HGG-47. SPATIAL SINGLE-CELL PROTEOMIC ANALYSIS MAPS THE DISTINCT PEDIATRIC-TYPE DIFFUSE HIGH-GRADE GLIOMA TUMOR ENTITIES AND DEFINES THEIR TUMOR AND IMMUNE MICROENVIRONMENTS
NEURO-ONCOLOGY(2024)
Bambino Gesu Childrens Hosp IRCCS | Univ Cambridge | Bambino Gesù Children's Hospital
Abstract
Paediatric-type diffuse high-grade gliomas (pDHGG) are a group of molecularly and clinically defined tumor entities of the central nervous system. The investigation of the spatial organization of pDHGG tissues may provide new insights to decipher their heterogeneous nature and identify vulnerabilities in their aggressive behaviour. Here we exploit Imaging Mass Cytometry (IMC), a spatial biology approach enabling multiplex tissue phenotyping at single-cell resolution. We used 16 total formalin-fixed paraffin-embedded (FFPE) tissues from 4 patients including 1 Diffuse hemispheric glioma H3G34-mutant (DHG-H3G34), 1 Diffuse pediatric-type high-grade glioma H3-wildtype and IDH-wildtype (DHGG-WT) and 2 Diffuse midline glioma H3K27-altered (DMG-H3K27, 1 H3.3K27M and 1 H3.1K27M). Classical pathology assessment and DNA methylation profile were also performed. For IMC staining, 2 metal-tag custom-designed antibody panels (tumour and immune) of 31 antibodies were used. The panels include antibodies for differentiated and stem-cell markers, extracellular matrices, immune markers and H3K27M and H3.3G34R variants. An IMC analysis pipeline was developed: images are extracted in tiff format from the proprietary MCD files produced by Hyperion instrument, filtered to remove hot pixels via top-hat and OpenCV in Python, segmented using steinbock and analysed using the IMC Data Analysis toolbox in R, using docker. A total of 209 mm2 of tissue were analysed. IMC analysis showed that DHG-H3G34 displays a higher heterogeneity in terms of cell phenotypes compared to the other tumor entities. Moreover, immune infiltrate of T, B cells, NK and monocytes was observed in the DHG-H3G34 and DHGG-WT tissues, while the DMG-H3K27 had low/no immune infiltrate with the H3.3K27M showing higher PDL-1 expression. Analysis of longitudinal and multi-region samples highlighted trajectories of tumor evolution and identification of extracellular matrix niches in tumor core versus the infiltrative front. Our study provides novel insights into pDHGG complex biology by investigating their tissue architecture, spatial organization and cell-cell interactions.
MoreTranslated text
PDF
View via Publisher
AI Read Science
AI Summary
AI Summary is the key point extracted automatically understanding the full text of the paper, including the background, methods, results, conclusions, icons and other key content, so that you can get the outline of the paper at a glance.
Example
Background
Key content
Introduction
Methods
Results
Related work
Fund
Key content
- Pretraining has recently greatly promoted the development of natural language processing (NLP)
- We show that M6 outperforms the baselines in multimodal downstream tasks, and the large M6 with 10 parameters can reach a better performance
- We propose a method called M6 that is able to process information of multiple modalities and perform both single-modal and cross-modal understanding and generation
- The model is scaled to large model with 10 billion parameters with sophisticated deployment, and the 10 -parameter M6-large is the largest pretrained model in Chinese
- Experimental results show that our proposed M6 outperforms the baseline in a number of downstream tasks concerning both single modality and multiple modalities We will continue the pretraining of extremely large models by increasing data to explore the limit of its performance
Try using models to generate summary,it takes about 60s
Must-Reading Tree
Example
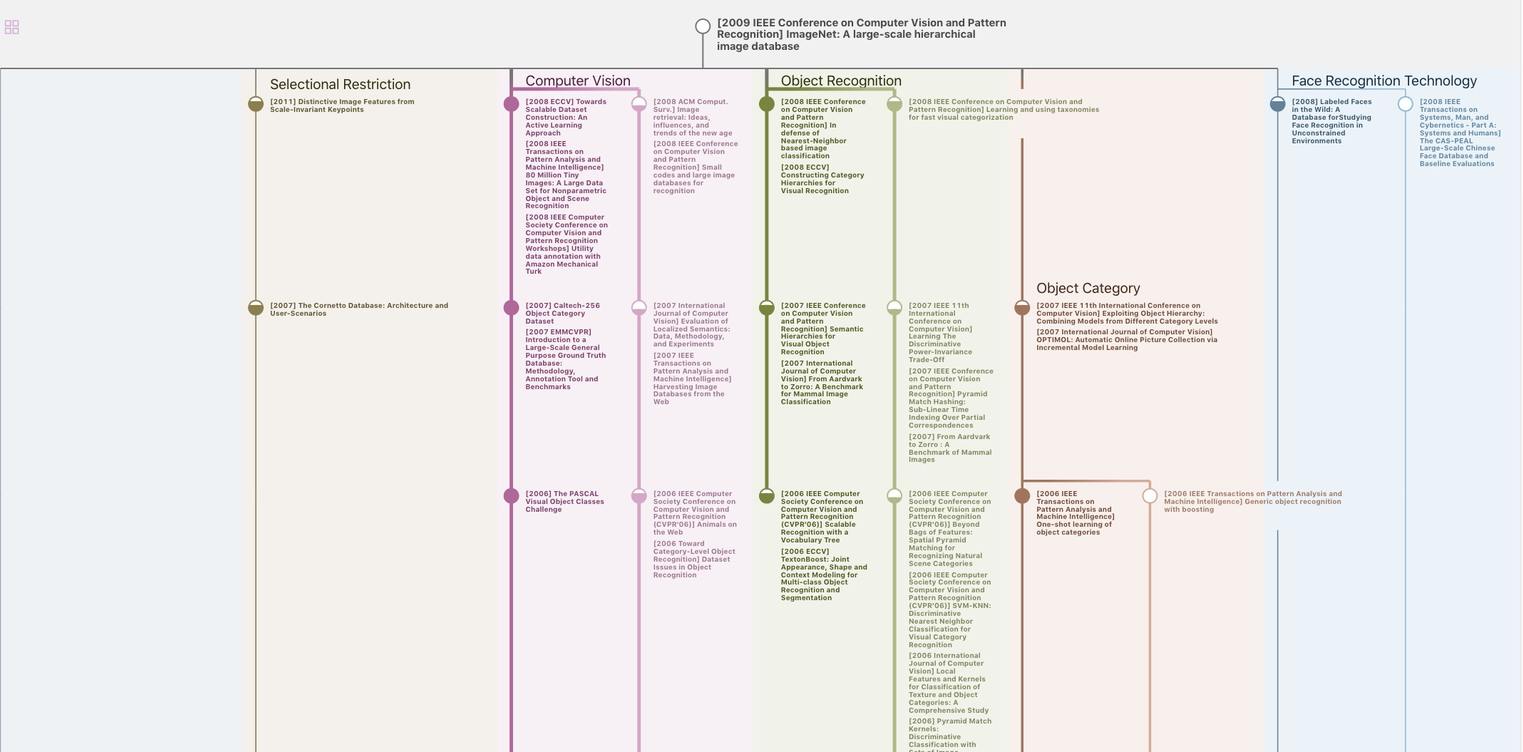
Generate MRT to find the research sequence of this paper
Data Disclaimer
The page data are from open Internet sources, cooperative publishers and automatic analysis results through AI technology. We do not make any commitments and guarantees for the validity, accuracy, correctness, reliability, completeness and timeliness of the page data. If you have any questions, please contact us by email: report@aminer.cn
Chat Paper
去 AI 文献库 对话