1997-LB: Curation of an AI-Ready Dataset Using Domain-Informed Features Derived from Type 1 Diabetes TrialNet Studies
Diabetes(2024)
Abstract
Introduction: Machine learning and artificial intelligence (ML/AI) will increasingly have pivotal roles in advancing scientific discoveries related to diabetes. The NIDDK Central Repository (NIDDK-CR) hosted a “Data Centric Challenge” (DCC) between December 2023 and February 2024 to enhance the potential for using its data resources in innovative ML/AI research that aligns with the FAIR (Findable, Accessible, Interoperable, Reusable) principles. Objective: As DCC participants, we describe our experience transforming data from multiple Type 1 Diabetes (T1D) TrialNet (TN) studies into a single AI-ready dataset for ML/AI applications. Methods: For its intermediate/advanced Challenge, the NIDDK-CR provided fully deidentified data from four studies: TN01 (TN participant screening and monitoring), TN16 (long-term TN participant follow-up), TN19 (immunotherapy in new-onset T1D), and TN20 (antigen-specific immunotherapy). We first generated a single “raw” dataset comprising all data from the four studies by joining on participant ID. We then transformed structured data from TN01 and TN20 to create an AI-ready dataset. Results: Our raw dataset contained data for 237,324 TN participants (TN01 [n=237,048]; TN16 [n=561]; TN19 [n=119]; TN20 [n=115]). Since few individuals participated in ≥3 of these studies, we curated an AI-ready dataset comprised of fully harmonized, longitudinal immunologic, genetic, phenotypic, and demographic data - including numerous new, AI-ready data features (e.g., normalized fold change in autoantibody titers and CyTOF tetramers, genetic risk score, T1D stage) - from individuals who completed participation in TN01 and TN20 (n=75). All data handling processes are repeatable and thoroughly documented. Conclusion: Data from multiple TN studies can be transformed into domain-informed, AI-ready data. Future efforts will entail analyses of newly engineered data variables to inform ML modeling efforts for predicting progression to Stage 2 and Stage 3 T1D. Disclosure E.M. Tallon: None. M.R. Shapiro: None. A. Waghmode: None. R. Merritt: None. C. Wasserfall: None. R. Bacher: None. B. Lockee: None. C. Vandervelden: None. K. Panfil: None. W.V. Moore: None. M.A. Atkinson: None. T.M. Brusko: None. M.A. Clements: Research Support; Abbott. Consultant; Glooko, Inc. Research Support; Dexcom, Inc. Funding Emilie Rosebud Diabetes Research Foundation; Orlando Brown Jr.
MoreTranslated text
AI Read Science
Must-Reading Tree
Example
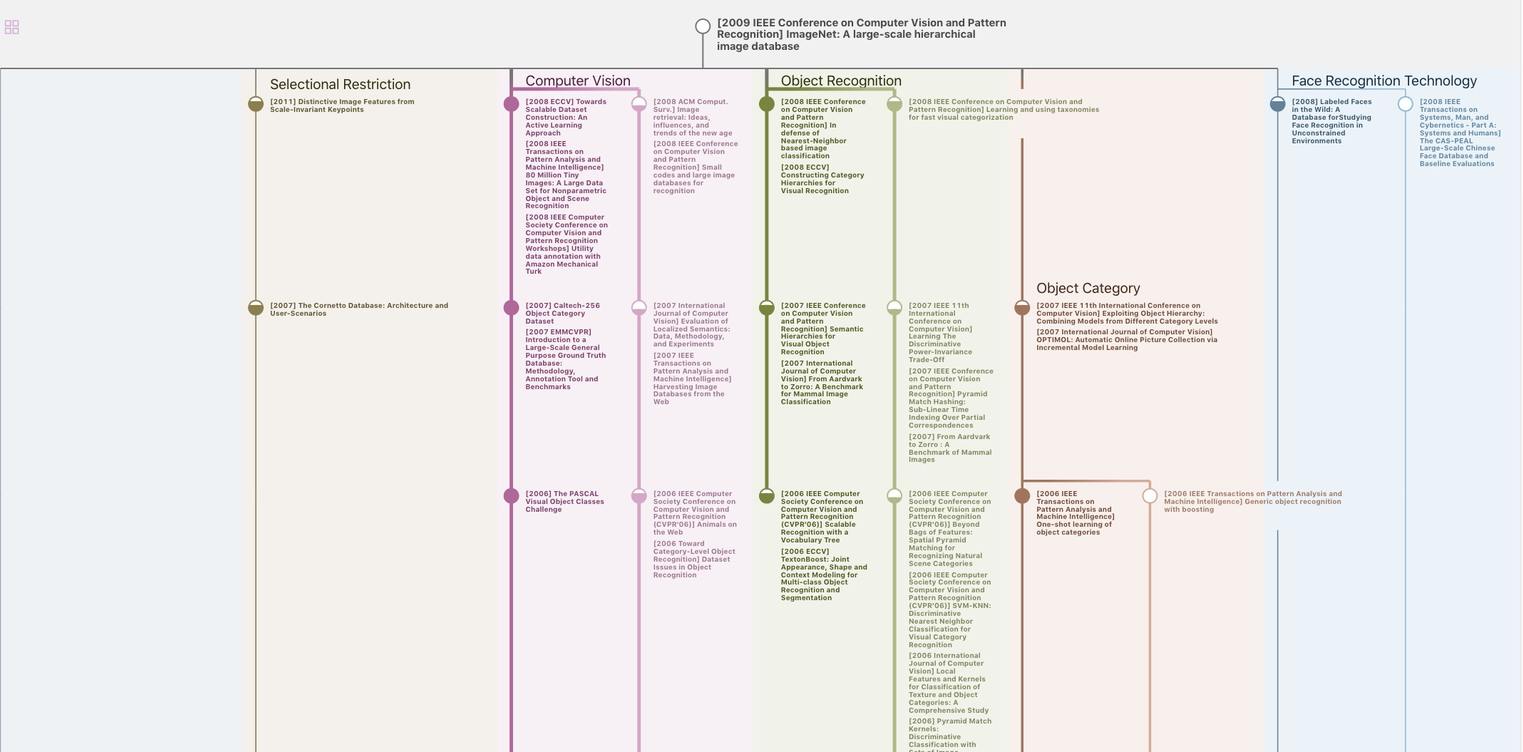
Generate MRT to find the research sequence of this paper
Chat Paper
Summary is being generated by the instructions you defined