Learning Crowd Scale and Distribution for Weakly Supervised Crowd Counting and Localization
IEEE Transactions on Circuits and Systems for Video Technology(2024)
摘要
The count supervision used in weakly-supervised crowd counting is derived from the number of point annotations, which means that the labeling cost is not effectively reduced. Moreover, due to the lack of spatial information about the pedestrians during training, previous works struggle to accurately learn the positions of individuals. To address these challenges, we propose a crowd counting and localization method based on scene-specific synthetic data for surveillance scenarios, which can accurately predict the number and location of person without any manually labeled point-wise or count-wise annotations. Our method dynamically adjust scene-specific synthetic data to minimize domain differences from surveillance scenes by learning the crowd scale and distribution. Specifically, based on realistic synthetic data, the models learn precise location and scale information, which can then regenerate new synthetic data with a more reasonable pedestrian distribution and scale and generate high-quality pseudo point-wise annotations. Subsequently, the counter is trained using our proposed robust soft-weighted loss function, under the joint supervision of auto-generated point-wise annotations on synthetic data and pseudo point-wise annotations on real data in an end-to-end manner. Our proposed loss function, based on the designed weighted optimal transport, effectively mitigates noise in pseudo point-wise labels and is not only insensitive to hyperparemeters but also exhibits superior generalization ability on real data. We conduct comprehensive experiments across multiple scene-specific datasets, demonstrating our method’s superiority in counting and localization performance over count-supervised, fully-supervised, and state-of-the-art domain adaption algorithms.
更多查看译文
关键词
Crowd counting,Crowd localization,Weakly-supervised,Synthetic data
AI 理解论文
溯源树
样例
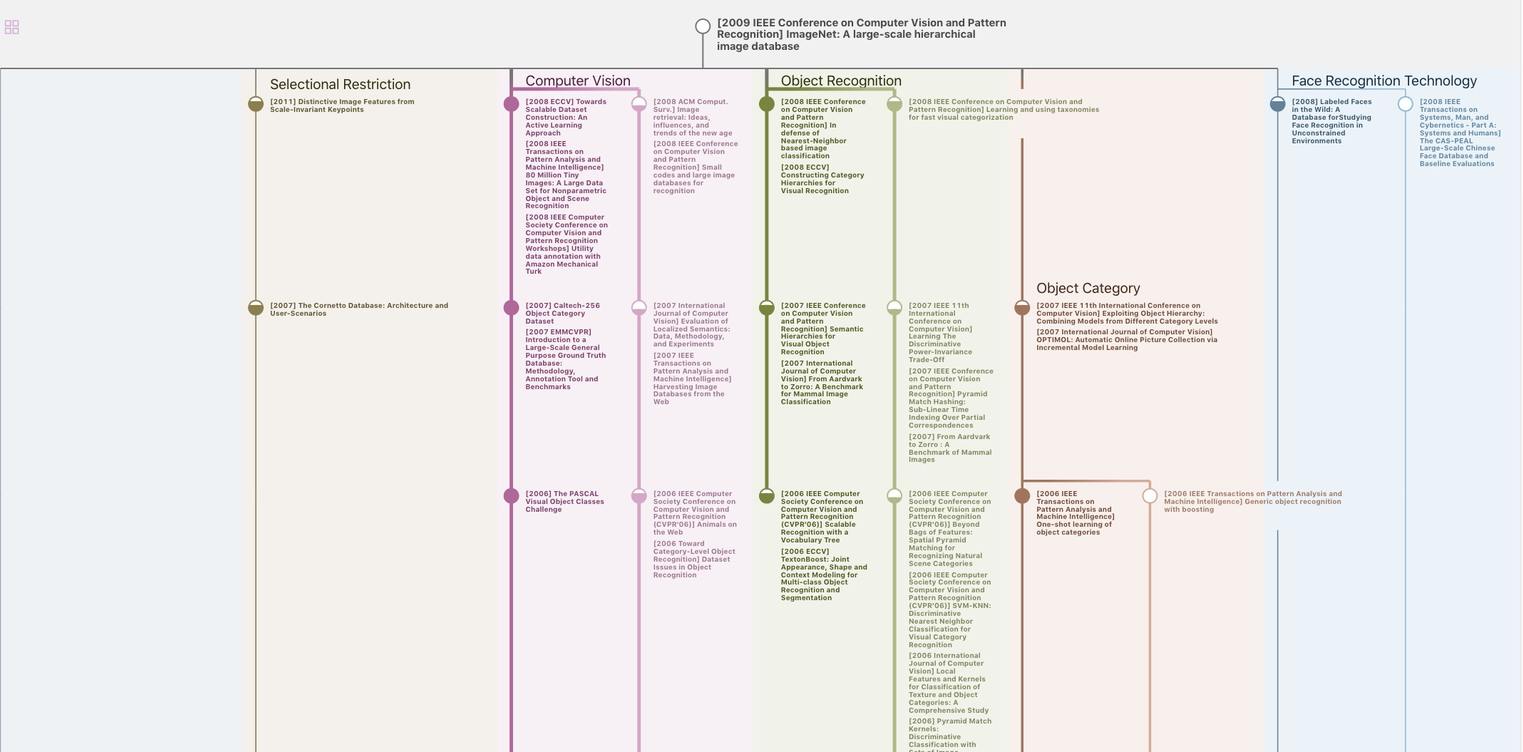
生成溯源树,研究论文发展脉络
Chat Paper
正在生成论文摘要