Spatio-Temporal Dynamic Attention Graph Convolutional Network Based on Skeleton Gesture Recognition
Electronics(2024)
摘要
Dynamic gesture recognition based on skeletal data has garnered significant attention with the rise of graph convolutional networks (GCNs). Existing methods typically calculate dependencies between joints and utilize spatio-temporal attention features. However, they often rely on joint topological features of limited spatial extent and short-time features, making it challenging to extract intra-frame spatial features and long-term inter-frame temporal features. To address this, we propose a new GCN architecture for dynamic hand gesture recognition, called a spatio-temporal dynamic attention graph convolutional network (STDA-GCN). This model employs dynamic attention spatial graph convolution, enhancing spatial feature extraction capabilities while reducing computational complexity through improved cross-channel information interaction. Additionally, a salient location channel attention mechanism is integrated between spatio-temporal convolutions to extract useful spatial features and avoid redundancy. Finally, dynamic multi-scale temporal convolution is used to extract richer inter-frame gesture features, effectively capturing information across various time scales. Evaluations on the SHREC’17 Track and DHG-14/28 benchmark datasets show that our model achieves 97.14% and 95.84% accuracy, respectively. These results demonstrate the superior performance of STDA-GCN in dynamic gesture recognition tasks.
更多查看译文
关键词
dynamic hand gesture recognition,deep learning,graph convolutional network,channel attention,hand skeleton points
AI 理解论文
溯源树
样例
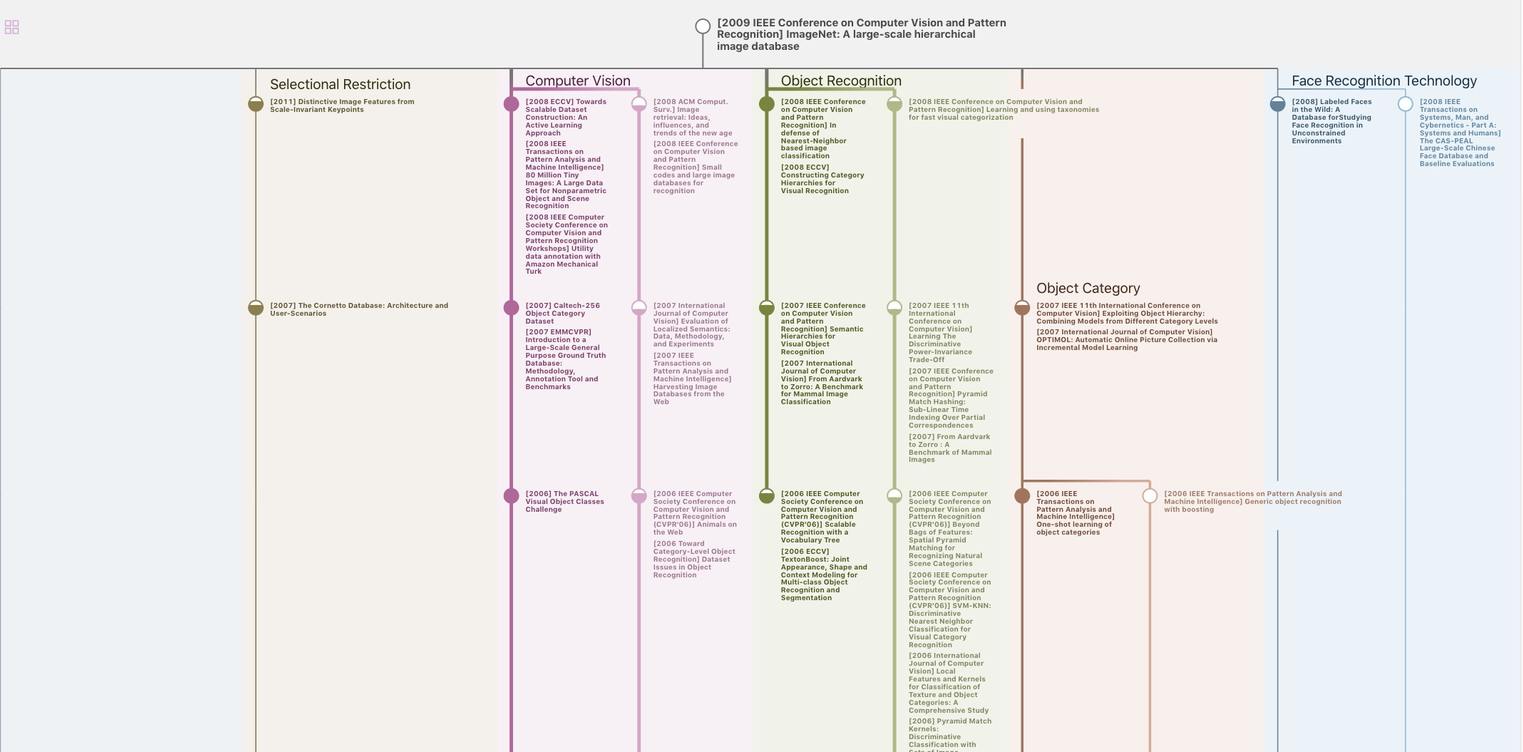
生成溯源树,研究论文发展脉络
Chat Paper
正在生成论文摘要