ManufVisSGG: A Vision-Language-Model Approach for Cognitive Scene Graph Generation in Manufacturing Systems
IEEE International Conference on Automation Science and Engineering (CASE) : [proceedings](2024)
School of Mechanical Engineering and Automation | Department of Industrial and Systems Engineering
Abstract
To establish a cognitive manufacturing system, scene graph generation (SGG) that lets machines/robots understand objects and their relations under varied scenarios is an essential task. Existing research on SGG primarily focuses on detection and panoptic segmentation approaches, where objects are identified through bounding boxes or panoptic segmentation, followed by the prediction of their pairwise relationships. This process means that the quality of the final scene graph predictions is heavily influenced by the quality of costly annotations. To tackle this issue, we propose the Manufacturing Visual Scene Graph Generation (ManufVisSGG) method, a simple yet powerful approach that leverages the capabilities of Vision-Language Models (VLMs) to generate scene graphs quickly and accurately without any additional object annotations. Furthermore, leveraging the ManufVisSGG method, we have implemented a meticulous annotation procedure to compile a high-quality manufacturing scene graph generation (MSG) dataset, comprising 10,000 images of manufacturing and other industrial scenes. Through comparisons with various scene graph generation methods and benchmarks across two other datasets, we have showcased the superiority of the ManufVisSGG method and underscored the benefits of the MSG dataset over existing datasets.
MoreTranslated text
Key words
Manufacturing Systems,Scene Graph,Powerful Approach,Bounding Box,Pairwise Relationships,Annotation Quality,Additional Annotations,Object Annotations,Human Activities,Visual Information,Cognitive Tasks,Object Detection,Image Object,Language Model,Fundamental Task,Scene Understanding
求助PDF
上传PDF
View via Publisher
AI Read Science
AI Summary
AI Summary is the key point extracted automatically understanding the full text of the paper, including the background, methods, results, conclusions, icons and other key content, so that you can get the outline of the paper at a glance.
Example
Background
Key content
Introduction
Methods
Results
Related work
Fund
Key content
- Pretraining has recently greatly promoted the development of natural language processing (NLP)
- We show that M6 outperforms the baselines in multimodal downstream tasks, and the large M6 with 10 parameters can reach a better performance
- We propose a method called M6 that is able to process information of multiple modalities and perform both single-modal and cross-modal understanding and generation
- The model is scaled to large model with 10 billion parameters with sophisticated deployment, and the 10 -parameter M6-large is the largest pretrained model in Chinese
- Experimental results show that our proposed M6 outperforms the baseline in a number of downstream tasks concerning both single modality and multiple modalities We will continue the pretraining of extremely large models by increasing data to explore the limit of its performance
Upload PDF to Generate Summary
Must-Reading Tree
Example
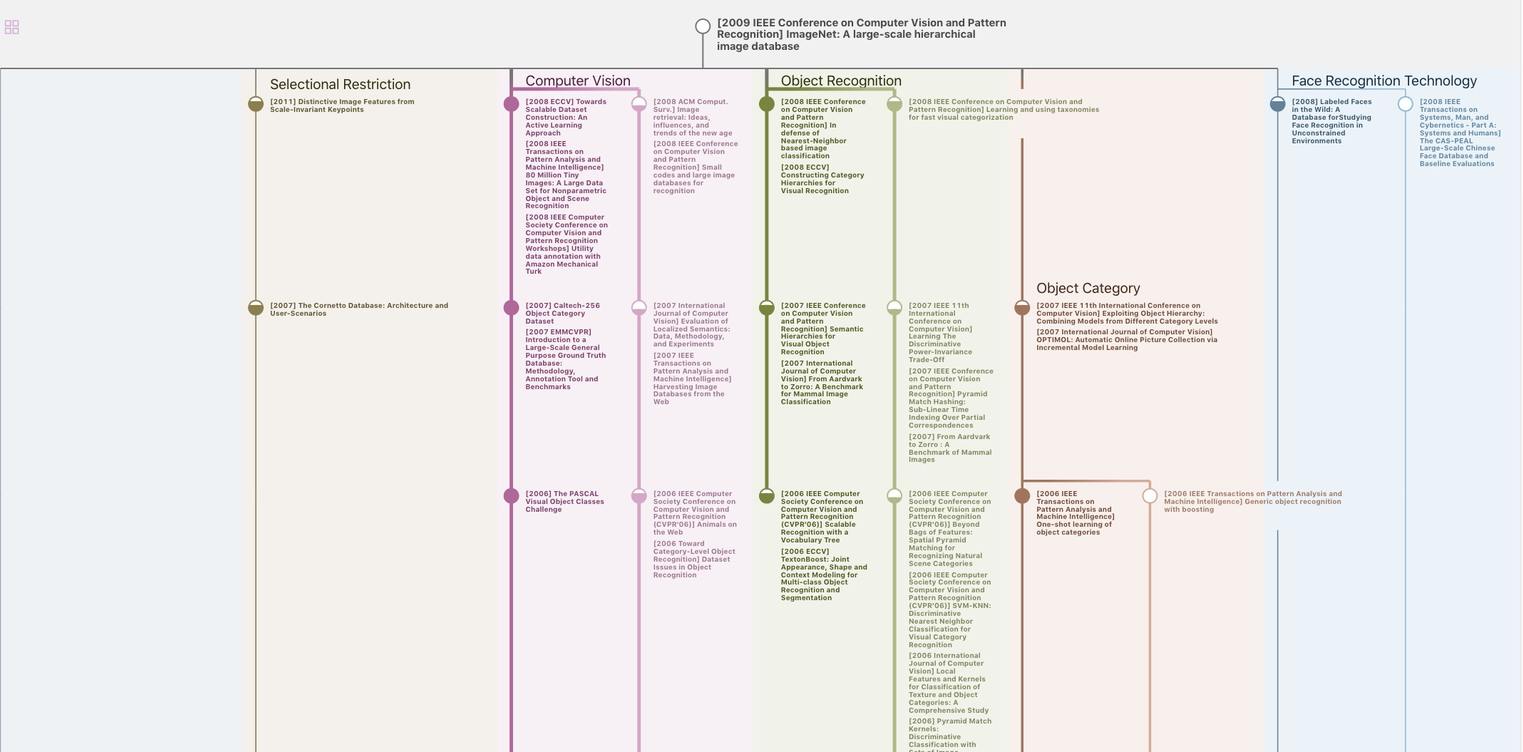
Generate MRT to find the research sequence of this paper
Data Disclaimer
The page data are from open Internet sources, cooperative publishers and automatic analysis results through AI technology. We do not make any commitments and guarantees for the validity, accuracy, correctness, reliability, completeness and timeliness of the page data. If you have any questions, please contact us by email: report@aminer.cn
Chat Paper