A Heuristic Framework for Sources Detection in Social Networks Via Graph Convolutional Networks
IEEE Transactions on Systems, Man, and Cybernetics Systems(2024)
摘要
The rapid development of social networks has given opportunities for rumors to disturb the order of society. However, due to the diversity and complexity of users and information dissemination dynamics, localizing the rumor sources in social networks is still a critical and crucial problem yet to be well solved. Recent years, although several methods have been proposed to attempt to solve this problem, they suffer from the contradiction between accuracy and model complexity. To detect information sources efficiently, this article propose a heuristic framework for sources detection (HFSD) in social networks via graph convolutional networks which handles three major challenges, including: 1) the diversity and complexity of users and information dissemination dynamics; 2) difficulty to detect multiple sources, especially without knowing the number of sources; and 3) the class imbalance problem caused by the large differences in sample size of sources and nonsources. Specifically, first, to counteract the diversity and complexity of users and information, different kinds of features of users and information are encoded in the raw feature vectors; second, to address multisource detection, we adopt a binary classification in the last layer of model, which is different from the $n$ -classification methods that are always applied to single-source scenario; finally, to solve the class imbalance problem, we design a balance mechanism which offsets the differences in sample size between the sets of sources and nonsources. Extensive experiments conducted on 12 real-world datasets demonstrate that HFSD can handle problems mentioned above and outperforms than state-of-the-art algorithms significantly.
更多查看译文
关键词
Class imbalance,graph convolutional networks (GCNs),information spreading,sources detection,user features
AI 理解论文
溯源树
样例
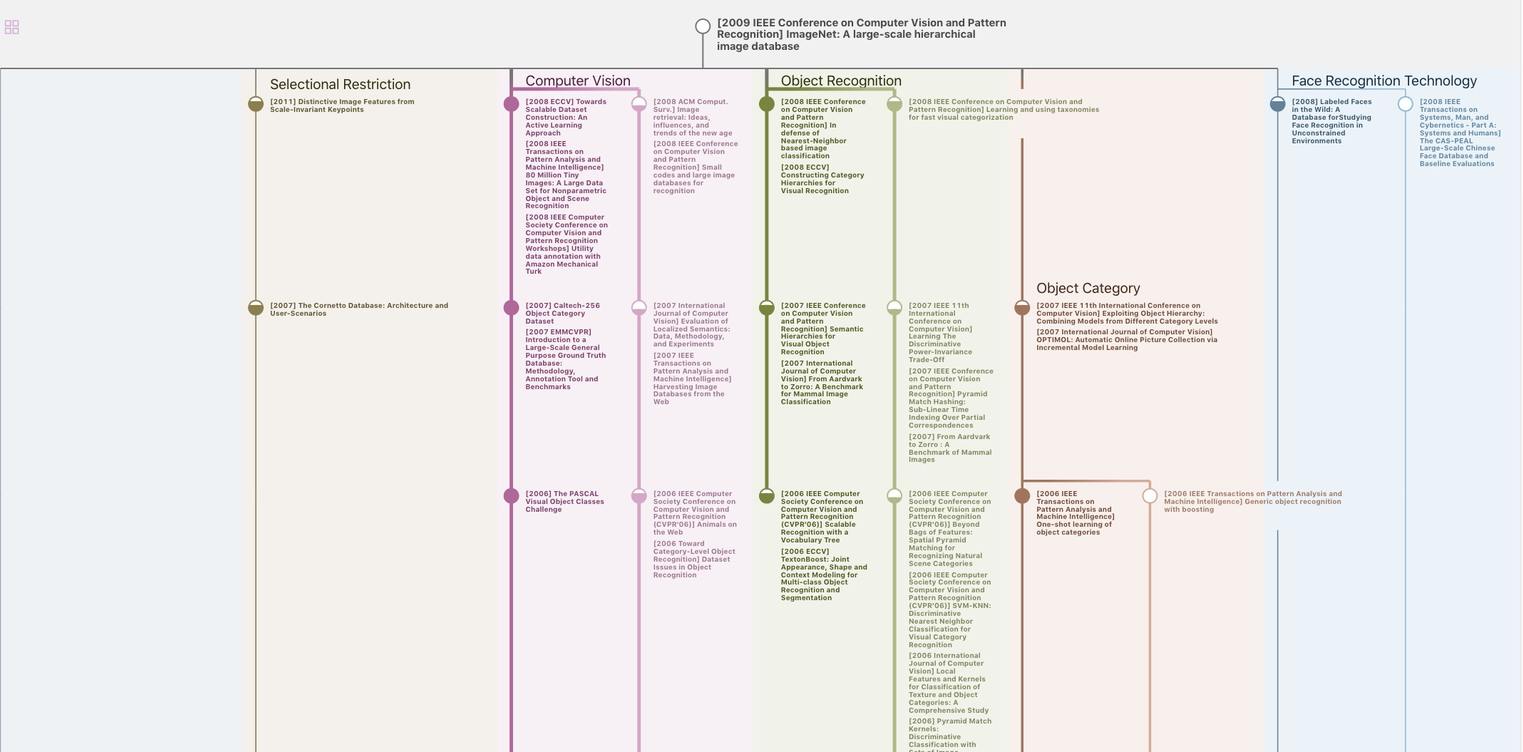
生成溯源树,研究论文发展脉络
Chat Paper
正在生成论文摘要