Income, Wealth, and Debt among Families with a History of Cancer.
JCO oncology practice(2024)SCI 3区SCI 4区
American Cancer Society | University of Virginia Comprehensive Cancer Center and School of Medicine | Department of Surveillance and Health Equity Science
Abstract
350 Background: Cancer survivors and their families often experience financial hardship due to high out-of-pocket medical costs and lost income from time away from work during treatment for patients and informal family caregivers. Little is known about detailed income, wealth, and debt among families with a history of cancer. Methods: The 2021 Panel Study of Income Dynamics was used to identify families with a cancer history. Family characteristics included survey response person’s age, sex, marital status, and race and ethnicity, number of dependent children living in the family, and time since cancer diagnosis. For families with multiple cancer diagnoses, time since cancer diagnosis was calculated according to the most recent cancer diagnosis. Family income was assessed by total income in tax year 2020, which consisted of combined taxable and transfer income of the response person, spouse/partner, and other family members. Wealth with and without equity (i.e., value of home minus mortgage) were assessed by sum of values of different types of assets (e.g. savings, checking, bonds, retirement accounts) net of debts (e.g., credit card debt, student loans, and medical bills). Results: A total of 701 families were identified with a cancer history. Within families, half of cancer survivors were 18-64 years and the other half were >65 years at the survey. About two thirds were married or living with partners, three fourths of response persons were male, one fifth had dependent children living in the same family, two thirds were non-Hispanic white, and two thirds of cancer survivors were diagnosed > 6 years prior to the survey. The median family income was $67,498 (inter-quartile range: $33,480 to $121,200), and the median wealth with and without equity were $188,000 and $5,5000, respectively. Moreover, 29.9% of families had credit debt (median: $4,500; inter-quartile range [IQR]: $1800-$14,000); 11.3% of families carried student loans (median: $4,500; IQR: $15,000-$60,000); and 10.7% of families had outstanding medical bills (median: $4,000; IQR: $2,000-$9,000). Conclusions: Families with a history of cancer have multiple types of debts, including medical debt, even years after diagnosis. Efforts to identify changes in wealth and income before and after cancer diagnosis are warranted. Income, wealth, and debt among families with a history of cancer. Family income Wealth without equity Wealth with equity Mean (Std) Median (IQR) Mean (Std) Median (IQR) Mean (Std) Median (IQR) $94,776(123,870) $67,498(33,480, 121,200) $459,956(1,142,647) $5,5000(4,000, 391,364) $649,912(1,307,959) $188,000(30,400, 645,000) Credit card debt (n=693) Student loans (n=698) Medical bills (n=627) n (%) Mean (Std) Median (IQR) n (%) Mean (Std) Median (IQR) n (%) Mean (Std) Median (IQR) 207(29.9%) $8,832 (10,300) $4,500(1800, 14,000) 79(11.3%) $55,948(75,256) $35,000(15,000, 60,000) 67(10.7%) $13,309(32,525) $4000(2,000, 9,000)
MoreTranslated text
求助PDF
上传PDF
View via Publisher
AI Read Science
AI Summary
AI Summary is the key point extracted automatically understanding the full text of the paper, including the background, methods, results, conclusions, icons and other key content, so that you can get the outline of the paper at a glance.
Example
Background
Key content
Introduction
Methods
Results
Related work
Fund
Key content
- Pretraining has recently greatly promoted the development of natural language processing (NLP)
- We show that M6 outperforms the baselines in multimodal downstream tasks, and the large M6 with 10 parameters can reach a better performance
- We propose a method called M6 that is able to process information of multiple modalities and perform both single-modal and cross-modal understanding and generation
- The model is scaled to large model with 10 billion parameters with sophisticated deployment, and the 10 -parameter M6-large is the largest pretrained model in Chinese
- Experimental results show that our proposed M6 outperforms the baseline in a number of downstream tasks concerning both single modality and multiple modalities We will continue the pretraining of extremely large models by increasing data to explore the limit of its performance
Upload PDF to Generate Summary
Must-Reading Tree
Example
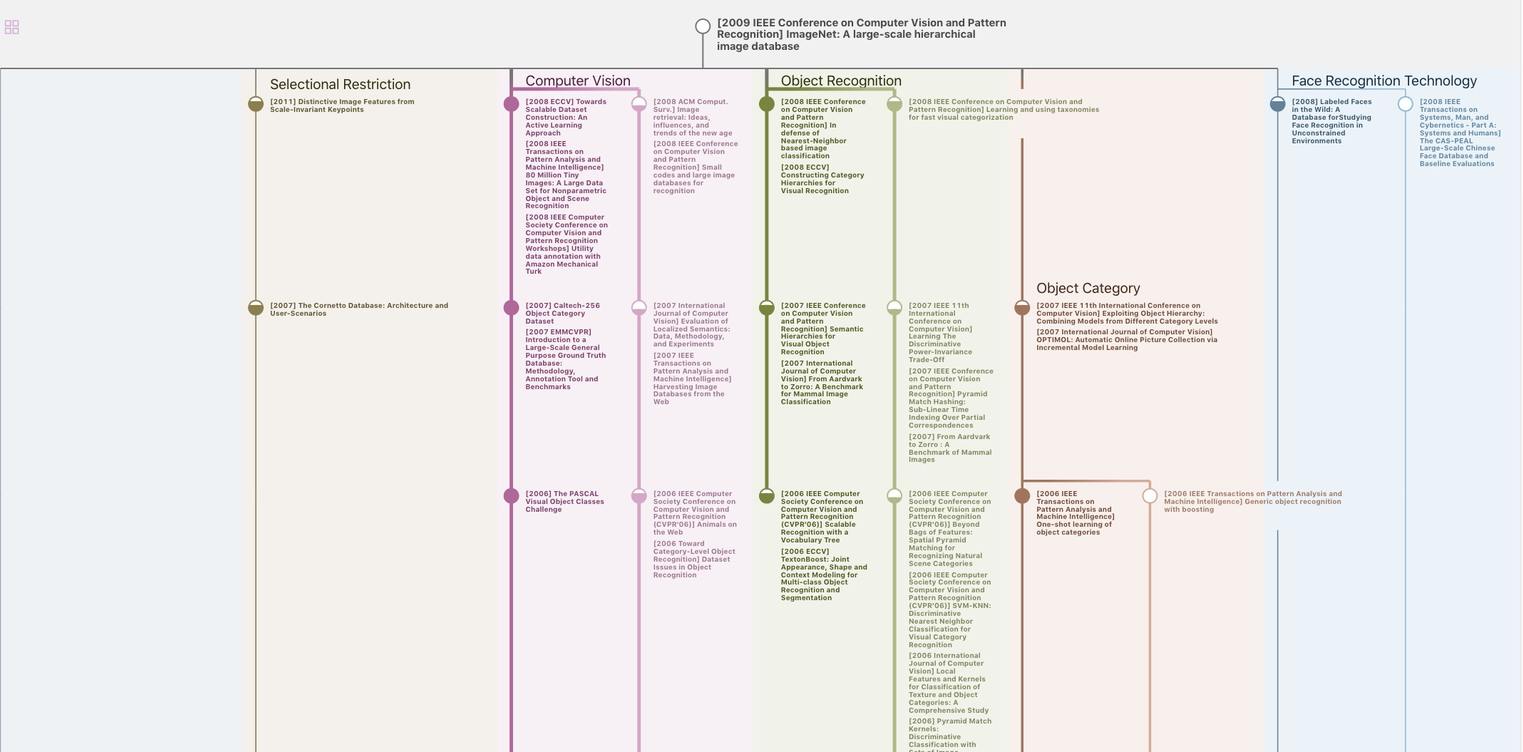
Generate MRT to find the research sequence of this paper
Data Disclaimer
The page data are from open Internet sources, cooperative publishers and automatic analysis results through AI technology. We do not make any commitments and guarantees for the validity, accuracy, correctness, reliability, completeness and timeliness of the page data. If you have any questions, please contact us by email: report@aminer.cn
Chat Paper
GPU is busy, summary generation fails
Rerequest