Dataset Construction for Machine Learning Based Wired Networks Prognosis
2024 Prognostics and System Health Management Conference (PHM)(2024)
CEA | UVSQ
Abstract
Prognostic methods leveraging Machine Learning (ML) require consistent and complete datasets to achieve accurate predictions. However, in the context of cable prognosis based on reflectometry, assembling such datasets is challenging due to the prolonged process of accumulating real condition monitoring data over the lifespan of cables and also the lack of such available datasets in the literature to the best of our knowledge. Hence, this paper proposes a solution for relevant data generation to simulate the degradation of cables during their lifetime. This initial step is highly important in our proposed cable prognosis methodology, which integrates distributed reflectometry sensors and ML techniques to estimate the remaining useful life of multi-branched wired networks. To do so, two use cases of cable aging, using model-driven and experimental approaches, are studied in this paper. As a conclusion, a hybrid approach will be considered based on accelerated aging experiments and simulations to set up a pertinent dataset and provide a deeper understanding of the cable degradation process.
MoreTranslated text
Key words
Cable prognosis,remaining useful life,machine learning,reflectometry,prognostics and health management
求助PDF
上传PDF
PPT
Code
Data
View via Publisher
AI Read Science
AI Summary
AI Summary is the key point extracted automatically understanding the full text of the paper, including the background, methods, results, conclusions, icons and other key content, so that you can get the outline of the paper at a glance.
Example
Background
Key content
Introduction
Methods
Results
Related work
Fund
Key content
- Pretraining has recently greatly promoted the development of natural language processing (NLP)
- We show that M6 outperforms the baselines in multimodal downstream tasks, and the large M6 with 10 parameters can reach a better performance
- We propose a method called M6 that is able to process information of multiple modalities and perform both single-modal and cross-modal understanding and generation
- The model is scaled to large model with 10 billion parameters with sophisticated deployment, and the 10 -parameter M6-large is the largest pretrained model in Chinese
- Experimental results show that our proposed M6 outperforms the baseline in a number of downstream tasks concerning both single modality and multiple modalities We will continue the pretraining of extremely large models by increasing data to explore the limit of its performance
Upload PDF to Generate Summary
Must-Reading Tree
Example
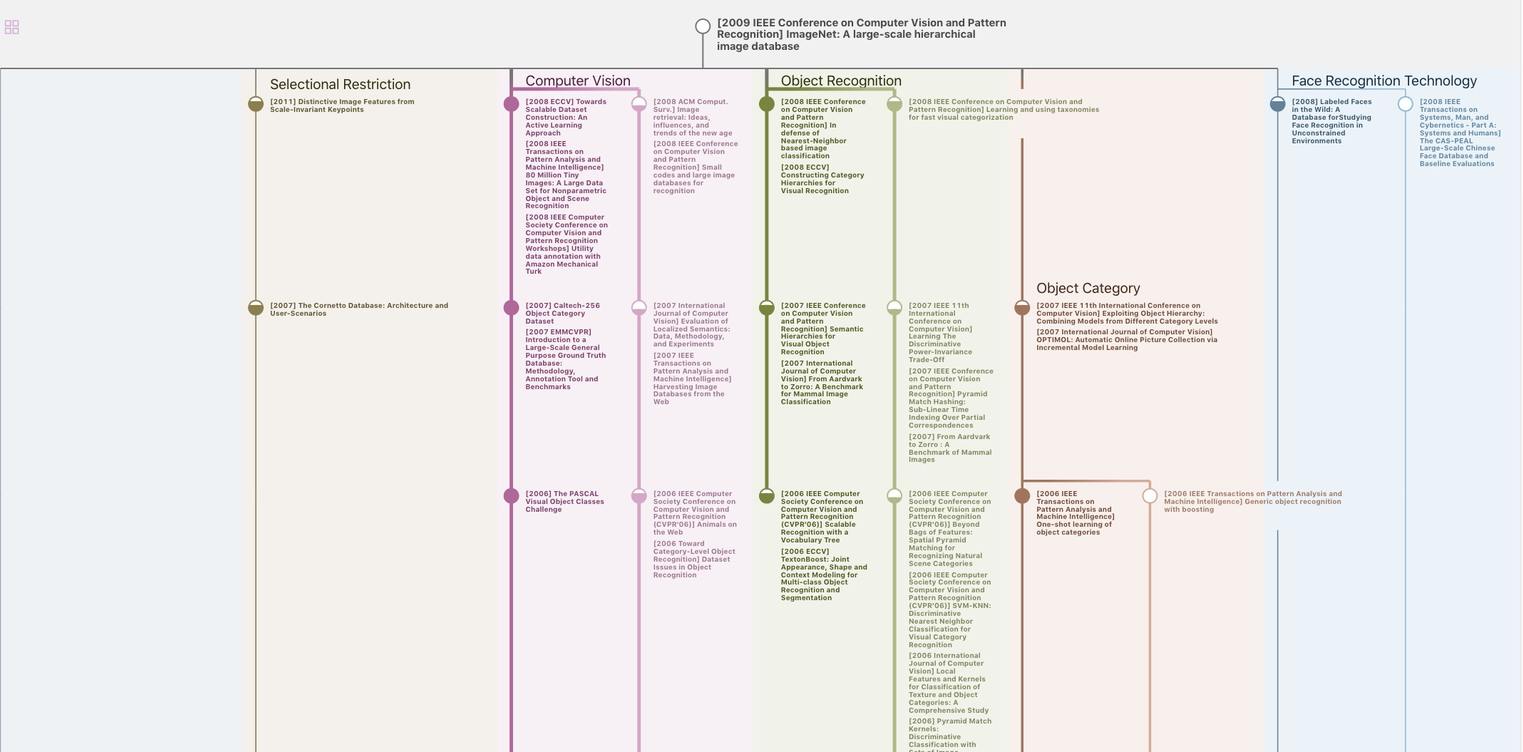
Generate MRT to find the research sequence of this paper
Data Disclaimer
The page data are from open Internet sources, cooperative publishers and automatic analysis results through AI technology. We do not make any commitments and guarantees for the validity, accuracy, correctness, reliability, completeness and timeliness of the page data. If you have any questions, please contact us by email: report@aminer.cn
Chat Paper
Summary is being generated by the instructions you defined