Prediction of Catalytic Performance of Metal Oxide Catalysts for Alkyne Hydrogenation Reaction Based on Machine Learning
Applied Catalysis A General(2024)
摘要
In the field of catalyst design, machine learning is gaining significant attention, especially in situations where data is limited. Facing this challenge, we have developed a neural network model that enhances predictive accuracy through the careful selection of catalyst descriptors and feature engineering, aiming to predict the conversion rate and selectivity of the acetylene semi-hydrogenation reaction. Our model has identified promising metal oxide catalysts, such as CuO, ZnO, and V2O5, for the acetylene semi-hydrogenation reaction, and these predictions have been experimentally validated. Among them, CuO achieved an acetylene conversion of 99.6% and an ethylene selectivity of 90% at 50-100°C, which is unprecedented at the reaction space velocity we tested. This high activity at relatively low temperatures indicates a more promising industrial application. Looking ahead, machine learning will play a pivotal role in catalyst design, accelerating the discovery and industrial application of new materials.
更多查看译文
关键词
machine learning,catalysis,metal oxide catalysts,alkyne hydrogenation,predictive modeling,CuO
AI 理解论文
溯源树
样例
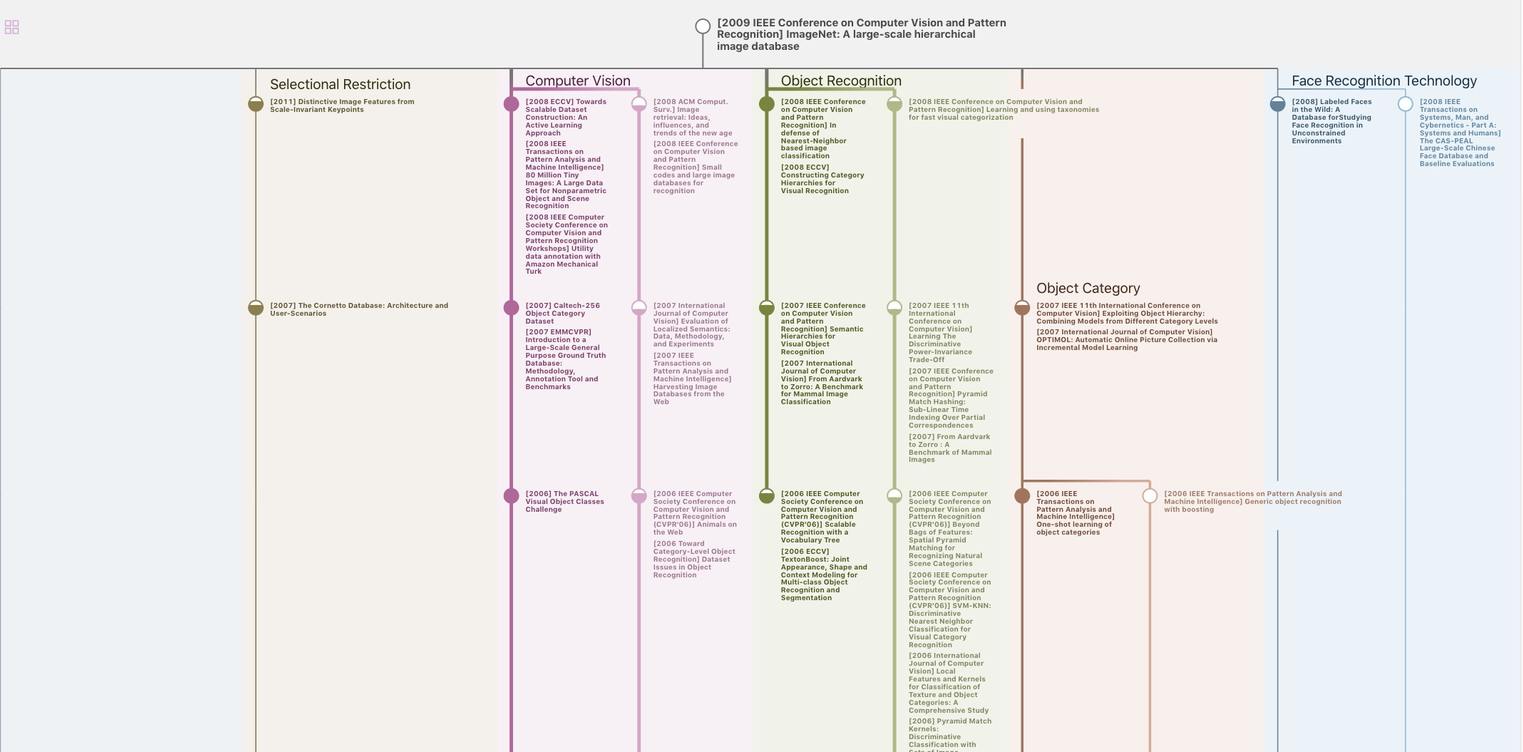
生成溯源树,研究论文发展脉络
Chat Paper
正在生成论文摘要