Learning the Dynamics in Sequential Recommendation by Exploiting Real-time Information
CIKM '24 Proceedings of the 33rd ACM International Conference on Information and Knowledge Management(2024)
摘要
Sequential recommender systems offer personalized suggestions by modeling users' interactions chronologically to capture dynamic user interest. Existing approaches typically fail to adequately describe the dynamics of the entire recommender system, including shifts in both user interest and item availability. To address this, we propose a simple yet effective framework with three key perspectives, tailored to the dynamics of recommender system by fully exploiting the time information. Firstly, we propose a dynamic candidate set construction approach to prevent the model from learning future interactions. Secondly, assuming that user behaviors remain consistent over short terms but may evolve over long terms, we employ a interval-weighted optimization target to model the correlation of users' historical interactions. Finally, we introduce a specialized time-aware attention module to enhance recommendations within specific temporal contexts. Extensive experiments demonstrate the effectiveness and generalizability of our framework. We make our codes publicly available.
更多查看译文
AI 理解论文
溯源树
样例
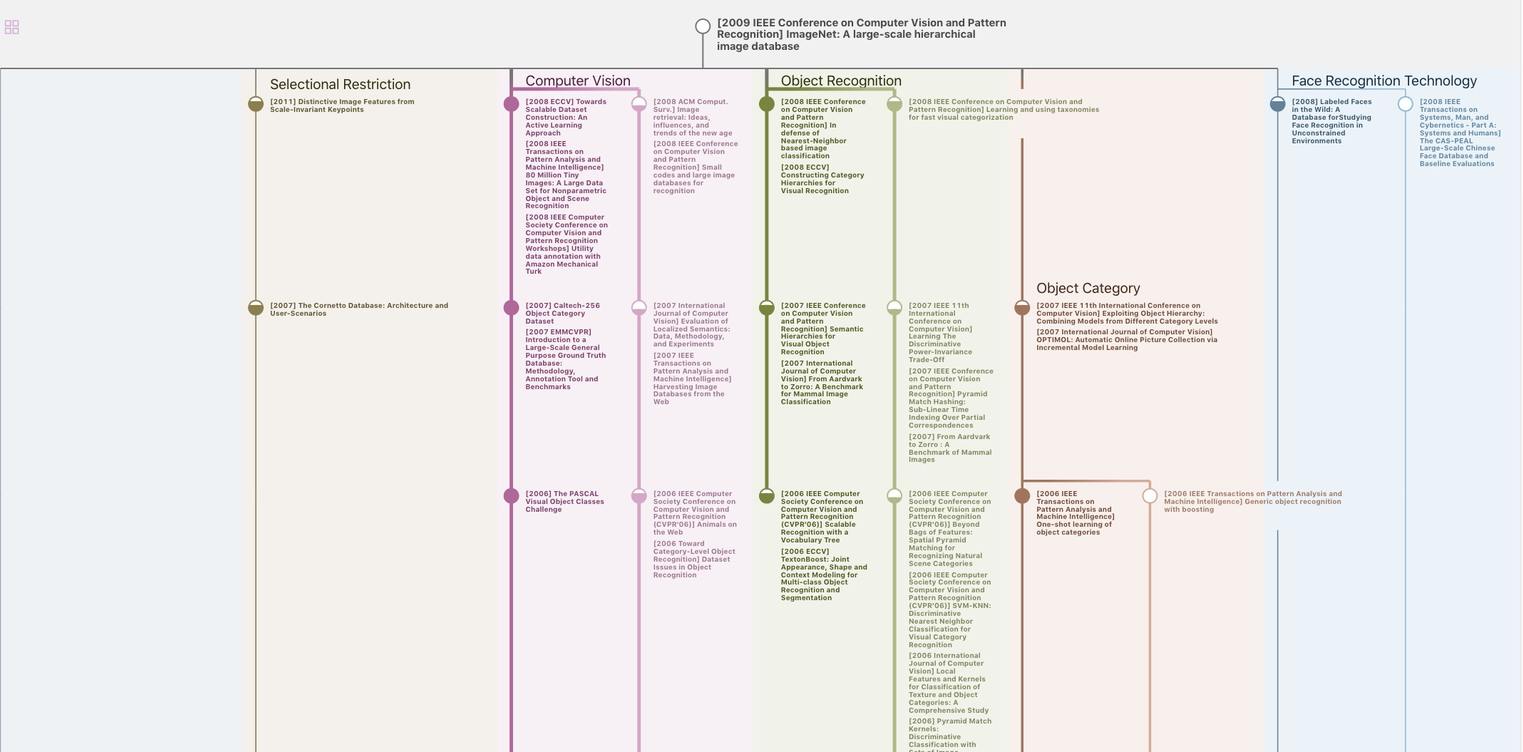
生成溯源树,研究论文发展脉络
Chat Paper
正在生成论文摘要