Sample-to-answer Centrifugal Microfluidic Droplet PCR Platform for Quantitation of Viral Load
Lab on a chip(2024)
摘要
Droplet digital polymerase chain reaction (ddPCR) stands out as a highly sensitive diagnostic technique that is gaining traction in infectious disease diagnostics due to its ability to quantitate very low numbers of viral gene copies. By partitioning the sample into thousands of droplets, ddPCR enables precise and absolute quantification without relying on a standard curve. However, current ddPCR systems often exhibit relatively low levels of integration, and the analytical process remains dependent on elaborate workflows for up-front sample preparation. Here, we introduce a fully-integrated system seamlessly combining viral lysis, RNA extraction, emulsification, reverse transcription (RT) ddPCR, and fluorescence readout in a sample-to-answer format. The system comprises a disposable microfluidic cartridge housing buffers and reagents required for the assay, and a centrifugal platform that allows for pneumatic actuation of liquids during rotation, enabling automation of the workflow. Highly monodisperse droplets (∼50 μm in diameter) are produced using centrifugal step emulsification and automatically transferred to an integrated heating module for target amplification. The platform is equipped with a miniature fluorescence imaging system enabling on-chip read-out of droplets after RT-ddPCR. We demonstrate sample-to-answer detection of SARS-CoV-2 N and E genes, along with RNase P endogenous reference, using hydrolysis probes and multiplexed amplification within single droplets for concentrations as low as 0.1 copy per μL. We also tested 14 nasopharyngeal swab specimens from patients and were able to distinguish positive and negative SARS-CoV-2 samples with 100% accuracy, surpassing results obtained by conventional real-time amplification.
更多查看译文
AI 理解论文
溯源树
样例
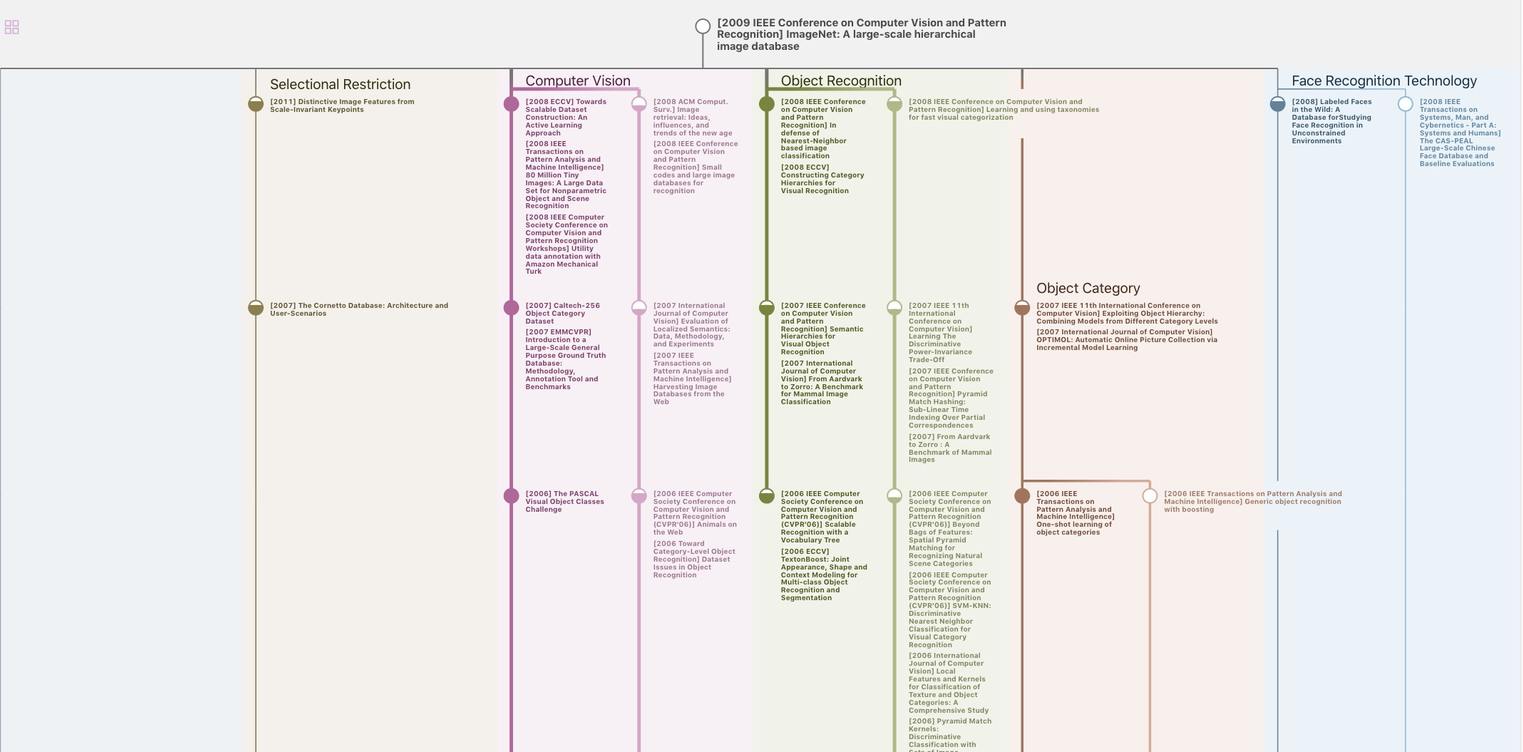
生成溯源树,研究论文发展脉络
Chat Paper
正在生成论文摘要