An Improved Transformer Method for Prediction of Aircraft Hard Landing Based on QAR Data
International Journal of Aeronautical and Space Sciences(2024)
摘要
In the civil aviation industry, hard landing is a typical unsafety event of great concern to airlines and civil aviation authorities, as their misidentification can potentially lead to catastrophic accidents. Therefore, this paper presents an innovative predictive model for aircraft hard landing events based on Quick Access Recorder (QAR) data. Initially, 44 feature parameters most relevant to hard landing incidents are selected from over 3,000 parameters collected by aircraft sensors, guided by flight dynamics and feature engineering principles. A data preprocessing process is proposed for the high heterogeneity of QAR data, including data cleaning, interpolation, and normalization steps. Subsequently, leveraging the filtered and processed sensor data, an improved transformer-based deep learning prediction model is established to predict hard landing events. The optimal model is determined by adjusting the model's structural parameters and conducting comparative experiments, with RMSE and MAPE serving as evaluation criteria. The experimental results demonstrate that the model exhibits superior performance and higher prediction accuracy than the existing model on the QAR dataset, indicating that the model is effective and accurate in predicting hard landing of aircraft, which contributes to ensuring passenger safety and reducing the occurrence of hard landing accidents. Moreover, the proposed method fosters innovation in the construction and advancement of Industrial Internet of Things (IoT) systems within the aviation field.
更多查看译文
关键词
Improved transformer,Hard landing prediction,Time-series QAR data,Convolutional neural network,Gated recurrent unit,Flight dynamics
AI 理解论文
溯源树
样例
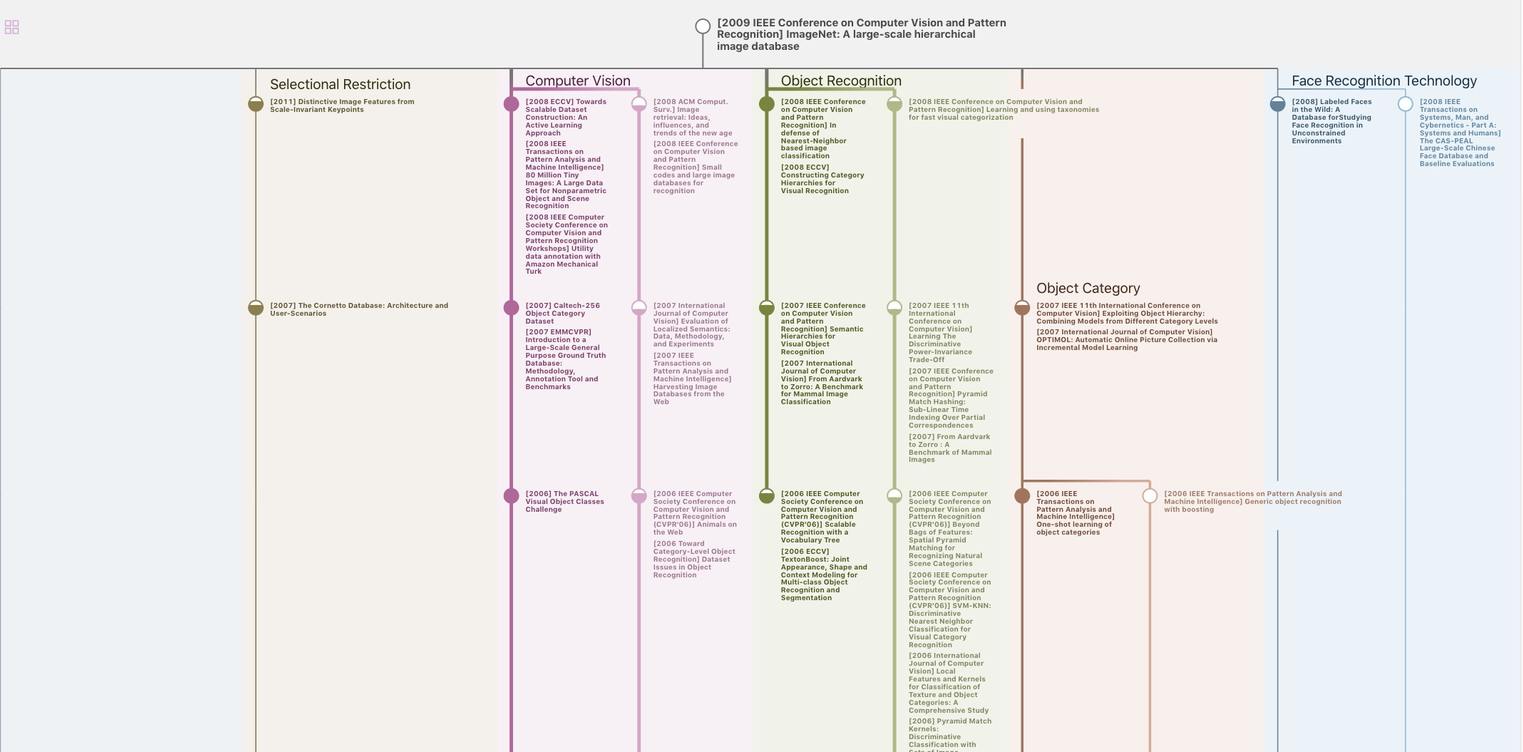
生成溯源树,研究论文发展脉络
Chat Paper
正在生成论文摘要