Sketched Lanczos Uncertainty Score: a Low-Memory Summary of the Fisher Information
arxiv(2024)
摘要
Current uncertainty quantification is memory and compute expensive, which hinders practical uptake. To counter, we develop Sketched Lanczos Uncertainty (SLU): an architecture-agnostic uncertainty score that can be applied to pre-trained neural networks with minimal overhead. Importantly, the memory use of SLU only grows logarithmically with the number of model parameters. We combine Lanczos' algorithm with dimensionality reduction techniques to compute a sketch of the leading eigenvectors of a matrix. Applying this novel algorithm to the Fisher information matrix yields a cheap and reliable uncertainty score. Empirically, SLU yields well-calibrated uncertainties, reliably detects out-of-distribution examples, and consistently outperforms existing methods in the low-memory regime.
更多查看译文
AI 理解论文
溯源树
样例
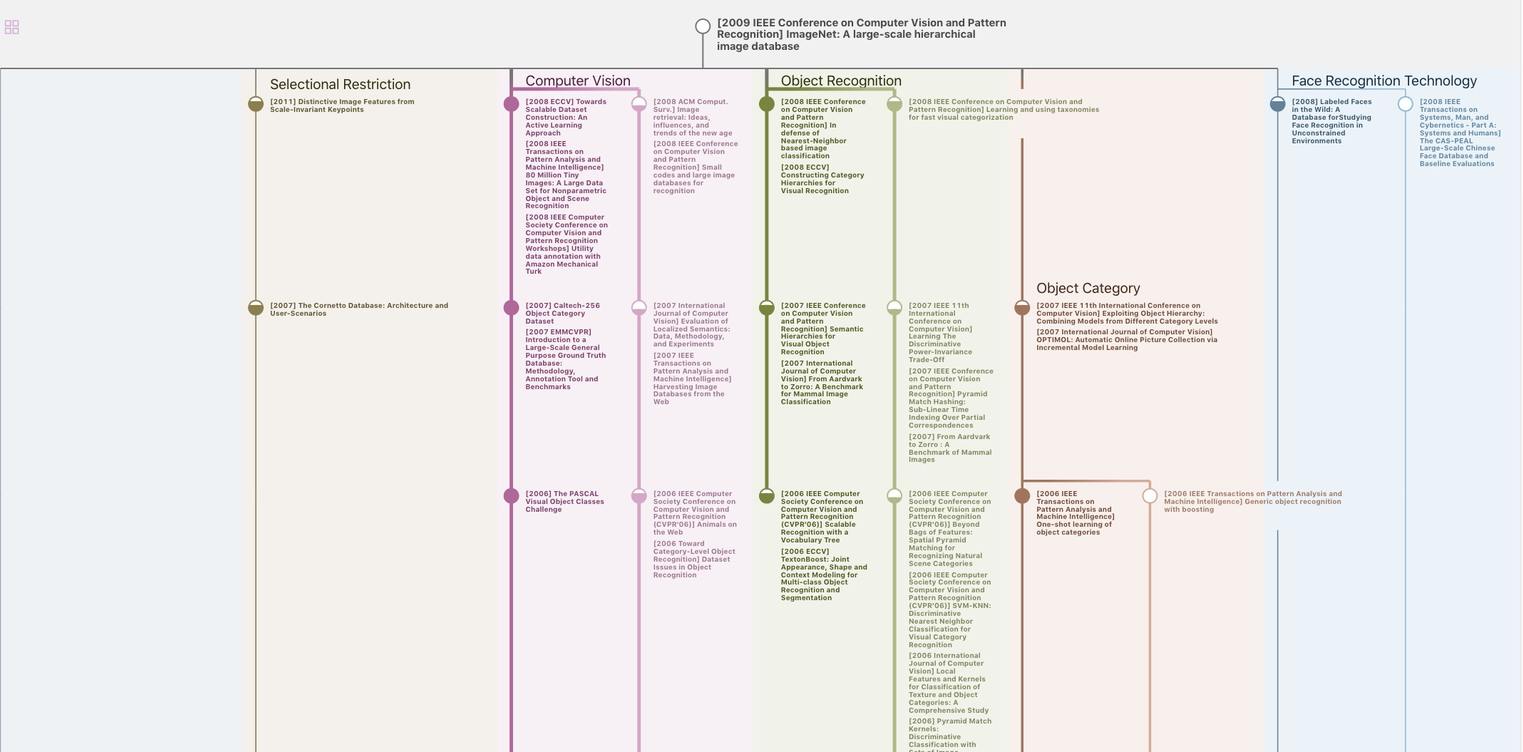
生成溯源树,研究论文发展脉络
Chat Paper
正在生成论文摘要