Artificial Intelligence-assisted Raman Spectroscopy for Liver Cancer Diagnosis
EPJ Web of Conferences(2024)
Optoelectronic Division-Engineering Department | Centro Regionale Information Communication Technology (CeRICT Scrl)
Abstract
Hepatocellular carcinoma (HCC), the most common form of primary liver cancer, represents a global health challenge due to its complexity and the limitations of current diagnostic techniques. By combining Raman spectroscopy and Artificial Intelligence (AI), we have succeeded in classifying tumor cells. In fact, we have performed a first Raman spectral analysis based on the characterization and differentiation between uncultured primary human liver cells derived from resected HCC tumor tissue and the adjacent non-tumor counterpart. Biochemical analysis of the collected Raman spectra revealed that there is more DNA in the nuclei of the tumor cells than in non-tumor cells. We then develop three machine learning approaches, including multivariate models and neural networks, to rapidly automate the recognition and classification of the Raman spectra of both cells. To evaluate the performance of the developed AI models, we prepared and analyzed two additional cell samples with a ratio of 4:1 and 3:1 between tumor and non-tumor cells and compared the obtained results with the nominal percentages (accuracy of 80 and 60%, respectively). These results confirm that the models are able to make classifications at the level of a single spectrum, indicating the possibility of rapidly analysing and classifying a primary HCC cell.
MoreTranslated text
求助PDF
上传PDF
View via Publisher
AI Read Science
AI Summary
AI Summary is the key point extracted automatically understanding the full text of the paper, including the background, methods, results, conclusions, icons and other key content, so that you can get the outline of the paper at a glance.
Example
Background
Key content
Introduction
Methods
Results
Related work
Fund
Key content
- Pretraining has recently greatly promoted the development of natural language processing (NLP)
- We show that M6 outperforms the baselines in multimodal downstream tasks, and the large M6 with 10 parameters can reach a better performance
- We propose a method called M6 that is able to process information of multiple modalities and perform both single-modal and cross-modal understanding and generation
- The model is scaled to large model with 10 billion parameters with sophisticated deployment, and the 10 -parameter M6-large is the largest pretrained model in Chinese
- Experimental results show that our proposed M6 outperforms the baseline in a number of downstream tasks concerning both single modality and multiple modalities We will continue the pretraining of extremely large models by increasing data to explore the limit of its performance
Upload PDF to Generate Summary
Must-Reading Tree
Example
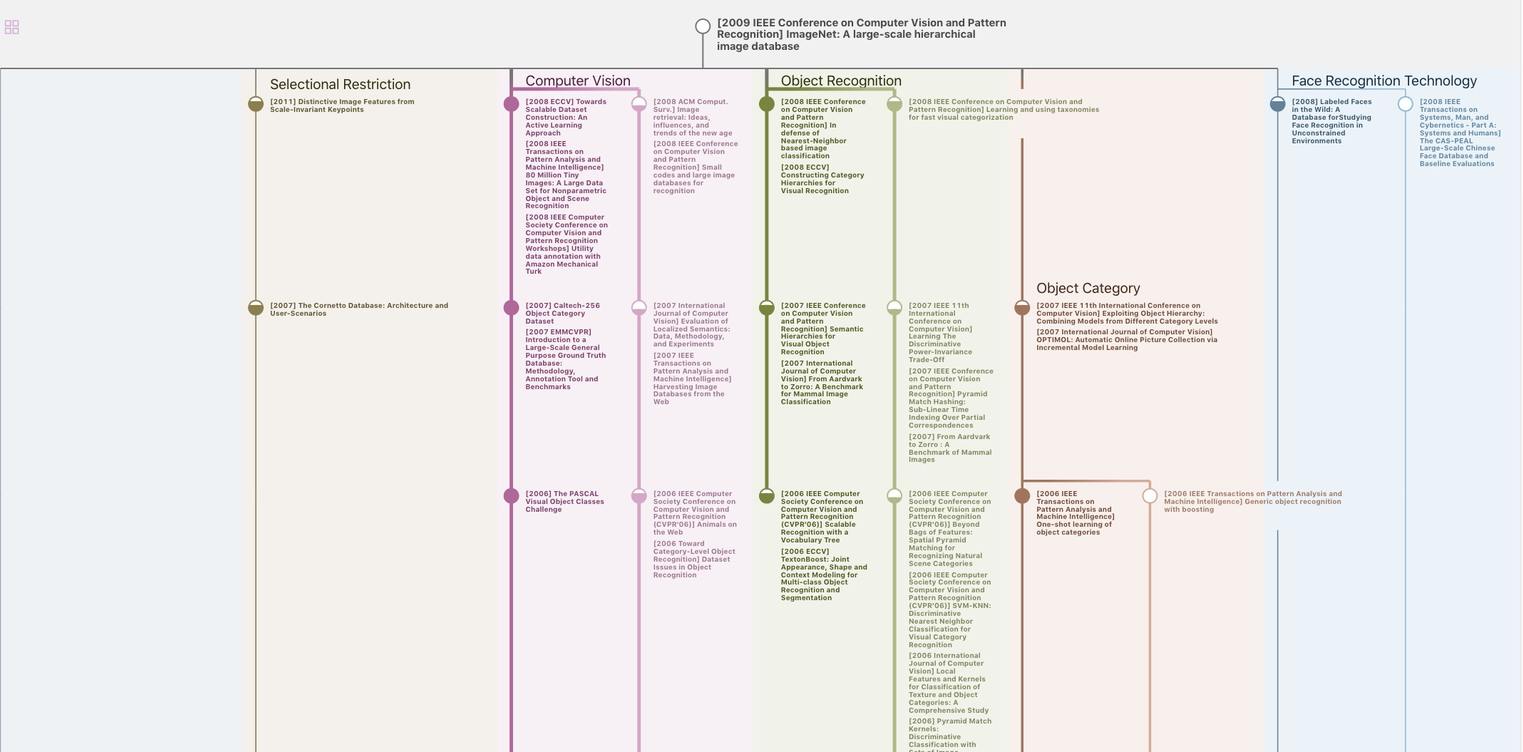
Generate MRT to find the research sequence of this paper
Data Disclaimer
The page data are from open Internet sources, cooperative publishers and automatic analysis results through AI technology. We do not make any commitments and guarantees for the validity, accuracy, correctness, reliability, completeness and timeliness of the page data. If you have any questions, please contact us by email: report@aminer.cn
Chat Paper