Recurrence and Resistance Risk Factors in Low-Risk Gestational Trophoblastic Neoplasia
International journal of gynecological cancer official journal of the International Gynecological Cancer Society(2025)
Abstract
Gestational trophoblastic neoplasia (GTN) is a group of rare but highly curable pregnancy-related tumors, especially in low-risk cases. However, around 25% of patients with GTN develop either resistant or recurrent disease after initial chemotherapy. To enhance the understanding of the mechanisms driving treatment failures and to develop more personalized and effective therapeutic strategies, this review explored diverse factors influencing low-risk GTN prognosis. These factors include FIGO (International Federation of Gynecology and Obstetrics) risk score, histology, patient age, pregnancy type, human chorionic gonadotropin (hCG) levels, disease duration, tumor characteristics, metastasis, Doppler ultrasonography, and consolidation chemotherapy. Additionally, the review examined independent risk determinants for disease recurrence and resistance to single-agent chemotherapy in patients with low-risk GTN. In most previous studies on the risk factors related to low-risk GTN, resistance and recurrence have typically been examined independently, despite their overlapping and interrelated nature. Furthermore, they often involve small sample sizes, suffer from methodological shortcomings, and exhibit limited statistical power.Studies utilizing multivariate analysis have shown that independent risk determinants for resistance to first-line treatment include FIGO score, metastatic disease, pre-treatment hCG level, interval between antecedent pregnancy and GTN diagnosis, tumor size, uterine artery pulsatility index (UAPI), choriocarcinoma, lung metastases, lung nodule size, and clearance hCG quartile. The independent predictive factors associated with recurrence include lung metastases, lung nodule size, interval between antecedent pregnancy and chemotherapy, interval from first chemotherapy to hCG normalization, post-delivery low-risk GTN, number of chemotherapy courses to achieve hCG normalization, and number of consolidation chemotherapy cycles. However, while these identified predictive factors offer valuable guidance, the variability in definitions and populations across studies may have implications for the generalizability of their findings. A comprehensive approach using clear definitions and taking into account multiple predictive factors may be necessary for accurately assessing the risk of resistance and recurrence in patients with low-risk GTN.
MoreTranslated text
求助PDF
上传PDF
View via Publisher
AI Read Science
AI Summary
AI Summary is the key point extracted automatically understanding the full text of the paper, including the background, methods, results, conclusions, icons and other key content, so that you can get the outline of the paper at a glance.
Example
Background
Key content
Introduction
Methods
Results
Related work
Fund
Key content
- Pretraining has recently greatly promoted the development of natural language processing (NLP)
- We show that M6 outperforms the baselines in multimodal downstream tasks, and the large M6 with 10 parameters can reach a better performance
- We propose a method called M6 that is able to process information of multiple modalities and perform both single-modal and cross-modal understanding and generation
- The model is scaled to large model with 10 billion parameters with sophisticated deployment, and the 10 -parameter M6-large is the largest pretrained model in Chinese
- Experimental results show that our proposed M6 outperforms the baseline in a number of downstream tasks concerning both single modality and multiple modalities We will continue the pretraining of extremely large models by increasing data to explore the limit of its performance
Upload PDF to Generate Summary
Must-Reading Tree
Example
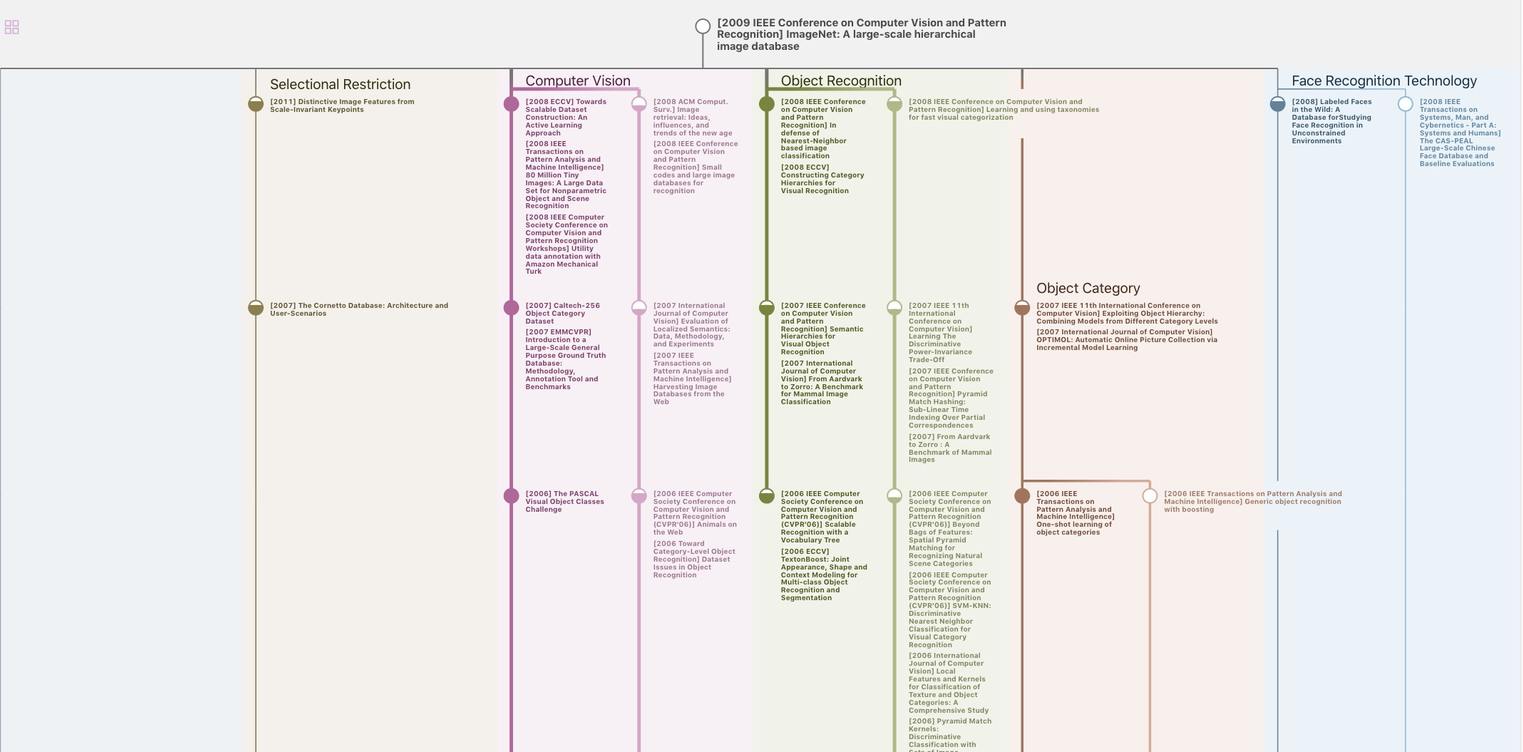
Generate MRT to find the research sequence of this paper
Data Disclaimer
The page data are from open Internet sources, cooperative publishers and automatic analysis results through AI technology. We do not make any commitments and guarantees for the validity, accuracy, correctness, reliability, completeness and timeliness of the page data. If you have any questions, please contact us by email: report@aminer.cn
Chat Paper