FLaRe: Achieving Masterful and Adaptive Robot Policies with Large-Scale Reinforcement Learning Fine-Tuning
arxiv(2024)
Abstract
In recent years, the Robotics field has initiated several efforts toward building generalist robot policies through large-scale multi-task Behavior Cloning. However, direct deployments of these policies have led to unsatisfactory performance, where the policy struggles with unseen states and tasks. How can we break through the performance plateau of these models and elevate their capabilities to new heights? In this paper, we propose FLaRe, a large-scale Reinforcement Learning fine-tuning framework that integrates robust pre-trained representations, large-scale training, and gradient stabilization techniques. Our method aligns pre-trained policies towards task completion, achieving state-of-the-art (SoTA) performance both on previously demonstrated and on entirely novel tasks and embodiments. Specifically, on a set of long-horizon mobile manipulation tasks, FLaRe achieves an average success rate of 79.5 simulation and +30.7 sparse rewards, our approach can enable generalizing to new capabilities beyond the pretraining data with minimal human effort. Moreover, we demonstrate rapid adaptation to new embodiments and behaviors with less than a day of fine-tuning. Videos can be found on the project website at https://robot-flare.github.io/
MoreTranslated text
AI Read Science
Must-Reading Tree
Example
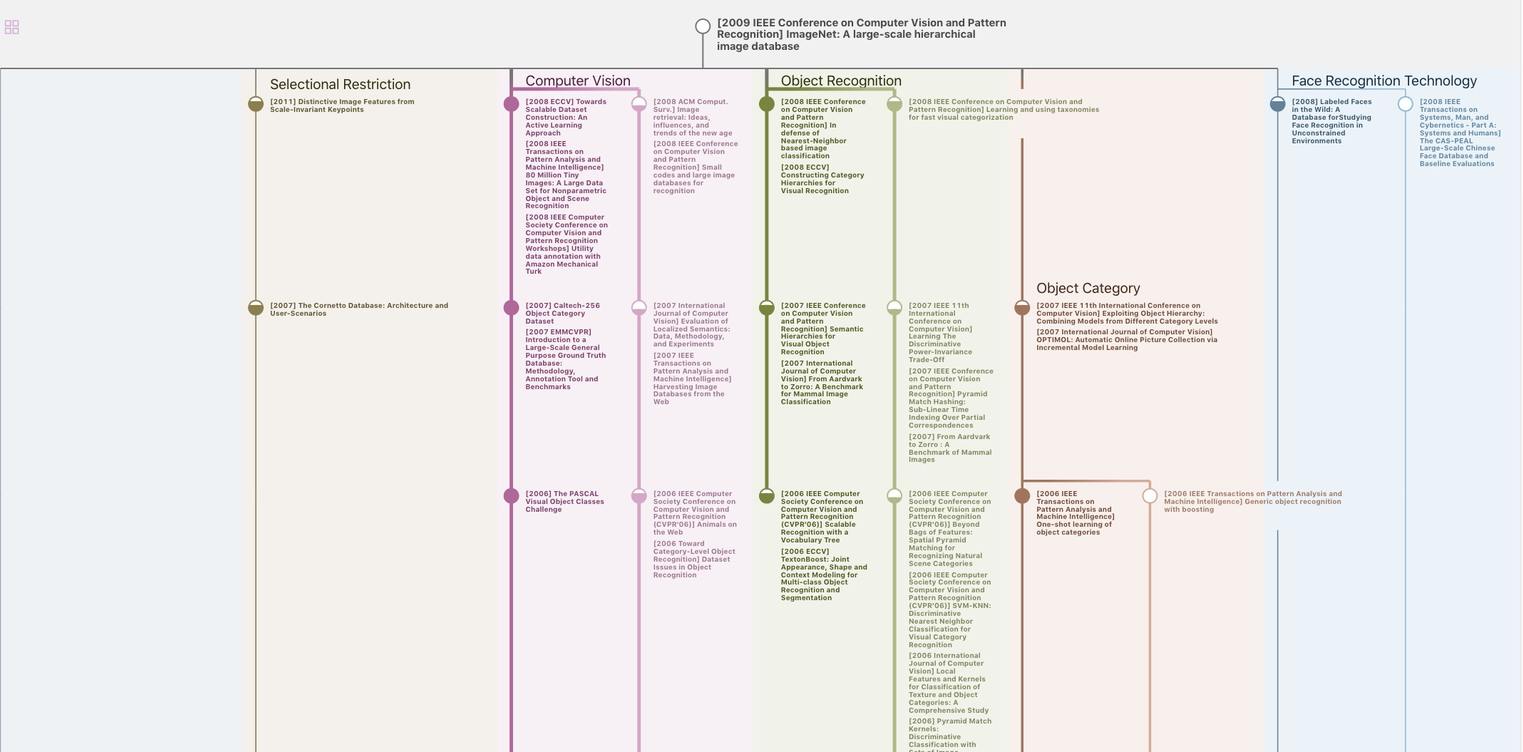
Generate MRT to find the research sequence of this paper
Chat Paper
Summary is being generated by the instructions you defined