Research Status of Electromagnetic Field-Assisted Laser Cladding Technology
International Journal of Mechanical and Electrical Engineering(2024)
Abstract
Laser cladding technology, renowned for its superior surface modification capabilities, is extensively utilized across aerospace, automotive, energy, chemical, metal products, and other heavy industrial sectors. However, issues such as porosity, cracks, and the non-uniformity of the cladding layer's composition and microstructure significantly hinder the advancement of this technology. Therefore, improving and enhancing the quality of the cladding layer is of paramount research importance. In recent years, there has been a growing body of research on the use of external physical fields to assist laser cladding. This research has expanded beyond the application of single physical fields to include the integration of electromagnetic fields, electromagnetic ultrasonic fields, and other coupled physical fields, which have effectively addressed the current challenges faced by laser cladding technology. This paper provides a comprehensive review of the progress made in the field of electromagnetic field-assisted laser cladding, based on an introduction to the principles and mechanisms of electromagnetic field assistance.
MoreTranslated text
PDF
View via Publisher
AI Read Science
AI Summary
AI Summary is the key point extracted automatically understanding the full text of the paper, including the background, methods, results, conclusions, icons and other key content, so that you can get the outline of the paper at a glance.
Example
Background
Key content
Introduction
Methods
Results
Related work
Fund
Key content
- Pretraining has recently greatly promoted the development of natural language processing (NLP)
- We show that M6 outperforms the baselines in multimodal downstream tasks, and the large M6 with 10 parameters can reach a better performance
- We propose a method called M6 that is able to process information of multiple modalities and perform both single-modal and cross-modal understanding and generation
- The model is scaled to large model with 10 billion parameters with sophisticated deployment, and the 10 -parameter M6-large is the largest pretrained model in Chinese
- Experimental results show that our proposed M6 outperforms the baseline in a number of downstream tasks concerning both single modality and multiple modalities We will continue the pretraining of extremely large models by increasing data to explore the limit of its performance
Try using models to generate summary,it takes about 60s
Must-Reading Tree
Example
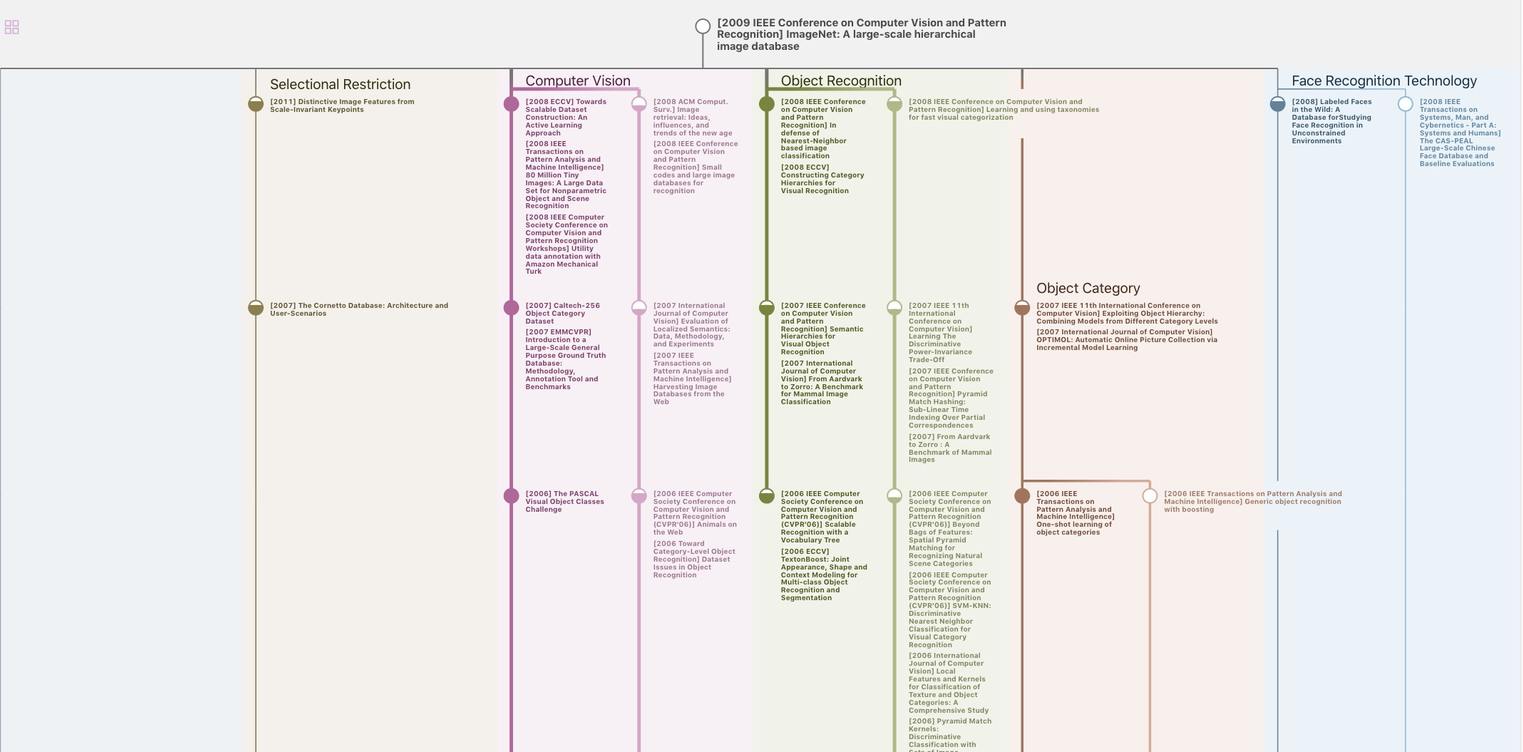
Generate MRT to find the research sequence of this paper
Data Disclaimer
The page data are from open Internet sources, cooperative publishers and automatic analysis results through AI technology. We do not make any commitments and guarantees for the validity, accuracy, correctness, reliability, completeness and timeliness of the page data. If you have any questions, please contact us by email: report@aminer.cn
Chat Paper
去 AI 文献库 对话