A Knowledge Distillation-Driven Lightweight CNN Model for Detecting Malicious Encrypted Network Traffic
2024 International Joint Conference on Neural Networks (IJCNN)(2024)
摘要
In the realm of cybersecurity, efficiently and precisely identifying and mitigating potential threats from malicious encrypted traffic is crucial. As deep learning evolves, methods relying on Convolutional Neural Networks (CNNs) to convert traffic payloads into visual representations for analysis have become popular. Although this technique effectively discerns malicious encrypted traffic, it faces inherent limitations, such as difficulties in deployment due to extensive network scales and high computational demands, especially in edge computing environments. To address these challenges, we propose a model for maliciously encrypted traffic detection, named LightMETD. The model consists of two stages. In the first stage, we utilize Random Forest (RF) with a feature selection algorithm to quickly identify easily distinguishable traffic patterns. In the second stage, the filtered traffic data is converted into grayscale images and classified using an innovative lightweight MobileNetV3-S architecture. Our LightMETD model addresses the problem of large volume and high computational requirements of CNN models. To enhance the effectiveness of the LightMETD, we propose an innovative knowledge distillation method to train LightMETD. This method significantly improves the accuracy of malicious encrypted traffic detection by designing a distillation loss function that enables the model to better capture the intricate relationships between samples. We use a USTC-TFC2016 public traffic dataset and a locally collected dataset to demonstrate the effectiveness of LightMETD. LightMETD achieves an impressive 97% average classification accuracy. LightMETD significantly outperforms other baselines in terms of volume and detection speed, underscoring its viability and superiority in addressing real-world challenges posed by malicious encrypted traffic.
更多查看译文
关键词
Cybersecurity,Convolutional Neural Networks,Knowledge Distillation,Malicious Encrypted Traffic,Lightweight
AI 理解论文
溯源树
样例
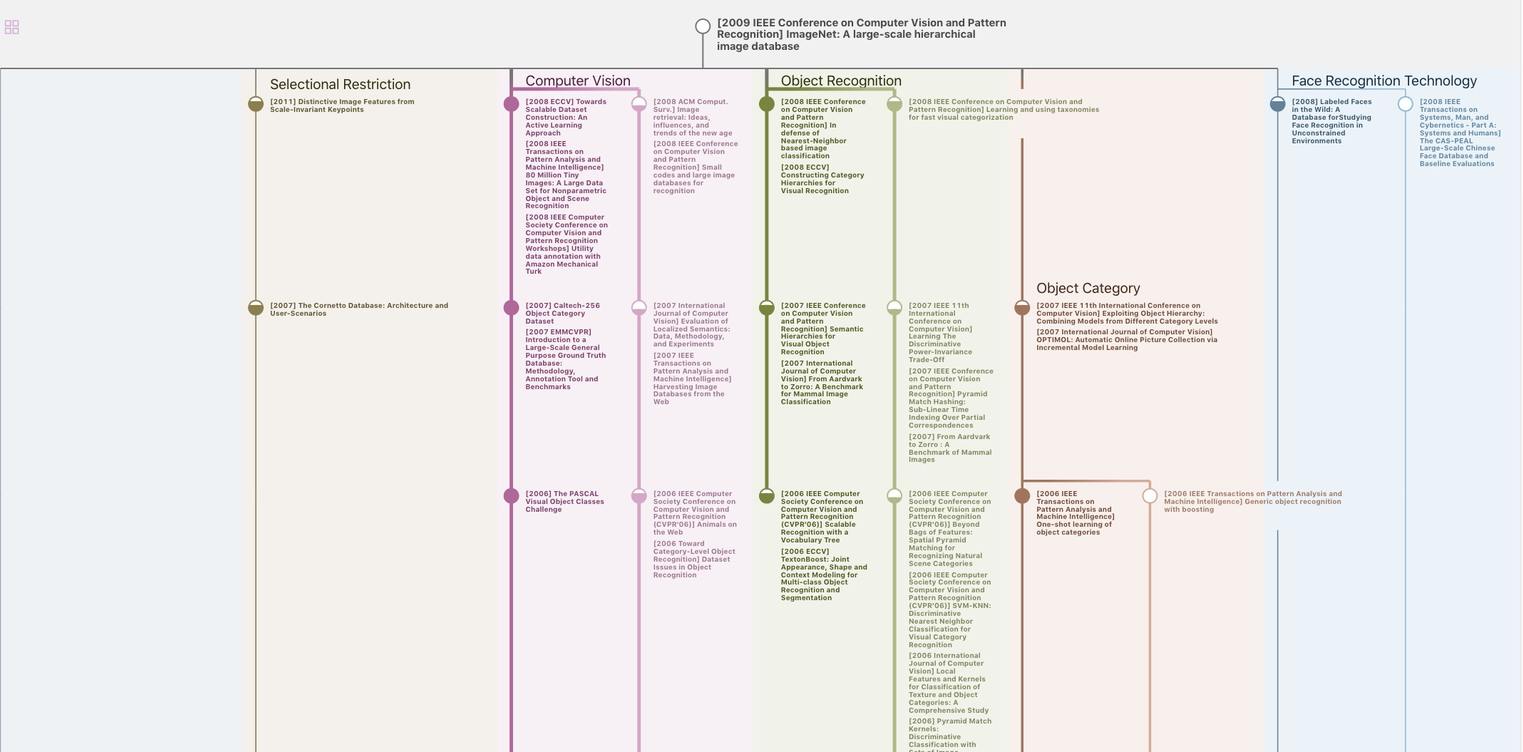
生成溯源树,研究论文发展脉络
Chat Paper
正在生成论文摘要