Multi-Layer Soil Moisture Estimation Using Combined L-and P-Band Radiometry: an Application of Machine Learning Algorithms
IGARSS 2024 - 2024 IEEE International Geoscience and Remote Sensing Symposium(2024)
Abstract
Understanding the vertical distribution of soil moisture is crucial for making informed decisions in various applications, ranging from precision agriculture to hydrological modeling. Four machine learning algorithms, including random forest, extreme gradient boosting, deep learning, and support vector regression were employed to estimate the soil moisture profile from collected tower-based L-band and P-band brightness temperature observations in Victoria, Australia. The results showed that random forest outperformed the other algorithms, with root mean square error (RMSE) values of 0.03, 0.04, and 0.06 m 3 /m 3 for depths of 0-5 cm, 0-30 cm, and 0-60 cm, respectively
MoreTranslated text
Key words
Soil moisture profile,machine learning,P-band,radiometry
AI Read Science
Must-Reading Tree
Example
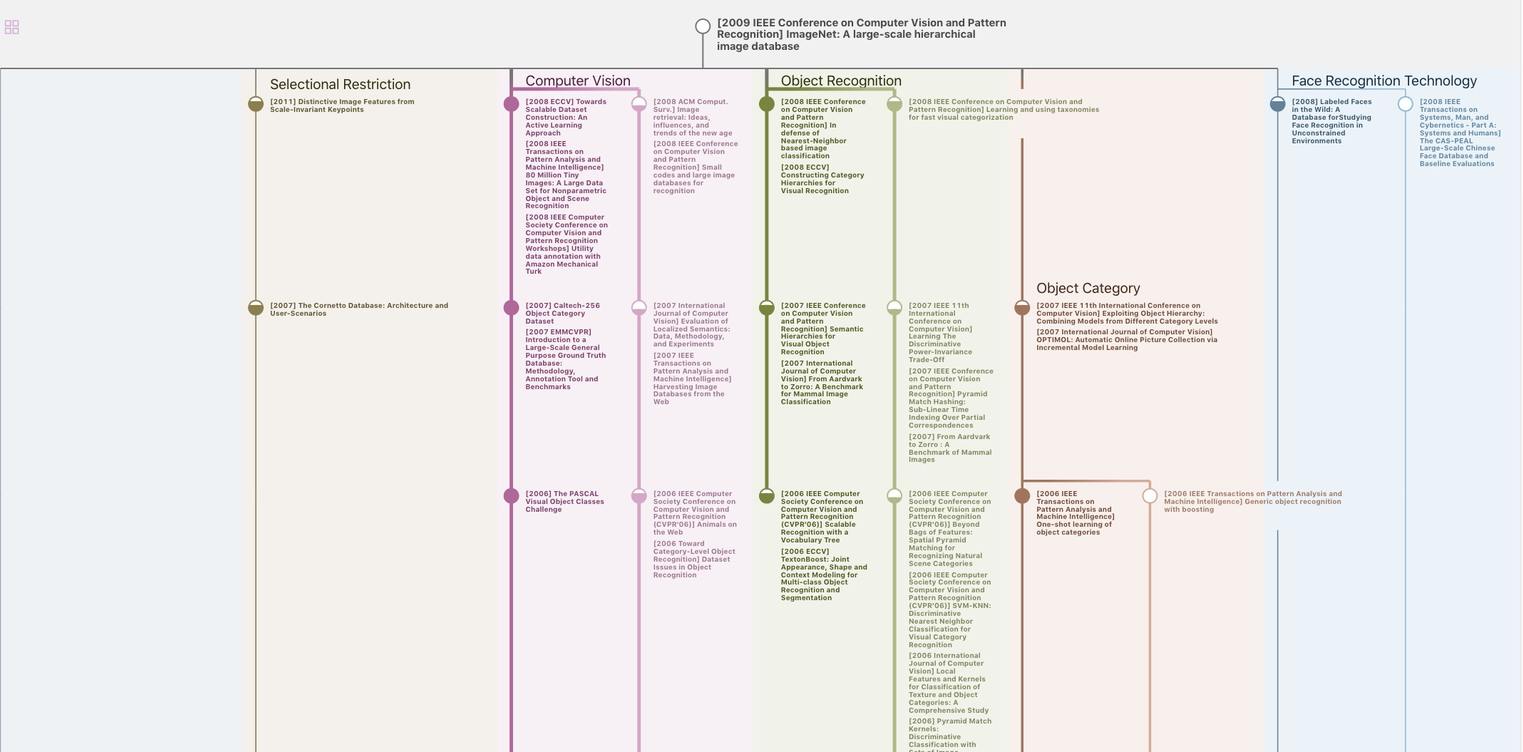
Generate MRT to find the research sequence of this paper
Chat Paper
Summary is being generated by the instructions you defined