Advances in Spatial Resolution and Radiation Dose Reduction Using Super-Resolution Deep Learning–based Reconstruction for Abdominal Computed Tomography: A Phantom Study
Academic Radiology(2024)
摘要
Rationale and Objectives This study evaluated the performance of super-resolution deep learning-based reconstruction (SR-DLR) and compared with it that of hybrid iterative reconstruction (HIR) and normal-resolution DLR (NR-DLR) for enhancing image quality in computed tomography (CT) images across various field of view (FOV) sizes, radiation doses, and noise reduction strengths. Materials and Methods A Catphan phantom equipped with an external body ring was used. CT images were reconstructed using filtered back-projection (FBP), HIR, NR-DLR, and SR-DLR across three noise reduction strengths: mild, standard, and strong. The noise power spectrum (NPS) was obtained from the FBP, HIR, NR-DLR, and SR-DLR images at various FOVs, radiation doses, and noise reduction strengths. The noise magnitude ratio (NMR) and central frequency ratio (CFR) were calculated from the HIR, NR-DLR, and SR-DLR images relative to the FBP images using NPS. The high-contrast value was obtained from the amplitude values of the peaks and valleys of profile curve and the task-based transfer function were also analyzed. Results SR-DLR consistently demonstrated superior noise reduction capabilities, with NMR of 0.29–0.36 at reduced dose and 0.35–0.45 at standard dose, outperforming HIR and showing comparable efficiency to NR-DLR. The high-contrast values for SR-DLR were highest at mild and standard levels for both low and standard doses (0.610 and 0.726 at mild and 0.725 and 0.603 at standard levels). At the standard dose, the spatial resolution of SR-DLR was significantly improved, regardless of the noise reduction strength and FOV. Conclusion SR-DLR images achieved more substantial noise reduction than HIR and similar noise reduction as NR-DLR reconstructions while also improving spatial resolution.
更多查看译文
关键词
Super-resolution deep learning based reconstruction,Spatial resolution,Noise reduction,Noise texture,Computed tomography
AI 理解论文
溯源树
样例
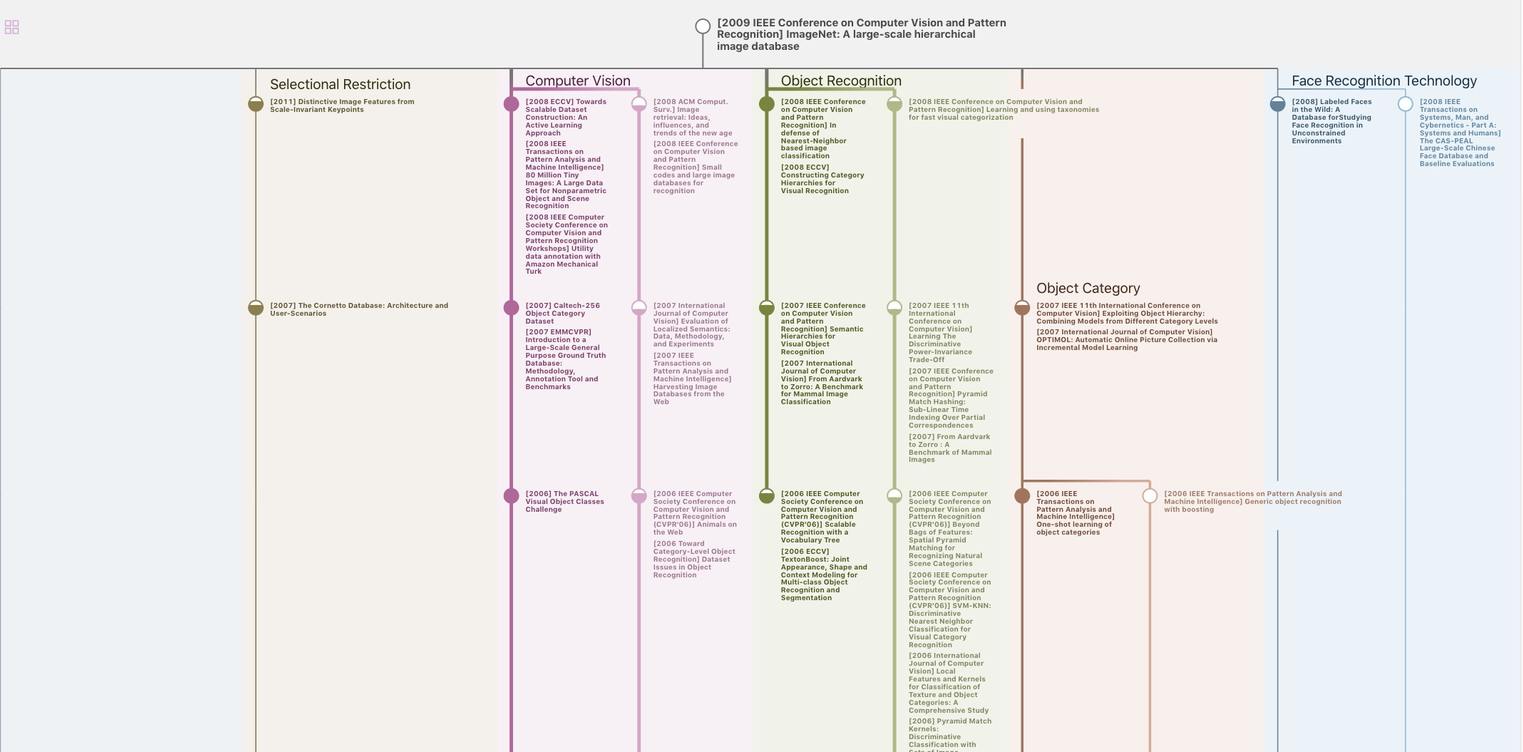
生成溯源树,研究论文发展脉络
Chat Paper
正在生成论文摘要