Artificial Intelligence-Enhanced Wearable Technology Enables Ventricular Arrhythmia Prediction
European Heart Journal - Digital Health(2024)
摘要
Abstract Background and aims Patterns in physical behaviour may be associated with an increased risk of ventricular arrhythmia. We examined associations between temporal dynamics in physical behaviour measured through wearable technology, and the risk of ventricular arrhythmia. Methods The multicenter, prospective SafeHeart study recruited 303 patients with an implantable cardioverter defibrillator (ICD) from May 2021 to September 2022. Continuous physical behaviour data were collected over 365 consecutive days and nights using wearable accelerometers. We characterised each 28-day behavioural time-series using statistical summary indices, and deep representations learned by neural networks. Logistic regression analyses were used to estimate the associations between physical behaviour and the subsequent risk of ventricular arrhythmia as detected by ICD and treated appropriately. Predictive performance was assessed using k-fold cross-validation and quantified by the area under the receiver operating characteristic curve (AUROC). Results From the SafeHeart cohort, 277 patients and 56 ventricular arrhythmia were analysed, contributing to a total of 64,995 days of behavioural data. Reduced numbers of daily physical activity bouts and low day-to-day variation in sleep characteristics were associated with an increased risk of an arrhythmic event. Deep representations improved the predictive performance, with an AUROC of 0.74 ±0.05, compared to statistical summary indices with an AUROC 0.67 ±0.14 (p=0.05 for comparison). Conclusion Our findings suggest that behavioural patterns captured through wearable technology are independently associated with ventricular arrhythmia, particularly when these are characterised by deep representations.
更多查看译文
AI 理解论文
溯源树
样例
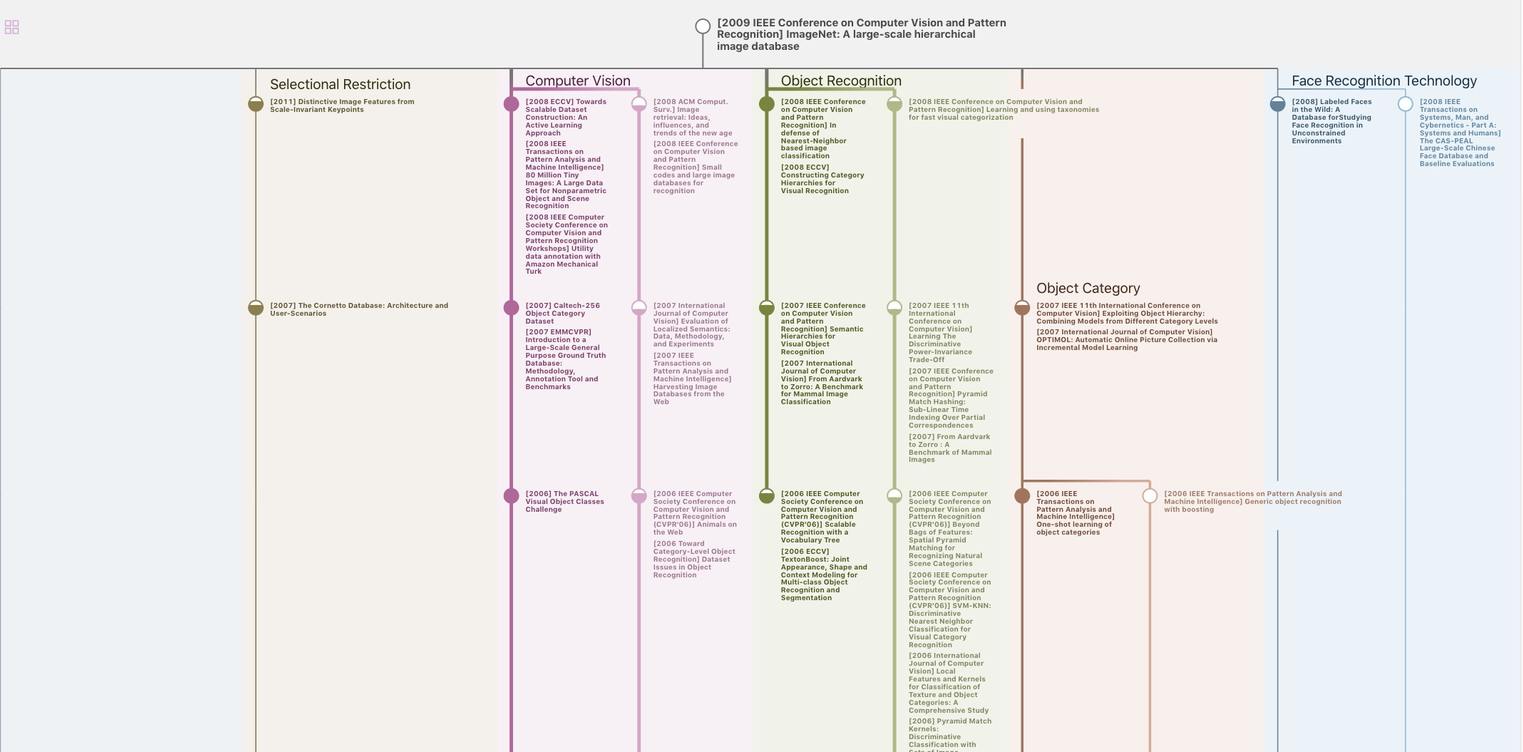
生成溯源树,研究论文发展脉络
Chat Paper
正在生成论文摘要