Fusing Multichannel Autoencoders with Dynamic Global Loss for Self-Supervised Fault Diagnosis
Computers in Industry(2025)
摘要
Engineering fault diagnosis often needs to be implemented without prior knowledge of labels. Considering the randomness and drift of fault features, this paper proposes fusing multichannel autoencoders with dynamic global loss (FMA-DGL) to enhance self-supervised fault diagnosis. Multiple autoencoders are employed to represent the fault features of multichannel vibration signals. A dynamic global loss function is utilized to self-supervise the generation of pseudo-labels, thereby integrating multichannel feature information together. The proposed dynamic global loss controls the degree of conflict of samples from different channels to construct clustering centers, allowing the clustering process to converge more smoothly. By leveraging both the common and complementary information across different channels, the randomness and drift issues of self-supervised pseudo-labels are addressed, effectively enhancing the fault diagnosis performance through multichannel fusion. Experiments were carried out using a public bearing dataset and a rotating machinery experimental setup, respectively. Results show that the proposed FMA-DGL outperforms the state-of-the-art peer methods, exhibiting good results and applicability in self-supervised fault diagnosis based on multichannel vibration signals.
更多查看译文
关键词
Multichannel fusion,Autoencoder,Dynamic global loss,Fault diagnosis,Self-supervised learning
AI 理解论文
溯源树
样例
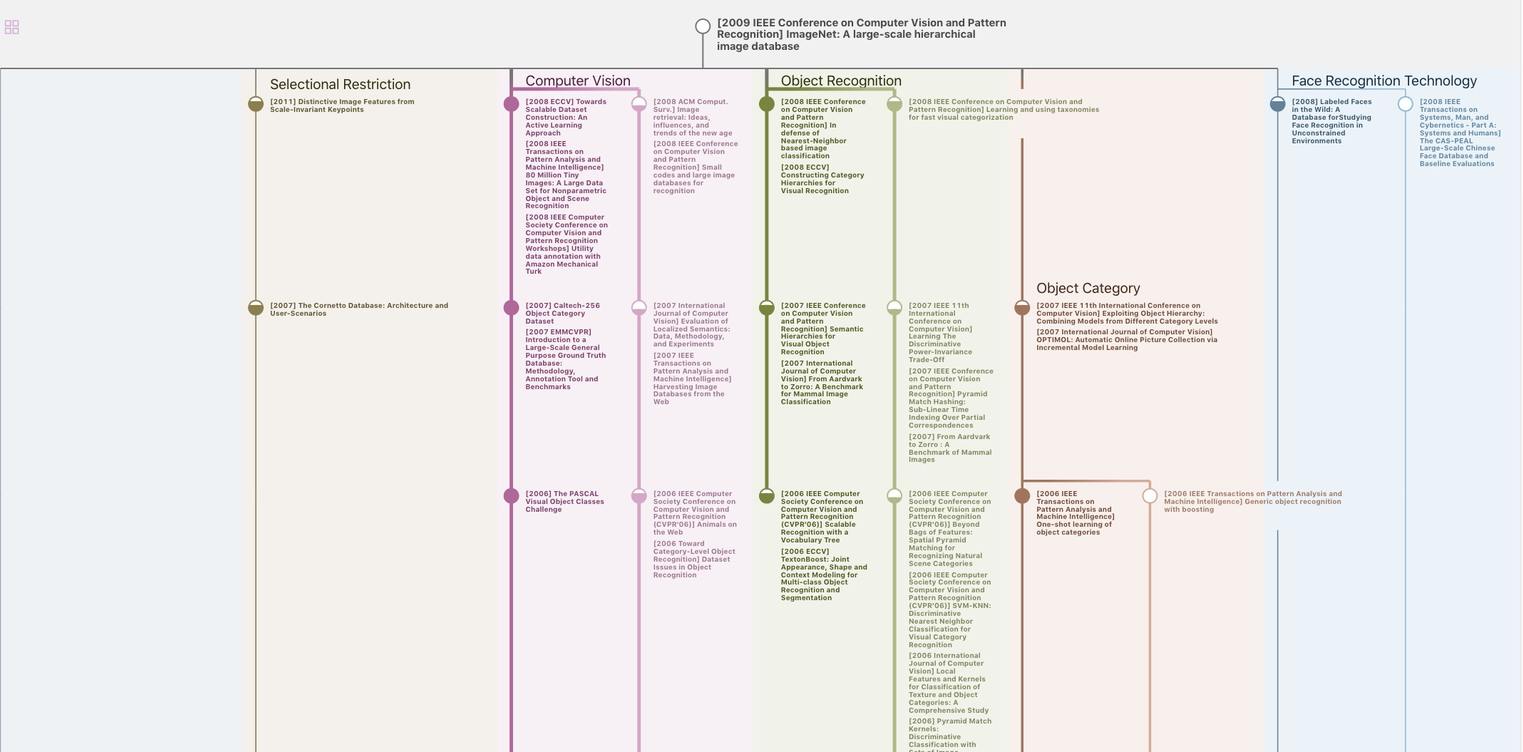
生成溯源树,研究论文发展脉络
Chat Paper
正在生成论文摘要