Software Using Artificial Intelligence for Nodule and Cancer Detection in CT Lung Cancer Screening: Systematic Review of Test Accuracy Studies
Thorax(2024)
摘要
OBJECTIVES:To examine the accuracy and impact of artificial intelligence (AI) software assistance in lung cancer screening using CT. METHODS:A systematic review of CE-marked, AI-based software for automated detection and analysis of nodules in CT lung cancer screening was conducted. Multiple databases including Medline, Embase and Cochrane CENTRAL were searched from 2012 to March 2023. Primary research reporting test accuracy or impact on reading time or clinical management was included. QUADAS-2 and QUADAS-C were used to assess risk of bias. We undertook narrative synthesis. RESULTS:Eleven studies evaluating six different AI-based software and reporting on 19 770 patients were eligible. All were at high risk of bias with multiple applicability concerns. Compared with unaided reading, AI-assisted reading was faster and generally improved sensitivity (+5% to +20% for detecting/categorising actionable nodules; +3% to +15% for detecting/categorising malignant nodules), with lower specificity (-7% to -3% for correctly detecting/categorising people without actionable nodules; -8% to -6% for correctly detecting/categorising people without malignant nodules). AI assistance tended to increase the proportion of nodules allocated to higher risk categories. Assuming 0.5% cancer prevalence, these results would translate into additional 150-750 cancers detected per million people attending screening but lead to an additional 59 700 to 79 600 people attending screening without cancer receiving unnecessary CT surveillance. CONCLUSIONS:AI assistance in lung cancer screening may improve sensitivity but increases the number of false-positive results and unnecessary surveillance. Future research needs to increase the specificity of AI-assisted reading and minimise risk of bias and applicability concerns through improved study design. PROSPERO REGISTRATION NUMBER:CRD42021298449.
更多查看译文
AI 理解论文
溯源树
样例
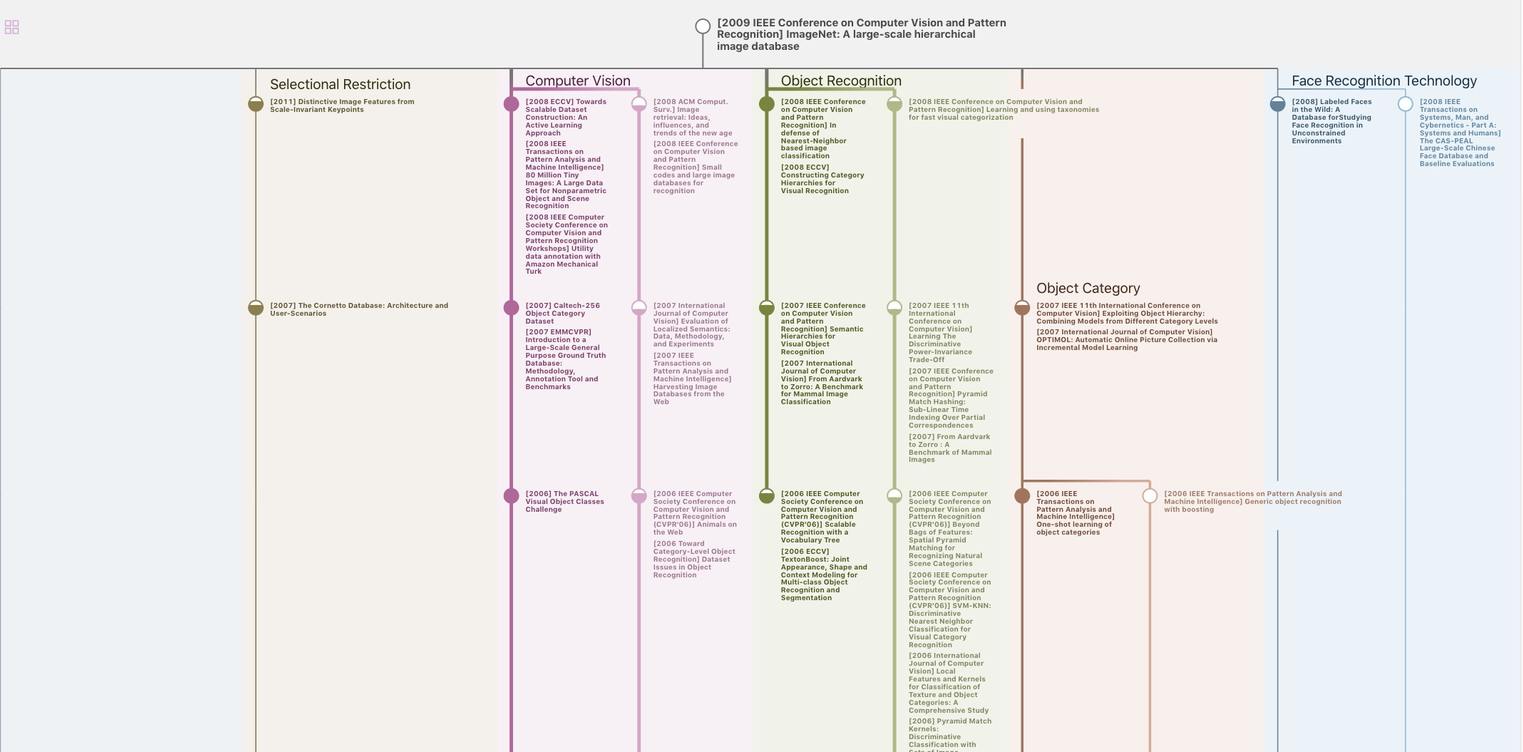
生成溯源树,研究论文发展脉络
Chat Paper
正在生成论文摘要