Achieving Synchronization and Chimera State of Modular Neural Networks by Using Dynamic Learning to Adjust Electromagnetic Induction
Nonlinear Dynamics(2024)
摘要
Synchronous firing of neurons in neural networks is of great physiological significance. Based on improved Hodgkin-Huxley model with electromagnetic induction, a modular neural network consisting of two small-world networks unidirectionally coupled is studied. By utilizing dynamic learning of synchronization (DLS) technique, it is shown that both synchronization and chimera states of modular neural networks can be achieved by dynamic adjustment of electromagnetic induction strength. Therefore, the generation of synchronized states can be controlled. By adjusting the electromagnetic induction strengths of all neurons in the network as a whole and in clusters, the global synchronization and cluster synchronization modes of the network are achieved, respectively. The chimera state is occurred by regulating the electromagnetic induction strength of part of the neurons in networks through DLS. When the first subnetwork is the chimera state mode, increasing the number of synchronized neurons makes the synchronization of second subnetwork more stable. The robustness of the DLS regulated synchronization mode is also demonstrated under different noise intensities and network topology. Our results showed that the DLS technique is highly effective in adjusting electromagnetic induction parameters to improve the synchronization of complex neural networks, which may provide new insights for various neural networks to transmit physiological information by utilizing synchronization state.
更多查看译文
关键词
Synchronization,Chimera,Modular neural network,Electromagnetic induction,Dynamic learning
AI 理解论文
溯源树
样例
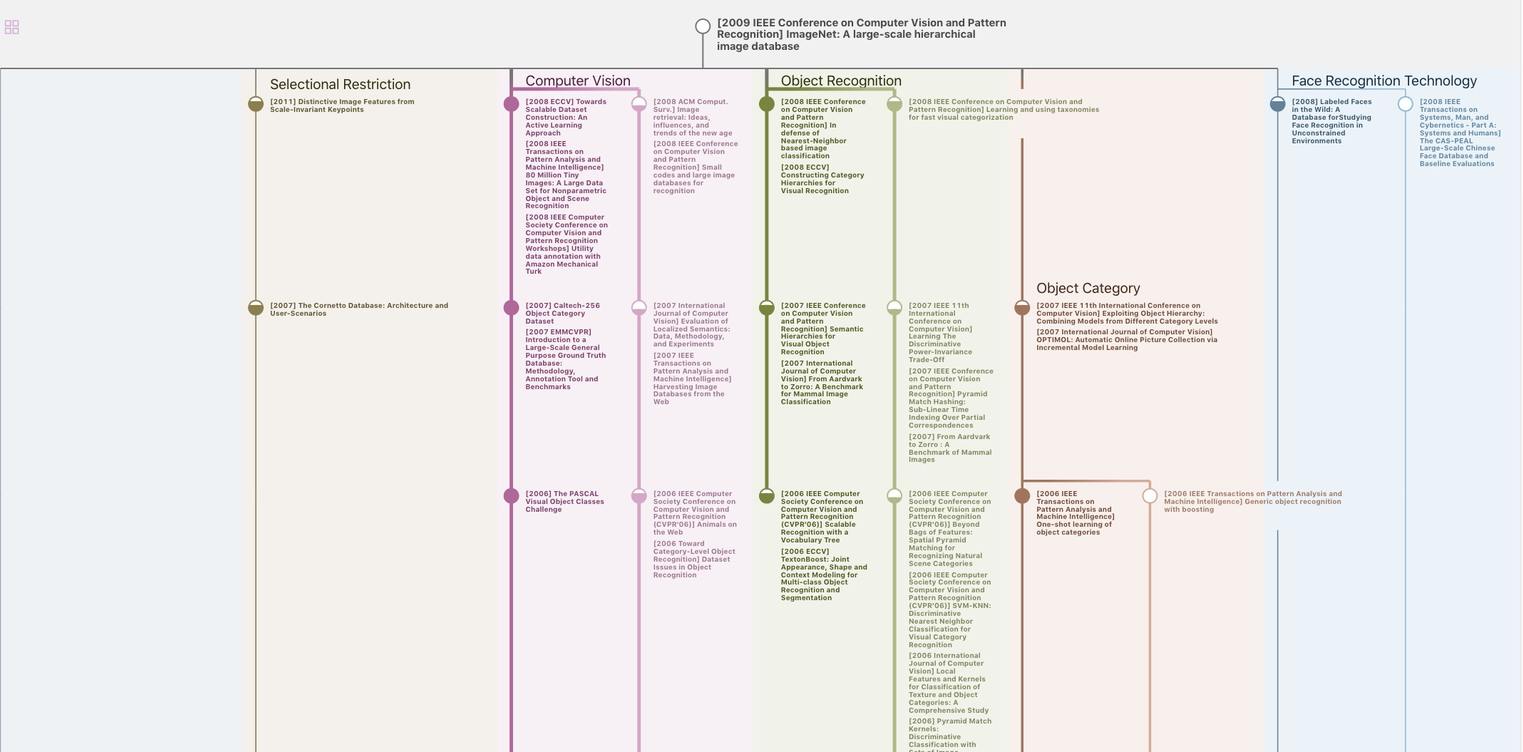
生成溯源树,研究论文发展脉络
Chat Paper
正在生成论文摘要