A Deep Learning Approach to Prediction of Blood Group Antigens from Genomic Data
TRANSFUSION(2024)
摘要
Background: Deep learning methods are revolutionizing natural science. In this study, we aim to apply such techniques to develop blood type prediction models based on cheap to analyze and easily scalable screening array genotyping platforms. Methods: Combining existing blood types from blood banks and imputed screening array genotypes for similar to 111,000 Danish and 1168 Finnish blood donors, we used deep learning techniques to train and validate blood type prediction models for 36 antigens in 15 blood group systems. To account for missing genotypes a denoising autoencoder initial step was utilized, followed by a convolutional neural network blood type classifier. Results: Two thirds of the trained blood type prediction models demonstrated an F1-accuracy above 99%. Models for antigens with low or high frequencies like, for example, C-w, low training cohorts like, for example, Co-b, or very complicated genetic underpinning like, for example, RhD, proved to be more challenging for high accuracy (>99%) DL modeling. However, in the Danish cohort only 4 out of 36 models (Co-b, C-w, D-weak, Kp(a)) failed to achieve a prediction F1-accuracy above 97%. This high predictive performance was replicated in the Finnish cohort. Discussion: High accuracy in a variety of blood groups proves viability of deep learning-based blood type prediction using array chip genotypes, even in blood groups with nontrivial genetic underpinnings. These techniques are suitable for aiding in identifying blood donors with rare blood types by greatly narrowing down the potential pool of candidate donors before clinical grade confirmation.
更多查看译文
关键词
AI,blood antigen,blood types,convolutional neural network,deep learning,denoising autoencoder,genetic prediction,Illumina GSA
AI 理解论文
溯源树
样例
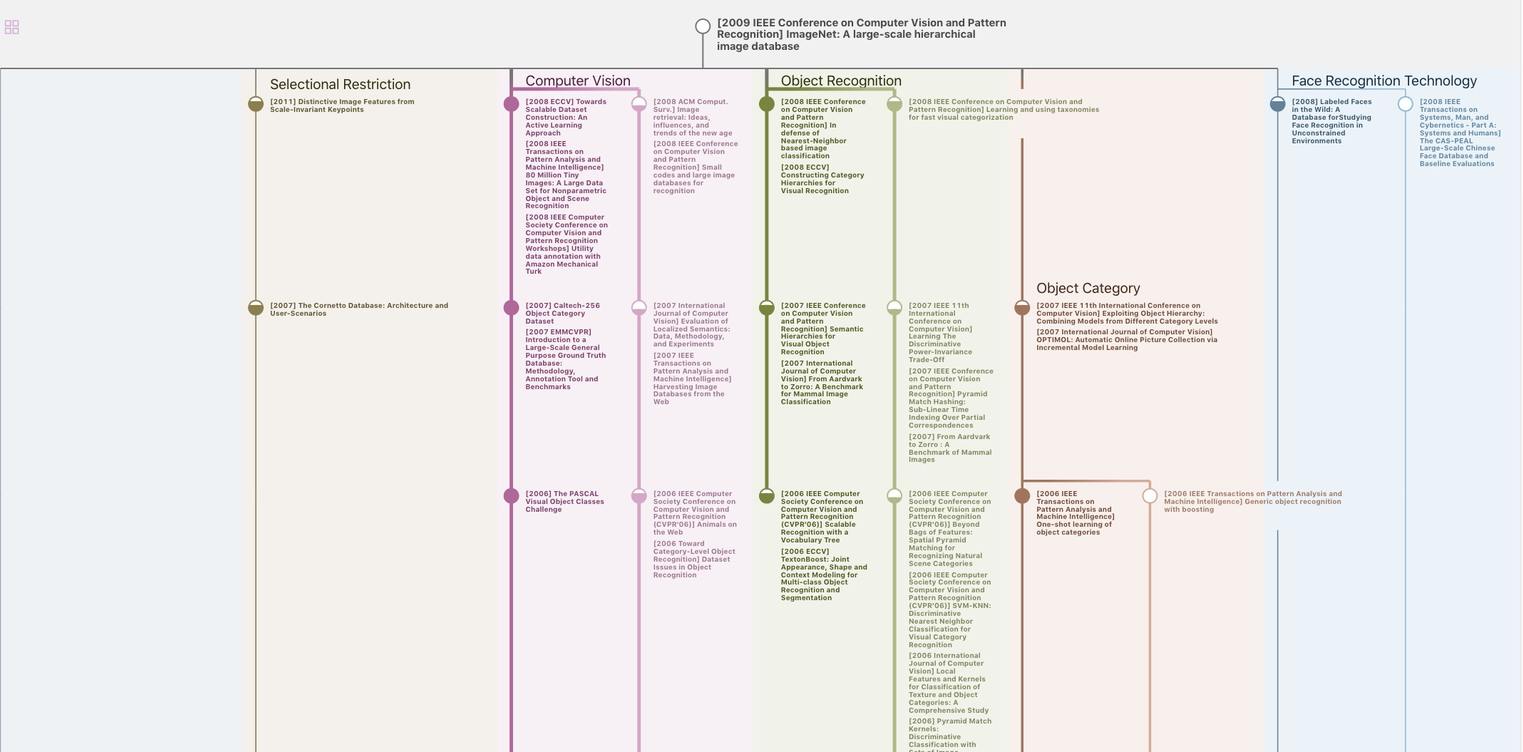
生成溯源树,研究论文发展脉络
Chat Paper
正在生成论文摘要