Multi-view Graph Convolutional Network with Comprehensive Structural Learning: Enhancing Dynamics Representation for Industrial Processes
Journal of Process Control(2024)
摘要
Quality variable prediction is crucial for improving product quality and ensuring safety for industrial processes. Recently, researchers have explored the application of graph neural networks (GNNs) for this task, leveraging process knowledge encoded in graphs. GNN-based methods have demonstrated high prediction accuracy and partial interpretability. However, these methods typically consider only one type of prior graph and fail to utilize the multi-view prior graphs that coexist in the same process. This knowledge bias prevents effective representation learning about process dynamics, leading to inconsistencies with true process dynamics and overfitting. Thus. their practical applications are limited, especially under scenarios of limited data availability. To address this, a multi-view graph convolutional network with information short (MVGCN-IS) framework is proposed. MVGCN-IS comprises three key components: multi-view graph utilization, multi-view graph fusion, and information shortcut. First, multi-view prior graphs are integrated through multiple pre-trained preliminary GCNs to extract view-specific node representations. Then, a multi-view fusion module aggregates node representations from different views into unified unit representations, capturing comprehensive process structural information. Finally, an information shortcut extracts measurement representations and integrates detailed process measurement data to further enhance model performance. The proposed MVGCN-IS framework is validated on a benzene alkylation process and a debutanizer column process, with a special focus on model reliability under small data scenarios. Experimental results demonstrate the superior prediction accuracy and improved reliability of MVGCN-IS, validating its effectiveness in representation learning and capturing process dynamics.
更多查看译文
关键词
Quality prediction,Industrial processes,Representation learning,Graph neural network (GNN),Multi-view knowledge fusion
AI 理解论文
溯源树
样例
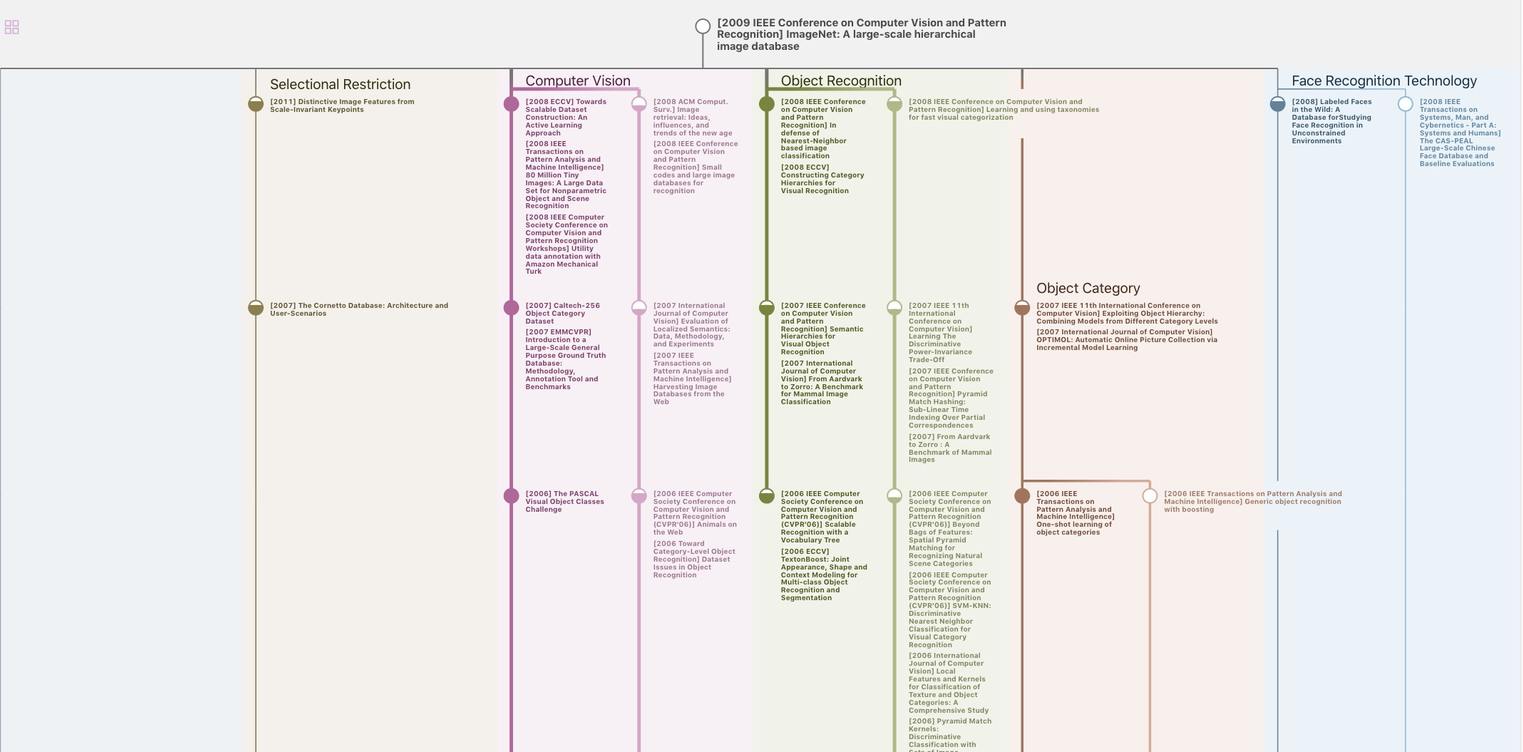
生成溯源树,研究论文发展脉络
Chat Paper
正在生成论文摘要