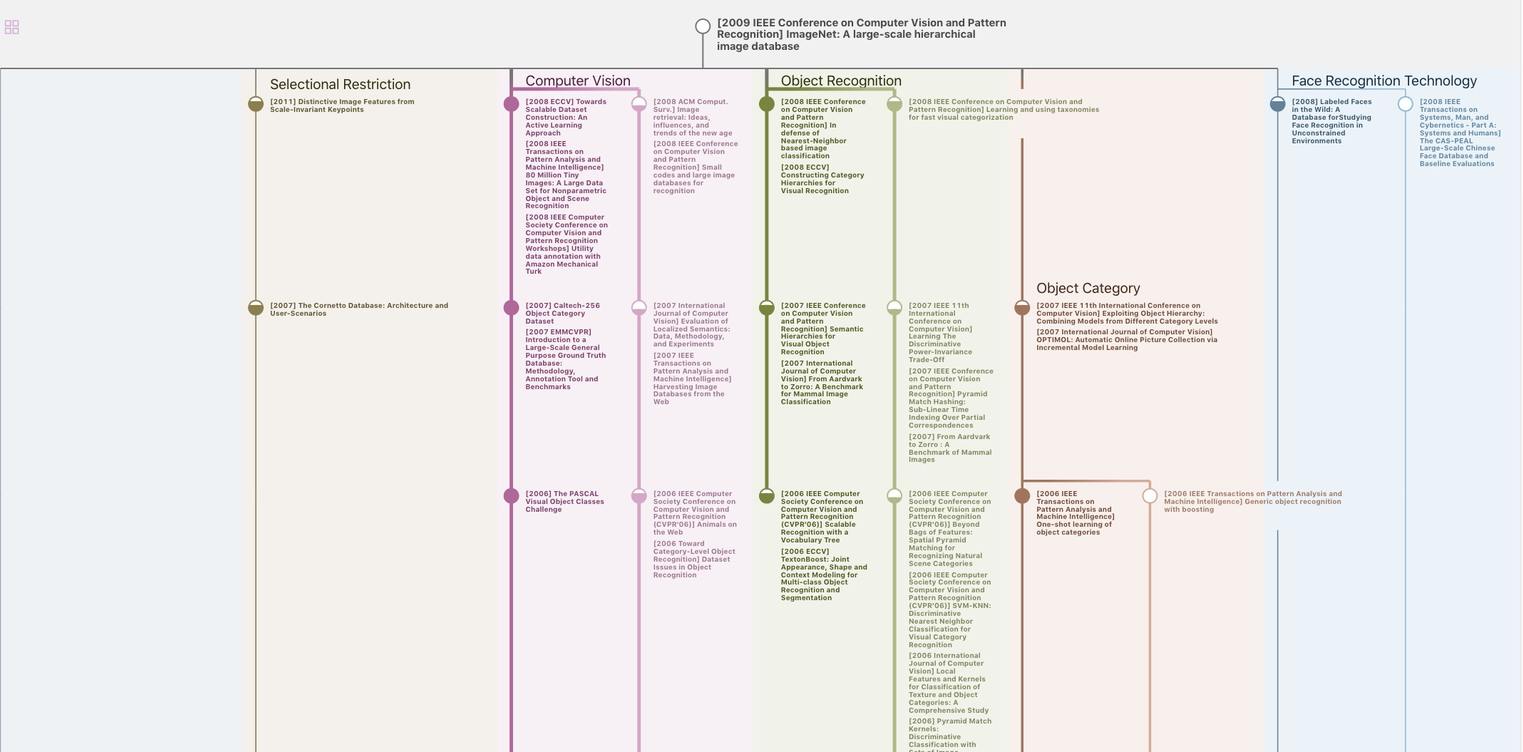
Generate MRT to find the research sequence of this paper
Data Disclaimer
The page data are from open Internet sources, cooperative publishers and automatic analysis results through AI technology. We do not make any commitments and guarantees for the validity, accuracy, correctness, reliability, completeness and timeliness of the page data. If you have any questions, please contact us by email: report@aminer.cn
Chat Paper
GPU is busy, summary generation fails
Rerequest