An Accurate and Efficient Forecast Framework for Fine PM2.5 Maps Using Spatiotemporal Recurrent Neural Networks
Journal of Cleaner Production(2024)
摘要
Commonly used numerical prediction models for PM2.5 maps suffer from low accuracy and high computation cost, which cannot meet the requirements for fine-scale air pollution control. In this study, we propose a framework based on the spatiotemporal recurrent neural network (PredRNN) to efficiently generate accurate 3-hour and 6-km PM2.5 maps with a lead time of 5 days. In this framework, two PredRNN networks are initially utilized to forecast PM2.5 concentration at ground monitoring sites and the spatial distribution of aerosol optical depth (AOD) by assimilating the output of numerical prediction model. Subsequently, the 3-hour and 6-km PM2.5 forecasted maps with a lead time of 5 days can be inferred by establishing the regression links between the forecasted results of PM2.5 concentration at ground sites and AOD maps. We evaluate the proposed framework in the Beijing-Tianjin-Hebei urban agglomeration region during 2017 to 2020. Compared with the numerical prediction products of the Copernicus Atmosphere Monitoring Service, the proposed framework achieves higher accuracy, with R2 of 0.83 at the forecast base time and 0.70 at the fifth day. The spatial information richness is also enhanced by approximately 15.67% according to the information entropy metrics. Notably, the proposed framework only requires one minute for forecasting 5-days PM2.5 maps. These results demonstrate that our framework can efficiently generate accurate and fine PM2.5 maps with a lead time of 5 days.
更多查看译文
关键词
PM2.5 forecast,high spatial information richness,chemical transport model,deep learning,efficiency,accurate
AI 理解论文
溯源树
样例
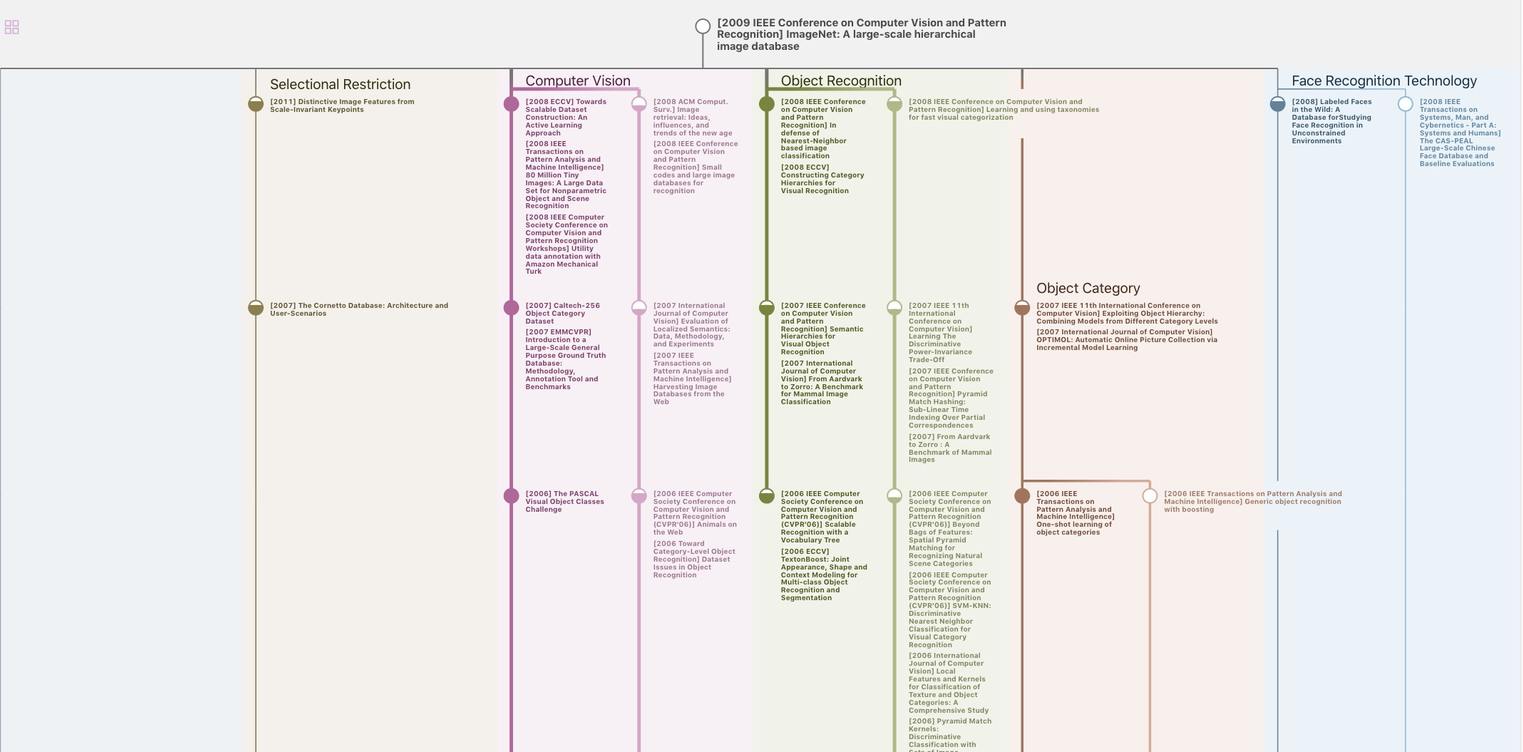
生成溯源树,研究论文发展脉络
Chat Paper
正在生成论文摘要