Cubature-based Uncertainty Estimation for Nonlinear Regression Models
arxiv(2024)
摘要
Calibrating model parameters to measured data by minimizing loss functions is an important step in obtaining realistic predictions from model-based approaches, e.g., for process optimization. This is applicable to both knowledge-driven and data-driven model setups. Due to measurement errors, the calibrated model parameters also carry uncertainty. In this contribution, we use cubature formulas based on sparse grids to calculate the variance of the regression results. The number of cubature points is close to the theoretical minimum required for a given level of exactness. We present exact benchmark results, which we also compare to other cubatures. This scheme is then applied to estimate the prediction uncertainty of the NRTL model, calibrated to observations from different experimental designs.
更多查看译文
AI 理解论文
溯源树
样例
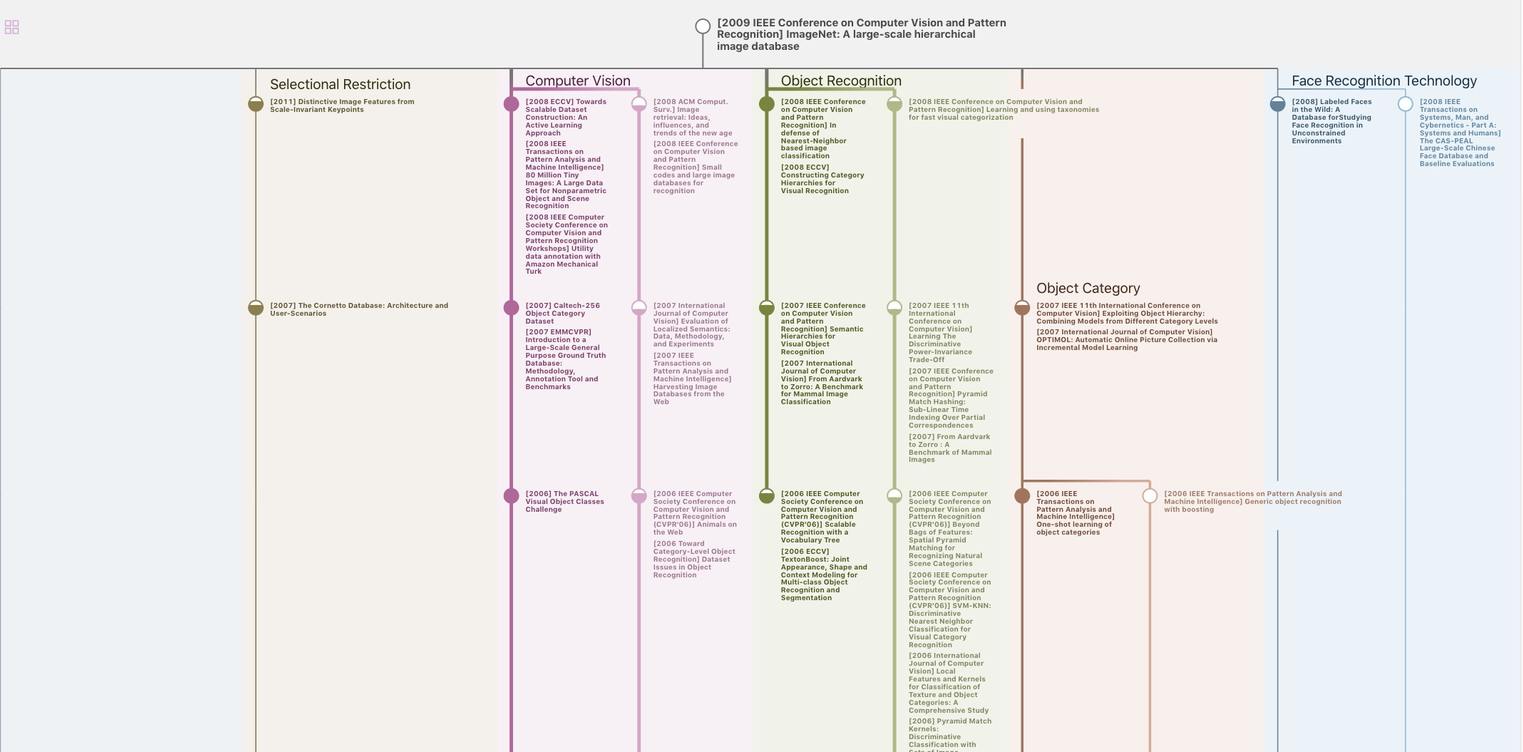
生成溯源树,研究论文发展脉络
Chat Paper
正在生成论文摘要