Contrastive Transformer Network for Track Segment Association with Two-Stage Online Method
Remote Sensing(2024)
摘要
Interrupted and multi-source track segment association (TSA) are two key challenges in target trajectory research within radar data processing. Traditional methods often rely on simplistic assumptions about target motion and statistical techniques for track association, leading to problems such as unrealistic assumptions, susceptibility to noise, and suboptimal performance limits. This study proposes a unified framework to address the challenges of associating interrupted and multi-source track segments by measuring trajectory similarity. We present TSA-cTFER, a novel network utilizing contrastive learning and TransFormer Encoder to accurately assess trajectory similarity through learned Representations by computing distances between high-dimensional feature vectors. Additionally, we tackle dynamic association scenarios with a two-stage online algorithm designed to manage tracks that appear or disappear at any time. This algorithm categorizes track pairs into easy and hard groups, employing tailored association strategies to achieve precise and robust associations in dynamic environments. Experimental results on real-world datasets demonstrate that our proposed TSA-cTFER network with the two-stage online algorithm outperforms existing methods, achieving 94.59% accuracy in interrupted track segment association tasks and 94.83% in multi-source track segment association tasks.
更多查看译文
关键词
radar data processing,track segment association,contrastive learning,transformer encoder,online association
AI 理解论文
溯源树
样例
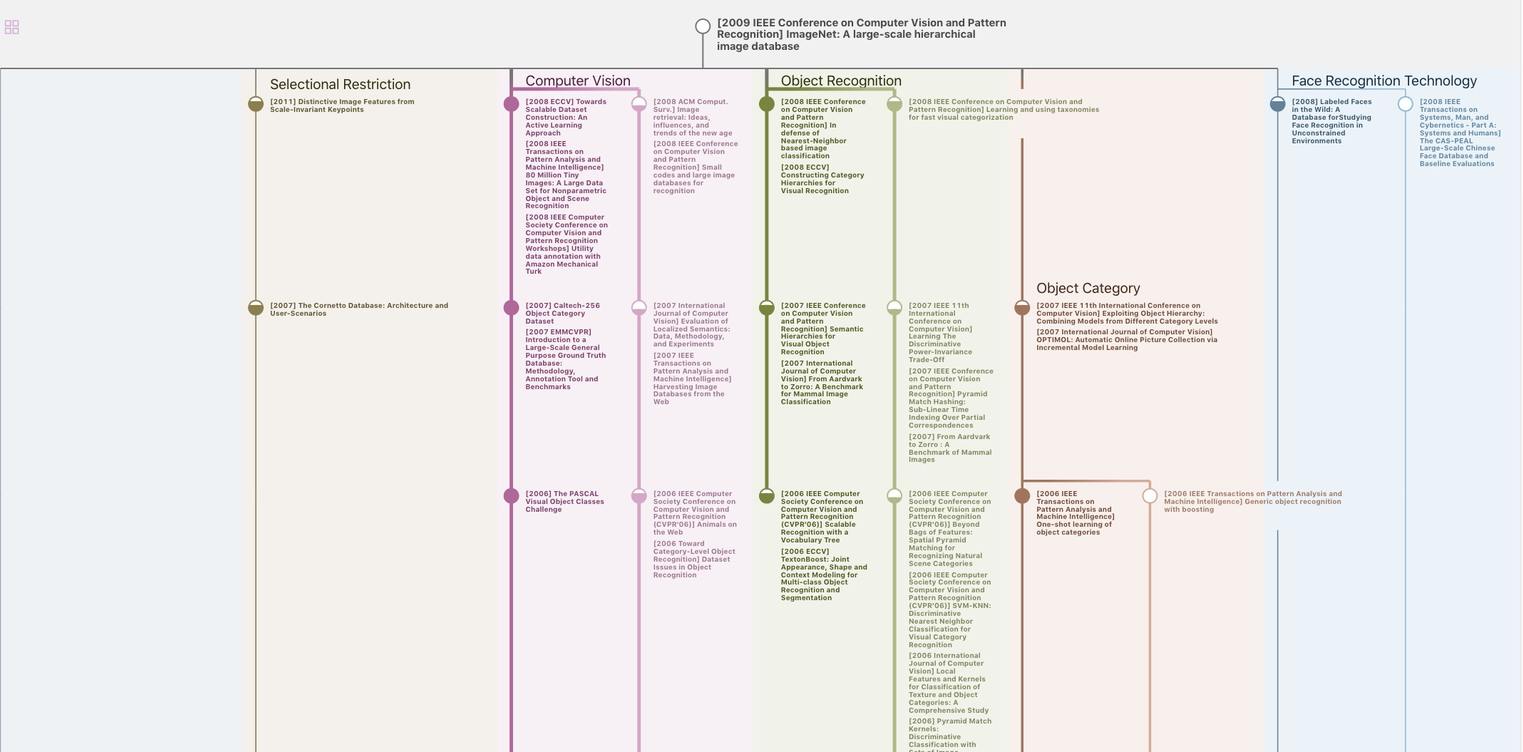
生成溯源树,研究论文发展脉络
Chat Paper
正在生成论文摘要