F85. GENETIC OVERLAP OF BRAIN STRUCTURE AND CONNECTIVITY WITH SCHIZOPHRENIA: DIFFERENT STATISTICAL METHODS PROVIDE COMPLEMENTARY INSIGHTS
Schizophrenia Research(2024)SCI 2区
Abstract
Background Schizophrenia is a highly heritable brain disorder with a complex genetic architecture and shows significant differences in brain morphology, white matter integrity, and functional connectivity. Many brain structural and functional phenotypes exhibit high heritability and polygenicity and are associated with a higher polygenic risk score of schizophrenia, suggesting that the genetic risk of schizophrenia may be linked to both brain structure and function. This study aims to deepen our understanding of the shared genetic architecture between schizophrenia and the brain using complementary statistical methods. Methods We utilized genome-wide association study data (GWAS) for schizophrenia from the Psychiatric Genomic Consortium wave 3 and brain imaging-derived phenotypes from the UK Biobank. Our study included 37 representative brain measures from T1 (total surface area, mean cortical thickness, intracranial volume, 8 subcortical volumes), diffusion MRI (mean FA of 15 white matter tracts), and resting-state fMRI (11 functional network nodes). We integrated these data with a clinical cohort with genotype and phenotype data (1065 cases, 1037 controls) to calculate brain polygenic scores (PGS) for each participant. For each schizophrenia-brain pair, linkage disequilibrium score regression (LDSC) was used to calculate the genetic correlation, and bivariate MiXeR analysis estimated the number of shared and trait-specific causal variants, as well as the fraction of shared variants with concordant effect directions. The regression coefficient between brain PGS and schizophrenia diagnosis assessed the relationship between schizophrenia and brain phenotypes. The conjunctional false discovery rate (conjFDR) analysis was employed to detect specific shared loci, followed by enrichment analysis of unique genes mapped from all identified loci using Gene Ontology (GO) and Kyoto Encyclopedia of Genes and Genomes (KEEG) pathways. Results The LDSC analysis revealed weak and insignificant genetic correlations between schizophrenia and all brain phenotypes, except for one functional node (left lateral network, r = 0.146, pFDR = 0.019). No PGSs for all brain measures were significantly associated with schizophrenia. However, bivariate MiXeR analysis showed that all the brain measures shared substantial variants with schizophrenia (11.41 % ∼ 95.47%), with a varied proportion of shared variants with concordant effect directions (0.144 ∼ 0.814). A significant positive correlation was observed between the genetic correlation and the proportion of shared variants with concordance effect direction (r = 0.780, pFDR = 0.020). The conjFDR identified 218, 139, and 24 shared loci between schizophrenia and T1, DTI, and fMRI brain measures, respectively. Enrichment analysis of 217 unique genes mapped from these loci indicated significant enrichment in four GO terms: molecular function, protein binding, cell adhesion molecule binding, and postsynaptic density. Discussion We provide evidence of extensive genetic overlap with mixed effect directions between schizophrenia and the brain phenotypes suggesting independent genetic architectures. The shared genetic component between schizophrenia and the brain may play roles in cell adhesion molecules binding and postsynaptic density. Diverse methodologies allow us to dissect the complex shared genetic architecture between schizophrenia and brain imaging phenotypes from different perspectives.
MoreTranslated text
求助PDF
上传PDF
View via Publisher
AI Read Science
AI Summary
AI Summary is the key point extracted automatically understanding the full text of the paper, including the background, methods, results, conclusions, icons and other key content, so that you can get the outline of the paper at a glance.
Example
Background
Key content
Introduction
Methods
Results
Related work
Fund
Key content
- Pretraining has recently greatly promoted the development of natural language processing (NLP)
- We show that M6 outperforms the baselines in multimodal downstream tasks, and the large M6 with 10 parameters can reach a better performance
- We propose a method called M6 that is able to process information of multiple modalities and perform both single-modal and cross-modal understanding and generation
- The model is scaled to large model with 10 billion parameters with sophisticated deployment, and the 10 -parameter M6-large is the largest pretrained model in Chinese
- Experimental results show that our proposed M6 outperforms the baseline in a number of downstream tasks concerning both single modality and multiple modalities We will continue the pretraining of extremely large models by increasing data to explore the limit of its performance
Upload PDF to Generate Summary
Must-Reading Tree
Example
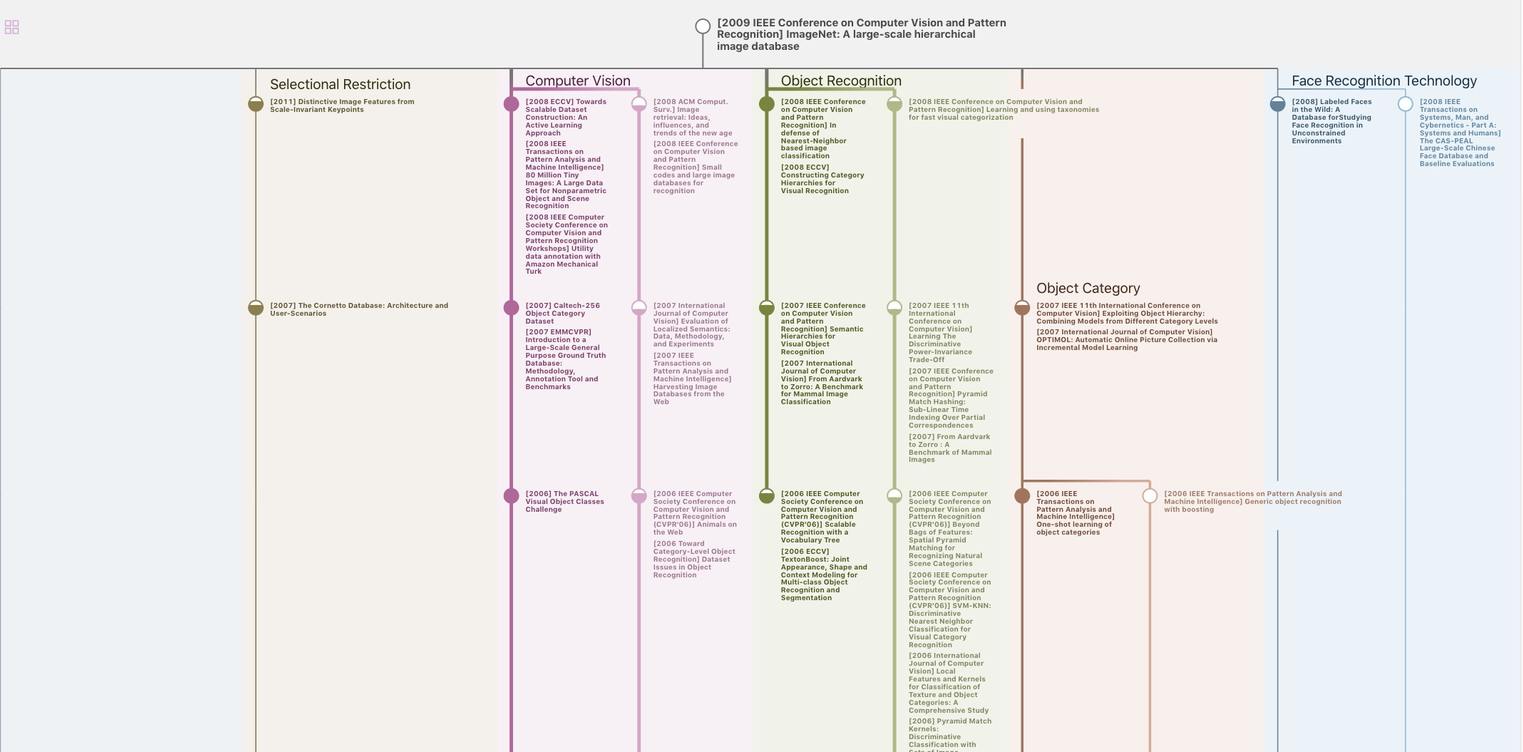
Generate MRT to find the research sequence of this paper
Data Disclaimer
The page data are from open Internet sources, cooperative publishers and automatic analysis results through AI technology. We do not make any commitments and guarantees for the validity, accuracy, correctness, reliability, completeness and timeliness of the page data. If you have any questions, please contact us by email: report@aminer.cn
Chat Paper
GPU is busy, summary generation fails
Rerequest