Metabolic Signature of Insulin Resistance and Risk of Alzheimer's Disease.
JOURNALS OF GERONTOLOGY SERIES A-BIOLOGICAL SCIENCES AND MEDICAL SCIENCES(2025)
Rovira i Virgili Univ URV | Univ Int Catalunya UIC | Pablo Olavide Univ UPO
Abstract
Background Substantial evidence supports the relationship between peripheral insulin resistance (IR) and the development of Alzheimer's disease (AD)-dementia. However, the mechanisms explaining these associations are only partly understood. We aimed to identify a metabolic signature of IR associated with the progression from mild cognitive impairment (MCI) to AD-dementia. Methods This is a case-control study on 400 MCI subjects, free of type 2 diabetes, within the ACE cohort, including individuals ATN + and ATN-. After a median of 2.1 years of follow-up, 142 subjects converted to AD-dementia. IR was assessed using the homeostasis model assessment for insulin resistance (HOMA-IR). A targeted multiplatform approach profiled over 600 plasma metabolites. Elastic net penalized linear regression with 10-fold cross-validation was employed to select those metabolites associated with HOMA-IR. The prediction ability of the signature was assessed using support vector machine and performance metrics. The metabolic signature was associated with AD-dementia risk using a multivariable Cox regression model. Using counterfactual-based mediation analysis, we investigated the mediation role of the metabolic signature between HOMA-IR and AD-dementia. The metabolic pathways in which the metabolites were involved were identified using MetaboAnalyst. Results The metabolic signature comprised 18 metabolites correlated with HOMA-IR. After adjustments by confounders, the signature was associated with increased AD-dementia risk (HR = 1.234; 95% CI = 1.019-1.494; p < .05). The metabolic signature mediated 35% of the total effect of HOMA-IR on AD-dementia risk. Significant metabolic pathways were related to glycerophospholipid and tyrosine metabolism. Conclusions We have identified a blood-based metabolic signature that reflects IR and may enhance our understanding of the biological mechanisms through which IR affects AD-dementia.
MoreTranslated text
Key words
Biomarkers,Blood,Dementia,Metabolomics
求助PDF
上传PDF
View via Publisher
AI Read Science
AI Summary
AI Summary is the key point extracted automatically understanding the full text of the paper, including the background, methods, results, conclusions, icons and other key content, so that you can get the outline of the paper at a glance.
Example
Background
Key content
Introduction
Methods
Results
Related work
Fund
Key content
- Pretraining has recently greatly promoted the development of natural language processing (NLP)
- We show that M6 outperforms the baselines in multimodal downstream tasks, and the large M6 with 10 parameters can reach a better performance
- We propose a method called M6 that is able to process information of multiple modalities and perform both single-modal and cross-modal understanding and generation
- The model is scaled to large model with 10 billion parameters with sophisticated deployment, and the 10 -parameter M6-large is the largest pretrained model in Chinese
- Experimental results show that our proposed M6 outperforms the baseline in a number of downstream tasks concerning both single modality and multiple modalities We will continue the pretraining of extremely large models by increasing data to explore the limit of its performance
Upload PDF to Generate Summary
Must-Reading Tree
Example
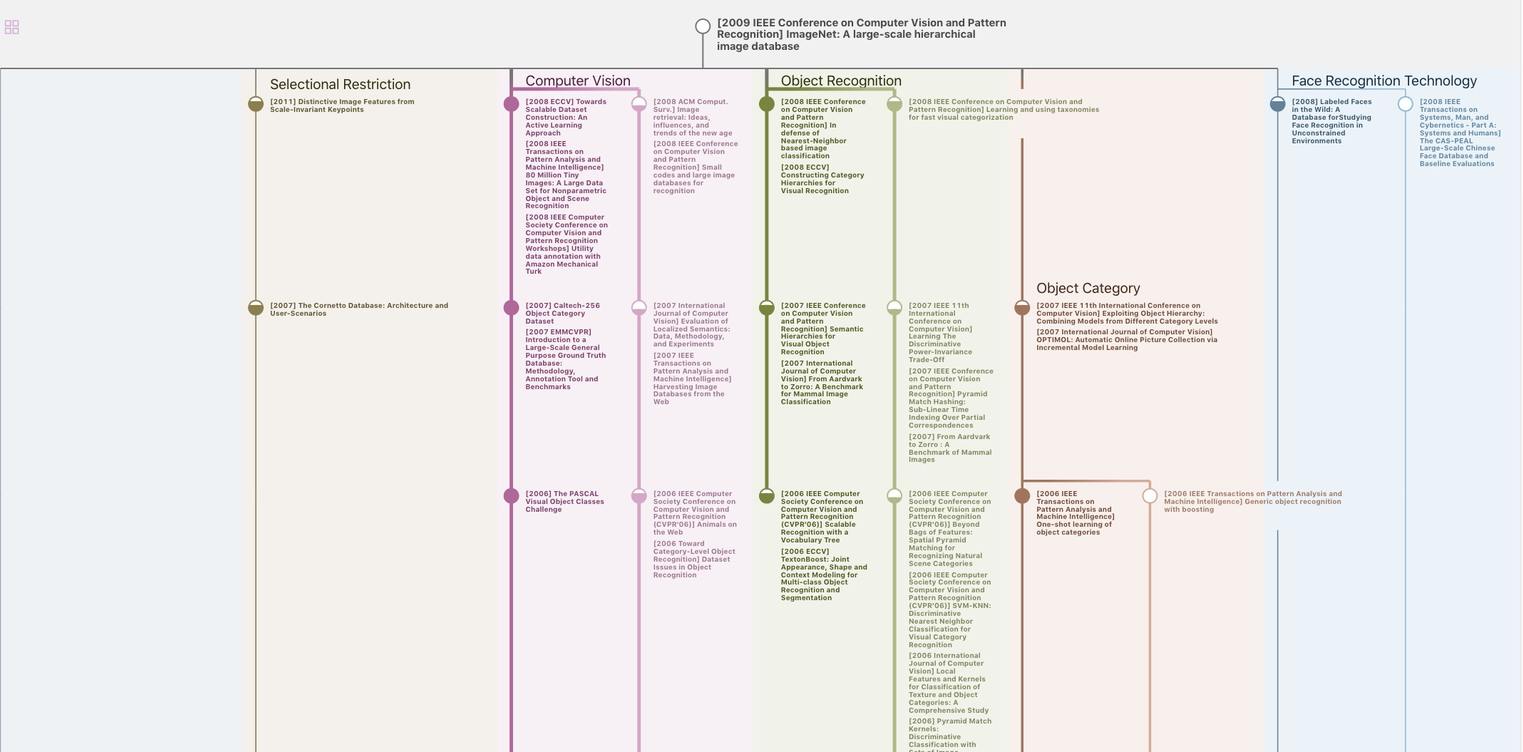
Generate MRT to find the research sequence of this paper
Data Disclaimer
The page data are from open Internet sources, cooperative publishers and automatic analysis results through AI technology. We do not make any commitments and guarantees for the validity, accuracy, correctness, reliability, completeness and timeliness of the page data. If you have any questions, please contact us by email: report@aminer.cn
Chat Paper