Assessing the Affective Quality of Soundscape for Individuals: Using Third-Party Assessment Combined with an Artificial Intelligence (TPA-AI) Model
The Science of the total environment(2024)
摘要
When investigating the relationship between the acoustic environment and human wellbeing, there is a potential problem resulting from data source self-correlation. To address this data source self-correlation problem, we proposed a third-party assessment combined with an artificial intelligence (TPA-AI) model. The TPA-AI utilized acoustic spectrograms to assess the soundscape's affective quality. First, we collected data on public perceptions of urban sounds (i.e., inviting 100 volunteers to label the affective quality of 7051 10-s audios on a polar scale from annoying to pleasant). Second, we converted the labeled audios to acoustic spectrograms and used deep learning methods to train the TPA-AI model, achieving a 92.88 % predictive accuracy for binary classification. Third, geographic ecological momentary assessment (GEMA) was used to log momentary audios from 180 participants in their daily life context, and we employed the well-trained TPA-AI model to predict the affective quality of these momentary audios. Lastly, we compared the explanatory power of the three methods (i.e., sound level meters, sound questionnaires, and the TPA-AI model) when estimating the relationship between momentary stress level and the acoustic environment. Our results indicate that the TPA-AI's explanatory power outperformed the sound level meter, while using a sound questionnaire might overestimate the effect of the acoustic environment on momentary stress and underestimate other confounders.
更多查看译文
关键词
Soundscape,Affective quality,Momentary stress,Data source self-correlation problem,Third-party assessment,Deep learning
AI 理解论文
溯源树
样例
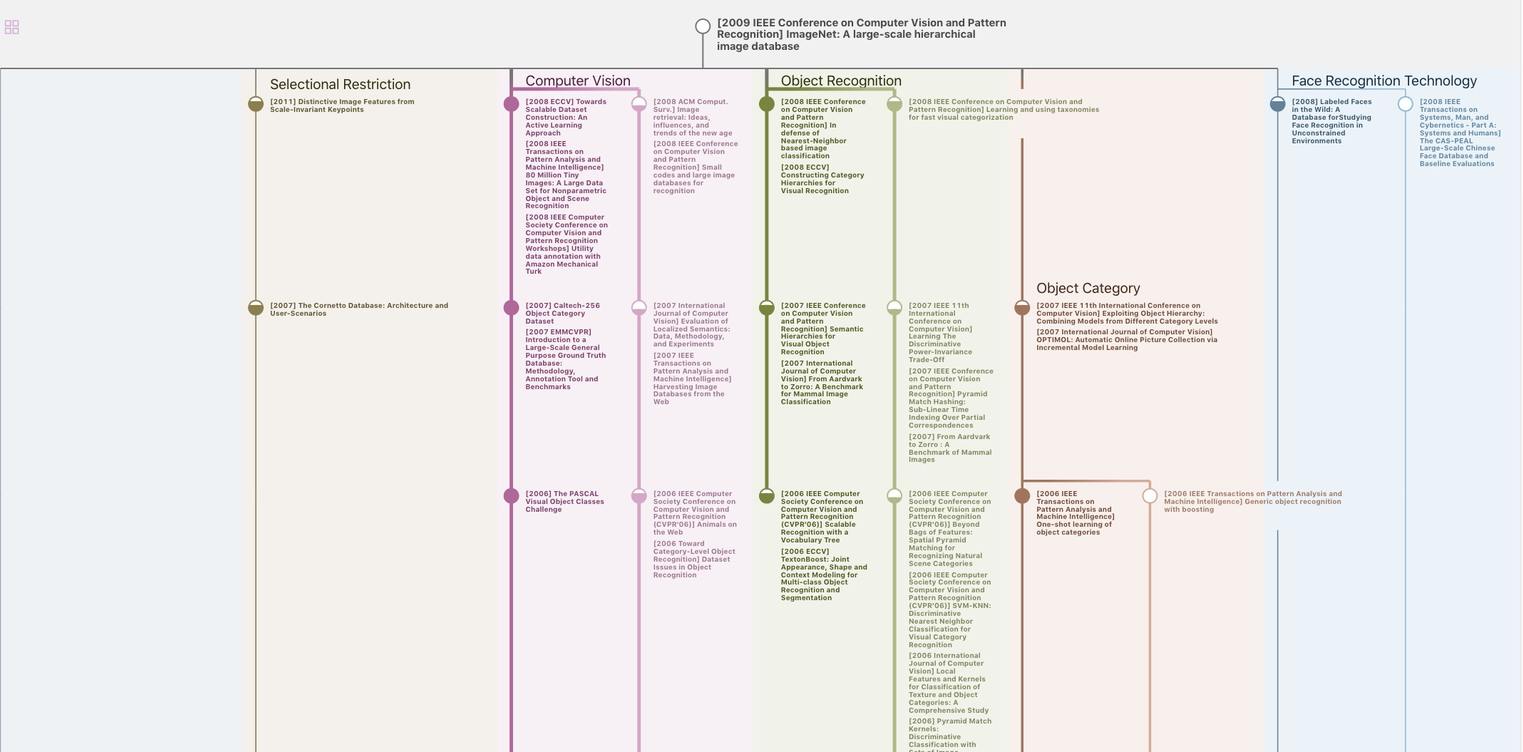
生成溯源树,研究论文发展脉络
Chat Paper
正在生成论文摘要