Predictive Gene Signature for Trabectedin Efficacy in Advanced Soft‐tissue Sarcoma: A Spanish Group for Research in Sarcoma (GEIS) Study.
Journal of clinical oncology(2024)
Abstract
11543 Background: Predictive biomarkers of trabectedin represent a clinical unmet need to support the rational selection of this drug in the advanced soft‐tissue sarcomas (STS) setting. A few potential predictive biomarkers of trabectedin had been described in previous studies, including ERCC1, BRCA1, and HMGA1, among others; however, they are based mostly on the analysis of single selected molecular factors. We presented here a predictive transcriptomic-based signature for trabectedin efficacy in STS. Methods: A total of 140 patients with advanced STS (at diagnosis or at any time from then on), with paraffin tumor block available, with at least 2 lines of treatment in advanced disease (one of them trabectedin) were included in this study. Gene expression was evaluated by direct transcriptomics, using HTG EdgeSeq Oncology Biomarkers Panel (HTG Molecular Diagnostics, Inc.; Tucson, AZ, USA). Raw counts were normalized to transcripts per million (TPM). Univariate Cox regression analysis was performed to find genes significantly associated with PFS and only those genes with significantly different curves were further retained (P < 0.05). These remaining genes were used as input to build a gene expression signature, using a multivariate Cox regression applying a Lasso penalty. Risk scores were calculated by multiplying the expression of every gene with its corresponding Cox regression coefficient. Results: A series of 140 patients was studied, with a median age of 51, 54% males, and a median follow-up from diagnostics of 45 months. The most frequent subtypes were L-sarcomas (54%). A twenty-nine-gene predictive signature of trabectedin efficacy was built for advanced STS treatment. This signature included 13 and 16 genes associated with resistance or sensitivity to trabectedin, respectively. Genes associated with low efficacy of trabectedin included HMGA1, NES, FANCA, and FANCB, among others, whereas the high expression of genes such as NTRK2 or CDKN2Awere associated with higher activity of trabectedin. Patients in the high‐risk gene signature group showed a significantly worse PFS compared with patients in the low‐risk group: [2.0 months (95% CI 1.7-2.2) vs. 8.2 months (95% CI 5.1-11.3), p<0.001]. Conclusions: Our study identified a new 29-gene-based signature that significantly predicts trabectedin efficacy in STS patients. Prospective validation of this predictive gene signature is warranted.
MoreTranslated text
AI Read Science
Must-Reading Tree
Example
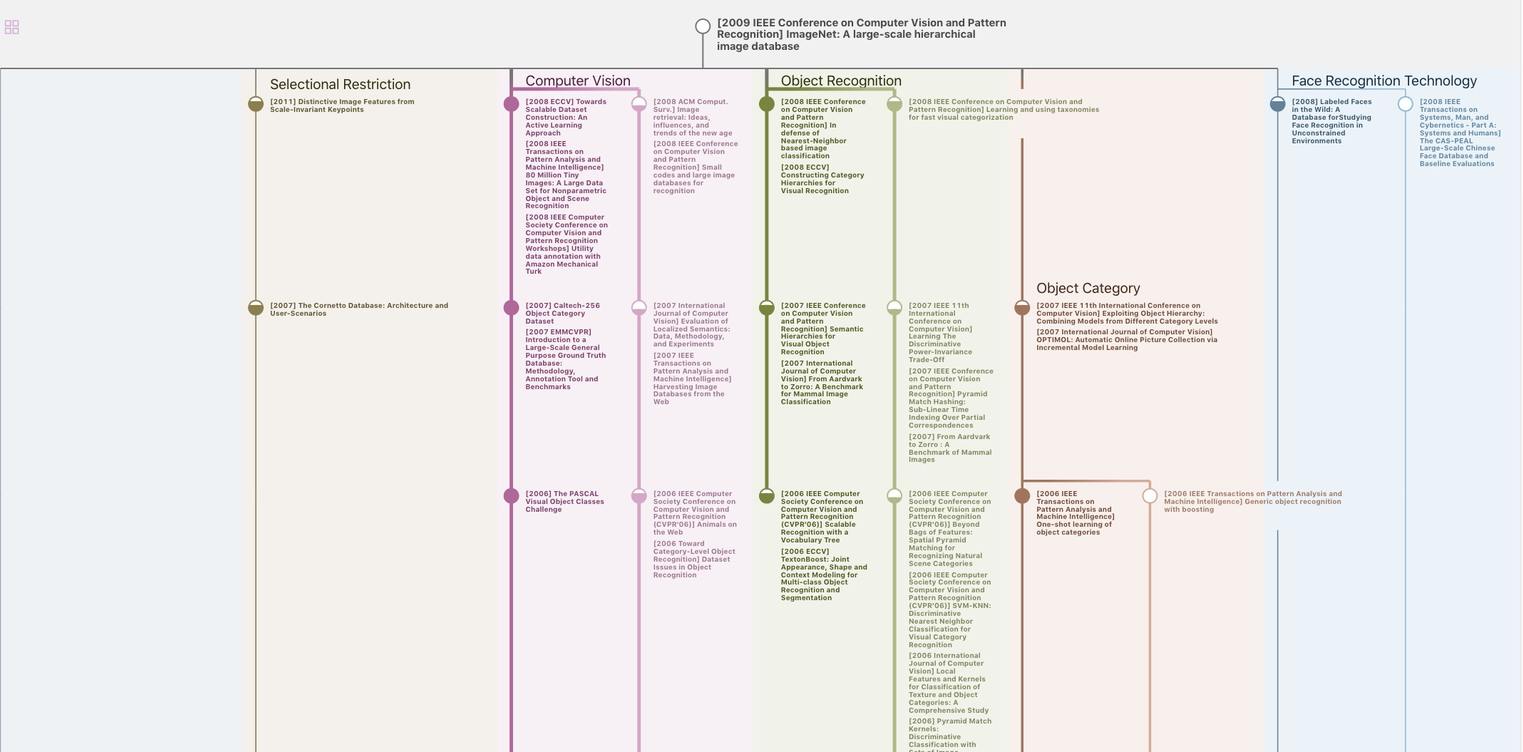
Generate MRT to find the research sequence of this paper
Chat Paper
Summary is being generated by the instructions you defined