An Ensemble Learning-Based Transformer for Radar Jamming Recognition with Insufficient Samples
IGARSS 2024 - 2024 IEEE International Geoscience and Remote Sensing Symposium(2024)
摘要
Radar jamming recognition is a fundamental step for anti-jamming techniques. However, the recognition performance is impeded in insufficient samples situation. Ensemble learning provides an effective way to address this issue. In this paper, first, a novel ensemble learning-based radar Transformer (i.e., RadarTR-E) is proposed to improve recognition performance with insufficient samples. Specifically, it votes on the predictions among sub-recognizers to increase recognition accuracy, where RadarTR is employed to effectively capture long-range dependencies. Then, a dynamic label smoothing method (i.e., RadarTR-E-DLS) is further proposed to mitigate overfitting. In detail, dynamic soft labels are designed to prevent overconfidence towards certain radar jamming types. Therefore, the proposed RadarTR-E-DLS achieves better recognition accuracy. Compared with other advanced methods, the experimental results show the superior recognition performance of the proposed methods.
更多查看译文
关键词
Recognition,ensemble learning,dynamic label smoothing,radar jamming
AI 理解论文
溯源树
样例
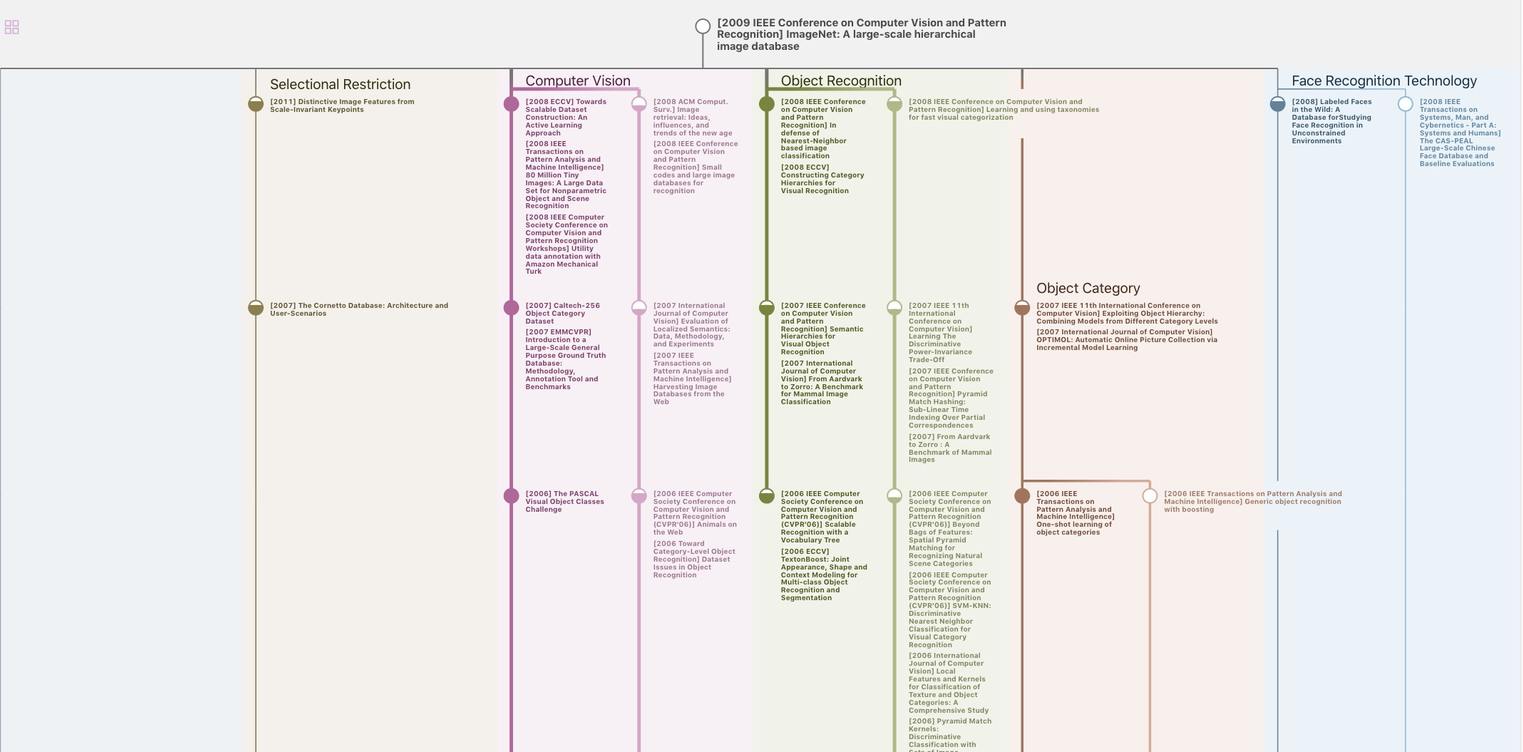
生成溯源树,研究论文发展脉络
Chat Paper
正在生成论文摘要