LASERBEAK: Evolving Website Fingerprinting Attacks with Attention and Multi-Channel Feature Representation
IEEE Transactions on Information Forensics and Security(2024)
摘要
In this paper, we present LASERBEAK, a new state-of-the-art website fingerprinting attack for Tor that achieves nearly 96% accuracy against FRONT-defended traffic by combining two innovations: (i) multi-channel traffic representations and (ii) advanced techniques adapted from state-of-the-art computer vision models. Our work is the first to explore a range of different ways to represent traffic data for a classifier. We find a multi-channel input format that provides richer contextual information, enabling the model to learn robust representations even in the presence of heavy traffic obfuscation. We are also the first to examine how recent advances in transformer models can take advantage of these representations. Our novel model architecture utilizing multi-headed attention layers enhances the capture of both local and global patterns. By combining these innovations, LASERBEAK demonstrates absolute performance improvements of up to 36.2% (e.g., from 27.6% to 63.8%) compared with prior attacks against defended traffic. Experiments highlight LASERBEAK’s capabilities in multiple scenarios, including a large open-world dataset where it achieves over 80% recall at 99% precision on traffic obfuscated with padding defenses. These advances reduce the remaining anonymity in Tor against fingerprinting threats, underscoring the need for stronger defenses.
更多查看译文
关键词
Website Fingerprinting,Privacy,Anonymity,Tor,Deep Learning,Transformer Neural Network
AI 理解论文
溯源树
样例
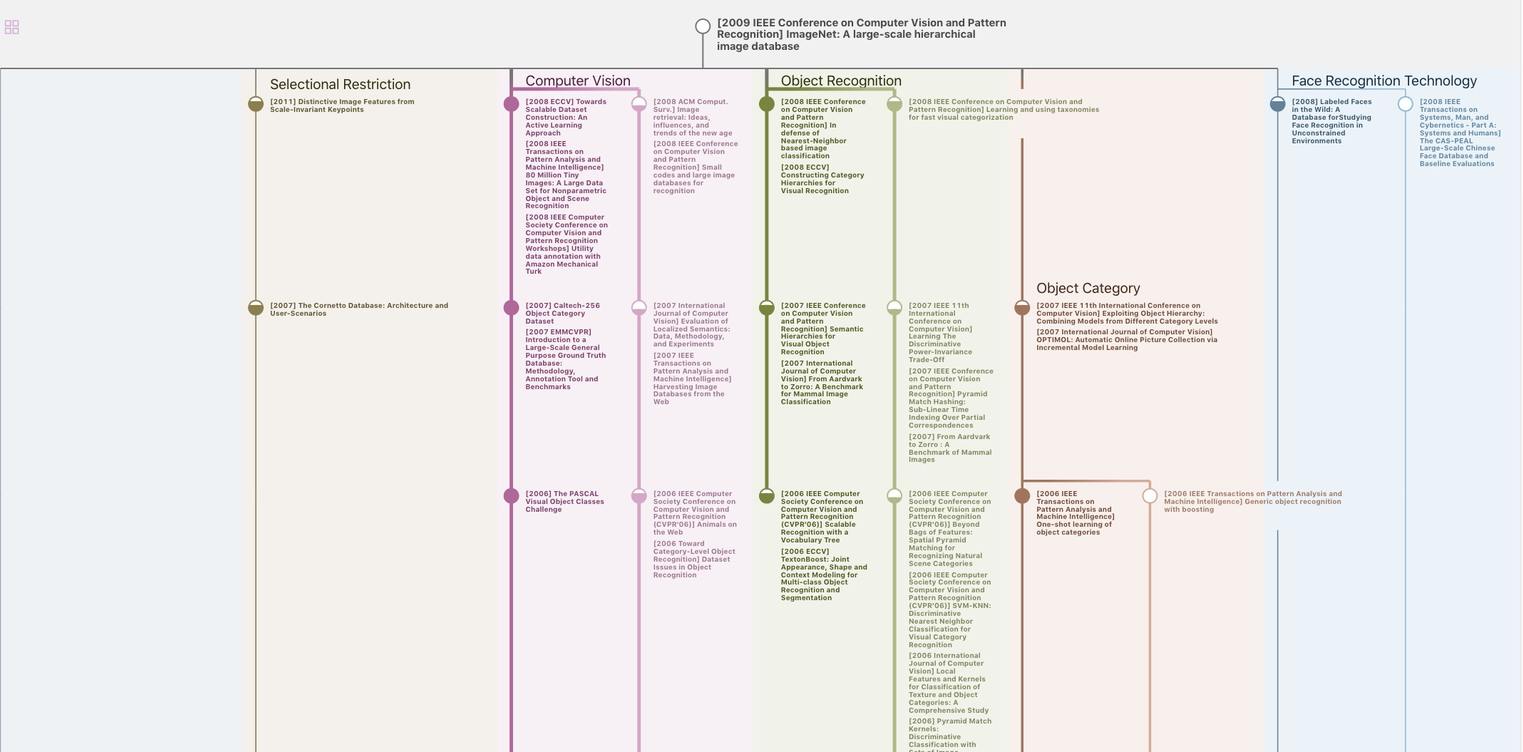
生成溯源树,研究论文发展脉络
Chat Paper
正在生成论文摘要