A 4.69-TOPS/W Training, 2.34- $\mu$ J/Image Inference On-Chip Training Accelerator with Inference-Compatible Backpropagation and Design Space Exploration in 28-Nm CMOS
IEEE Journal of Solid-State Circuits(2024)
摘要
On-chip training (OCT) accelerators improve personalized recognition accuracy while ensuring user privacy. However, previous OCT accelerators often required significant additional hardware costs to support retraining, even though inference is the primary use case. We propose an inference-pattern-compatible backpropagation (BP) circuit, which enables the training process to reuse inference hardware. To achieve high energy efficiency, we utilize three hardware-friendly optimization methods that significantly reduce redundant computation and external memory access (EMA). Additionally, we propose a design space exploration (DSE) to search for the optimal hardware configurations, which improves system performance while reducing the design time cost. Fabricated in a 28-nm CMOS process, this single-core OCT chip is able to train all the layers of a neural network (NN), achieving a peak training efficiency of 4.69 Tera operations per second per watt (TOPS/W). It also achieves the lowest inference energy of 2.34 $\mu$ J/inf/image under a core voltage of 0.48 V and 40 MHz.
更多查看译文
关键词
Backpropagation (BP) circuit,design space exploration (DSE),energy efficiency,neural network (NN),on-chip training (OCT),transfer learning
AI 理解论文
溯源树
样例
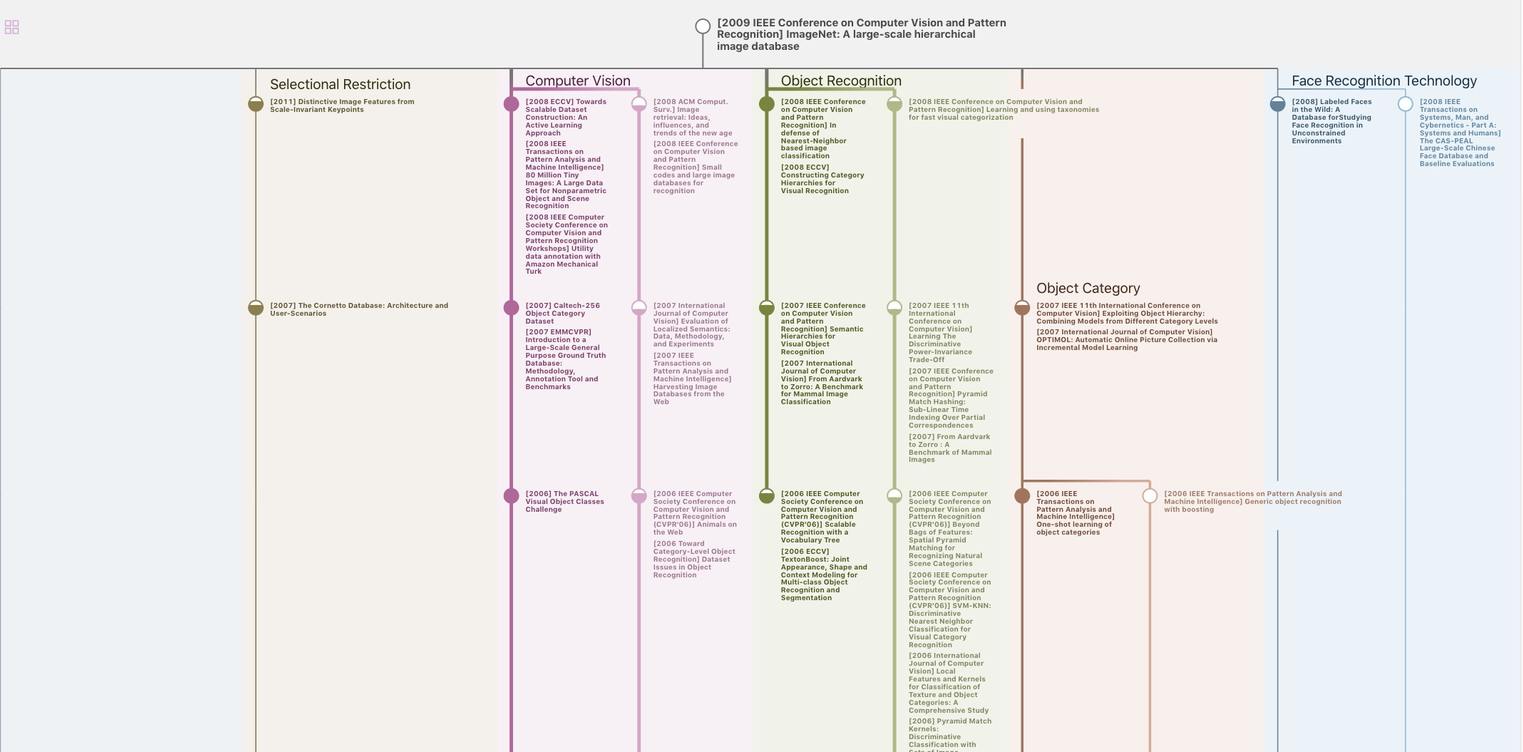
生成溯源树,研究论文发展脉络
Chat Paper
正在生成论文摘要