Silver Nanostructures for Determination of FKBP12 Protein
Engineering Proceedings(2024)
Abstract
FKBP12 is a peptidyl––prolyl cis–trans isomerase that was recently proposed as a candidate biomarker for cancer, for neurodegenerations and for anti–rejection therapy after organ transplant. We designed and fabricated a nanosensor platform for the rapid and efficient determination of FKBP12 concentration in biological fluids exploiting anisotropic silver nanoparticles (AgNps) to enhance the capabilities of Quartz Crystal Microbalance (QCM) detection. To this end, the QCM sensor was coated with a compact array of AgNPs that were further functionalized with a Self–Assembled–Monolayer containing a synthetic receptor, GPS–SH1, designed and synthesized specifically to selectively bind FKBP12. Silver nanoflowers, AgNFs, and silver dendrites, AgNDs, were prepared by electrodeposition and characterized by means of UV–Vis spectroscopy, Scanning Electron Microscopy (SEM), QCM and water contact angle (CA). The AgNPs@Au/GPS–SH1–functionalized QCM sensors were used to detect increasing concentrations of FKBP12 in solution; the results showed that the use of AgNDs significantly enhanced the sensitivity of the sensor with respect to flat Ag sensor chips, allowing the detection of FKBP12 at sub–picomolar concentrations.
MoreTranslated text
Key words
FKBP12,nanosensors,silver nanostructures,neurodegenerative diseases,cancer,transplant rejection
求助PDF
上传PDF
View via Publisher
AI Read Science
AI Summary
AI Summary is the key point extracted automatically understanding the full text of the paper, including the background, methods, results, conclusions, icons and other key content, so that you can get the outline of the paper at a glance.
Example
Background
Key content
Introduction
Methods
Results
Related work
Fund
Key content
- Pretraining has recently greatly promoted the development of natural language processing (NLP)
- We show that M6 outperforms the baselines in multimodal downstream tasks, and the large M6 with 10 parameters can reach a better performance
- We propose a method called M6 that is able to process information of multiple modalities and perform both single-modal and cross-modal understanding and generation
- The model is scaled to large model with 10 billion parameters with sophisticated deployment, and the 10 -parameter M6-large is the largest pretrained model in Chinese
- Experimental results show that our proposed M6 outperforms the baseline in a number of downstream tasks concerning both single modality and multiple modalities We will continue the pretraining of extremely large models by increasing data to explore the limit of its performance
Upload PDF to Generate Summary
Must-Reading Tree
Example
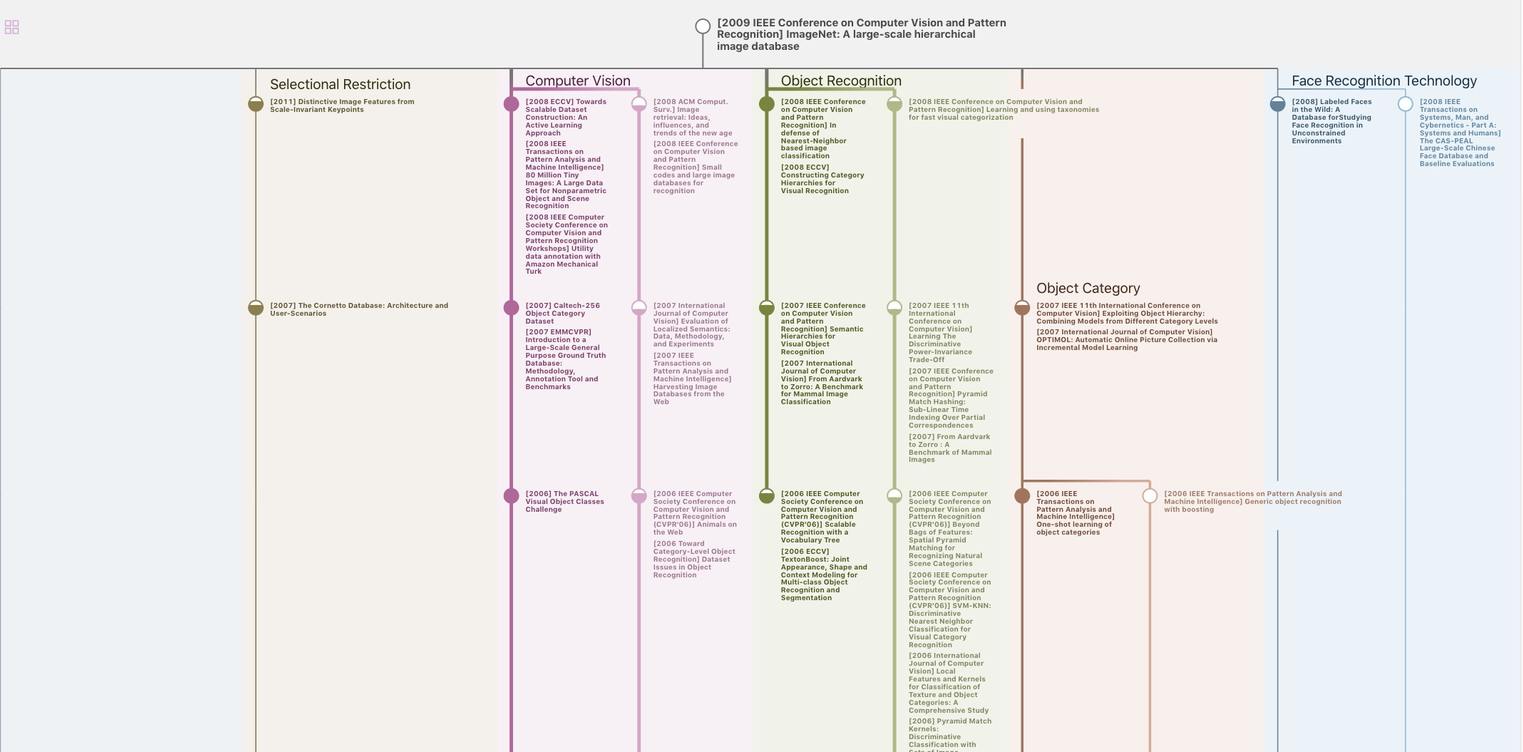
Generate MRT to find the research sequence of this paper
Data Disclaimer
The page data are from open Internet sources, cooperative publishers and automatic analysis results through AI technology. We do not make any commitments and guarantees for the validity, accuracy, correctness, reliability, completeness and timeliness of the page data. If you have any questions, please contact us by email: report@aminer.cn
Chat Paper