Forest Height Estimation with TanDEM-X SAR and InSAR Features Using Deep Learning
IEEE Geoscience and Remote Sensing Letters(2024)CCF CSCI 2区SCI 3区
German Aerosp Ctr DLR
Abstract
Accurate forest height estimates lead to improved accuracy of biomass estimation and are crucial for monitoring and conservation efforts. Interferometric synthetic aperture radar (InSAR) techniques use two synthetic aperture radar (SAR) images to measure the interferometric coherence that includes the volumetric decorrelation which is known to be related to forest canopy height. Several approximations and assumptions are made in different steps to compute volumetric decorrelation and to invert it to forest canopy height using physical models. Data-driven approaches overcome the potential bias introduced by these assumptions by directly estimating forest canopy height. However, the question of optimal representation and level of processing of the input data is often neglected. We address this gap comparing different SAR and InSAR input features such as single-look-complex (SLC) images, backscatter, coherence, and volumetric decorrelation. The resulting best model has a root-mean-squared error (RMSE) of 6.12 m with volumetric decorrelation as primary input feature. It is followed using coherence as primary input with an RMSE of 6.30 m.
MoreTranslated text
Key words
Forestry,Decorrelation,Estimation,Coherence,Laser radar,Biological system modeling,Synthetic aperture radar,Backscatter,Accuracy,Optical sensors,Deep learning (DL),forest canopy height,synthetic aperture radar (SAR),TanDEM-X
求助PDF
上传PDF
View via Publisher
AI Read Science
AI Summary
AI Summary is the key point extracted automatically understanding the full text of the paper, including the background, methods, results, conclusions, icons and other key content, so that you can get the outline of the paper at a glance.
Example
Background
Key content
Introduction
Methods
Results
Related work
Fund
Key content
- Pretraining has recently greatly promoted the development of natural language processing (NLP)
- We show that M6 outperforms the baselines in multimodal downstream tasks, and the large M6 with 10 parameters can reach a better performance
- We propose a method called M6 that is able to process information of multiple modalities and perform both single-modal and cross-modal understanding and generation
- The model is scaled to large model with 10 billion parameters with sophisticated deployment, and the 10 -parameter M6-large is the largest pretrained model in Chinese
- Experimental results show that our proposed M6 outperforms the baseline in a number of downstream tasks concerning both single modality and multiple modalities We will continue the pretraining of extremely large models by increasing data to explore the limit of its performance
Upload PDF to Generate Summary
Must-Reading Tree
Example
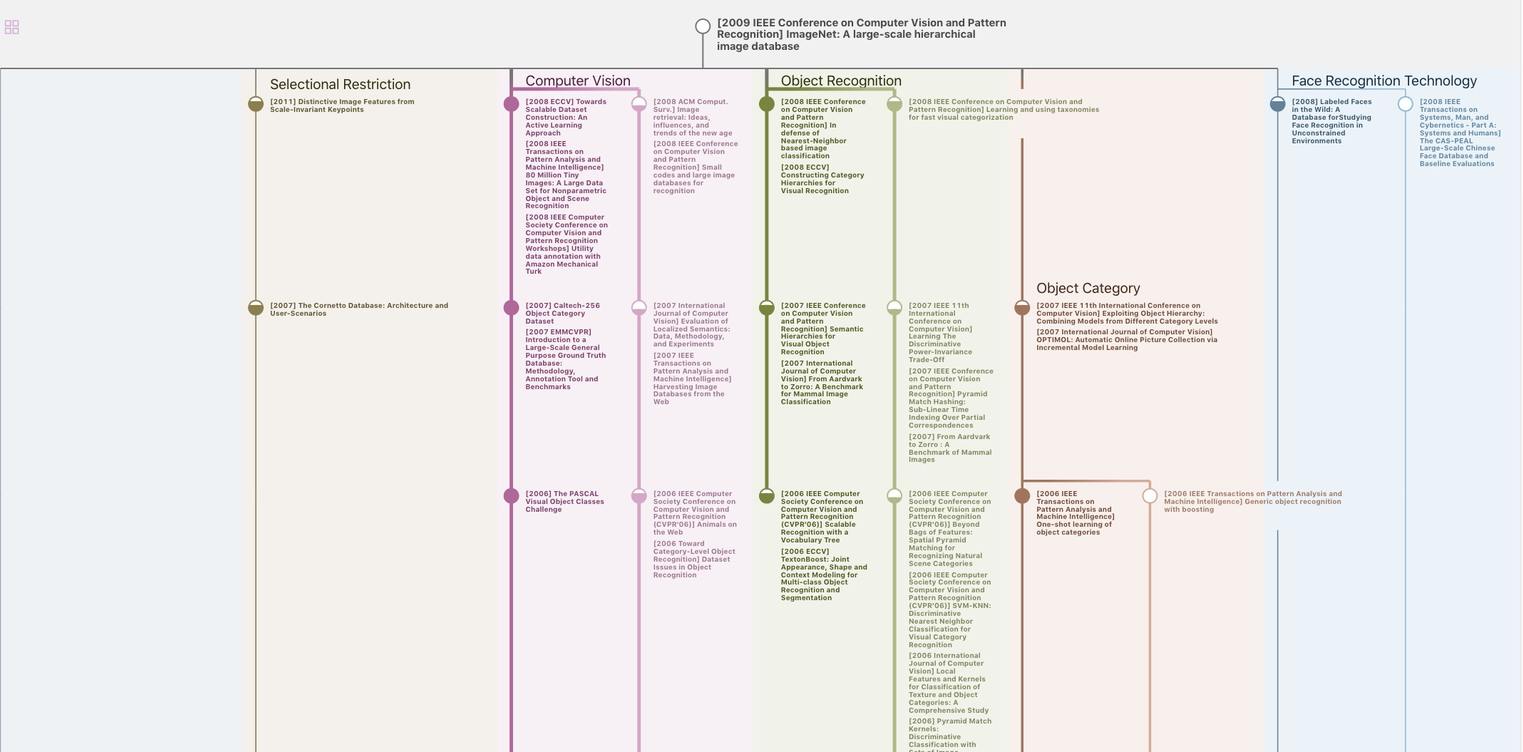
Generate MRT to find the research sequence of this paper
Data Disclaimer
The page data are from open Internet sources, cooperative publishers and automatic analysis results through AI technology. We do not make any commitments and guarantees for the validity, accuracy, correctness, reliability, completeness and timeliness of the page data. If you have any questions, please contact us by email: report@aminer.cn
Chat Paper
GPU is busy, summary generation fails
Rerequest