Tree Search Reinforcement Learning for Two-Dimensional Cutting Stock Problem with Complex Constraints
IEEE Transactions on Automation Science and Engineering(2024)
摘要
Reinforcement learning (RL) has been widely used in recent years to solve combinatorial optimization problems; however, it has some limitations when solving such problems with practical features. It is difficult for RL to ensure the feasibility of solutions when solving optimization problems with complex constraints, which limits its industrial application. Using a two-dimensional cutting stock problem derived from the practical plate design process in the steel industry as an example, we propose a learning and searching framework that enables RL to obtain a feasible solution that obeys complex constraints. We first formulate the two-dimensional cutting stock problem as a Markov decision process (MDP) and then design a tree-search-based reinforcement learning (TSRL) algorithm within the proposed learning and searching framework. In the learning process, we establish the approximate stage-independent Bellman equation (ASIB) of the MDP and obtain the agent decision model by solving the ASIB with approximate linear programming and column generation. In the search process, based on the obtained approximate value function, an efficient parallel two-stage tree search is developed as the agent decision generator of RL to obtain near-optimal plate design schemes. The experimental results show that the proposed TSRL algorithm outperforms baselines in terms of the capacity to obtain a feasible solution, computational time, and solution quality. Note to Practitioners —This article was motivated by the slab design problem in the steel industry but it also applied to other two-dimensional cutting stock problems with complex constraints. This work promotes making decisions in industrial production. Our key contribution is to provide a good-enough solution in a short time, which can improve the resource utilization and reduce the production cost of industrial enterprises. In terms of methodology, we provide new insight into reinforcement learning, which makes reinforcement learning more applicable to practice scenarios.
更多查看译文
关键词
Reinforcement learning,complex constraints,two-dimensional cutting stock problem,parallel tree search,supermodularity
AI 理解论文
溯源树
样例
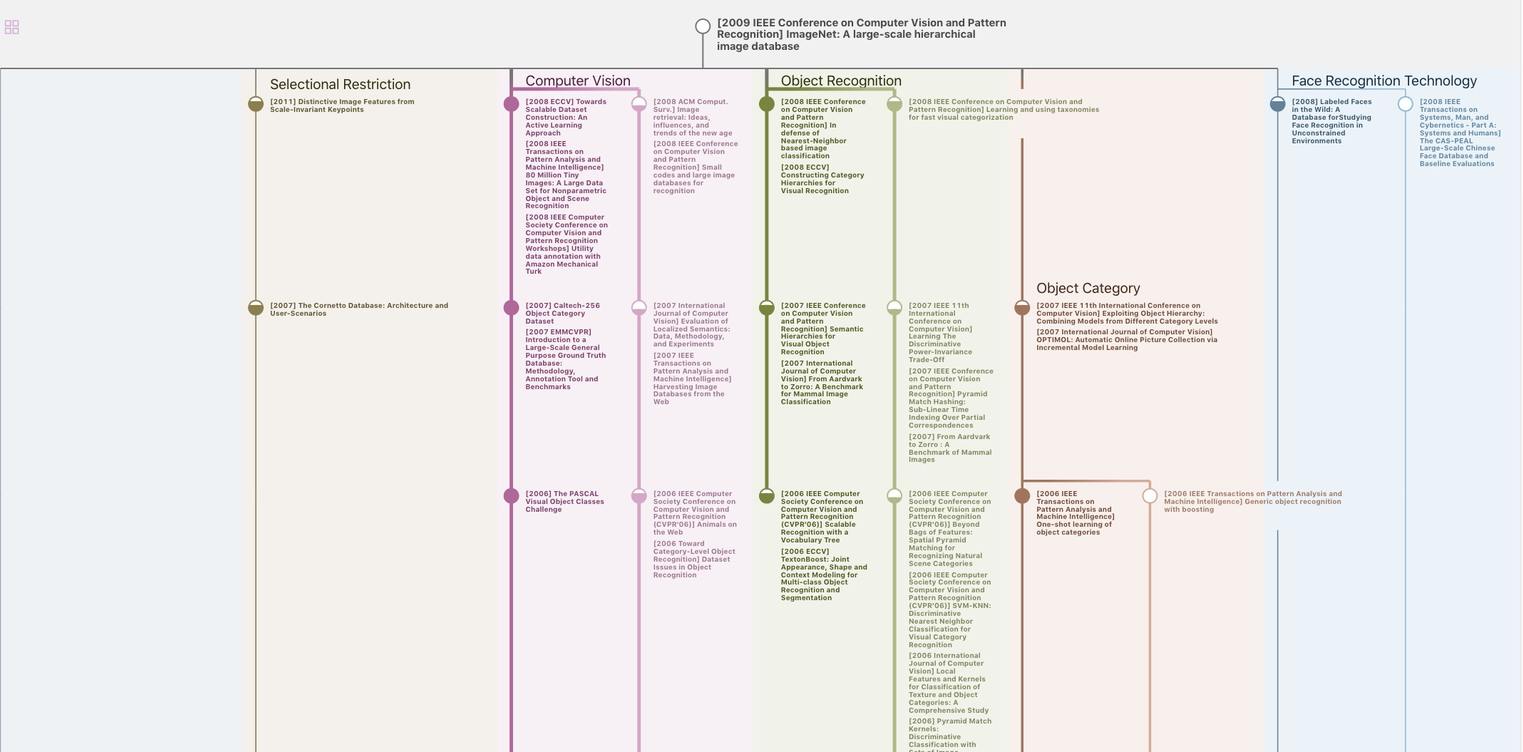
生成溯源树,研究论文发展脉络
Chat Paper
正在生成论文摘要