The Long-Term Memory Transformer with Multimodal Fusion for Radiology Report Generation
2024 International Joint Conference on Neural Networks (IJCNN)(2024)
摘要
Radiology report generation can simulate the diagnostic process of doctors. Automatically generate diagnostic reports has attracted more and more attention from researchers in recent years. However, existing report generation methods based on the encoder-decoder framework mainly choose convolutional neural networks (CNNs) as image feature extractors and transformers as decoder. To address the problems that a single image encoder cannot effectively alleviate the visual-textual cross-modal semantic gap and that traditional transformer cannot capture enough long-term dependencies, which leads to poor report generation quality, in this paper, we propose a framework for radiology report generation that achieves long-term memory transformer with visual-textual cross-fusion. Large vision-and-language pretraining (VLP) models are used to obtain visual and textual representations containing rich multimodal knowledge. A cross-fusion module is used to achieve deep interaction between visual and textual representations, aiming at exploring the subtle interactions between visual and textual representations. Thus complex cross-modal generation capabilities are enhanced. The memory module saves the global and the previous information, which is convenient for the model to integrate the global and the previous information in the decoding process, and better capture the long-term dependency relationships. Experiments on the IU X-Ray dataset and MIMIC-CXR dataset show that our approach significantly improves the accuracy of report generation, achieving advanced results on several evaluation metrics and demonstrating superior performance.
更多查看译文
关键词
radiology report generation,multimodal cross-fusion,vision-language pretraining,long-term memory transformer
AI 理解论文
溯源树
样例
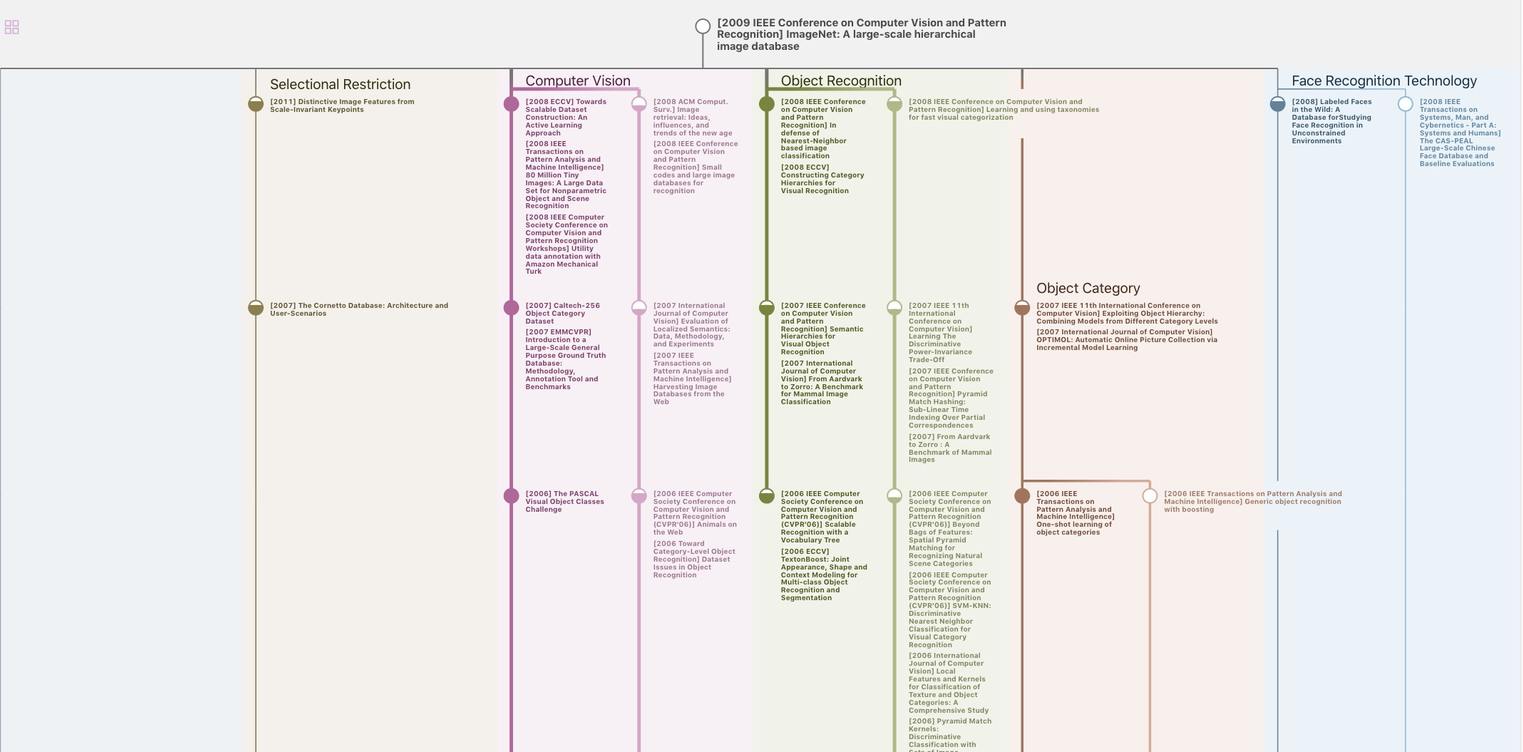
生成溯源树,研究论文发展脉络
Chat Paper
正在生成论文摘要