T&Amp;A-DEM-California: A Large-Scale Dem Elevation Error Prediction Dataset Based on Tandem-X and AW3D30 DEMs in California, U.S.A.
IGARSS 2024 - 2024 IEEE International Geoscience and Remote Sensing Symposium(2024)
摘要
High-precision Digital Elevation Model (DEM) is crucial in geoscience, ecology, agriculture, hydrology, and other applications. In recent years, the rise of artificial intelligence algorithms such as machine learning has provided a new way to obtain high-precision DEM. However, machine learning algorithms rely on a large amount of training data, but there is currently a lack of open, unified, large-scale, and standardized multi-source DEM elevation error prediction data sets for large areas. Based on this, this paper proposes an open-source dataset, which is a large-scale elevation error prediction dataset based on TanDEM-X and AW3D30 DEMs in California, USA (T&Amp;A-DEM-California). The dataset consists of 10 feature attributes, contains 760,000 samples, and covers an area of 423,970,000 square kilometers. T&Amp;A-DEM-California not only provides academic researchers with benchmark datasets for the validation of new algorithms for machine learning but also provides a research base for future DEM calibration.
更多查看译文
关键词
Digital Elevation Model (DEM),dataset,machine learning,calibration
AI 理解论文
溯源树
样例
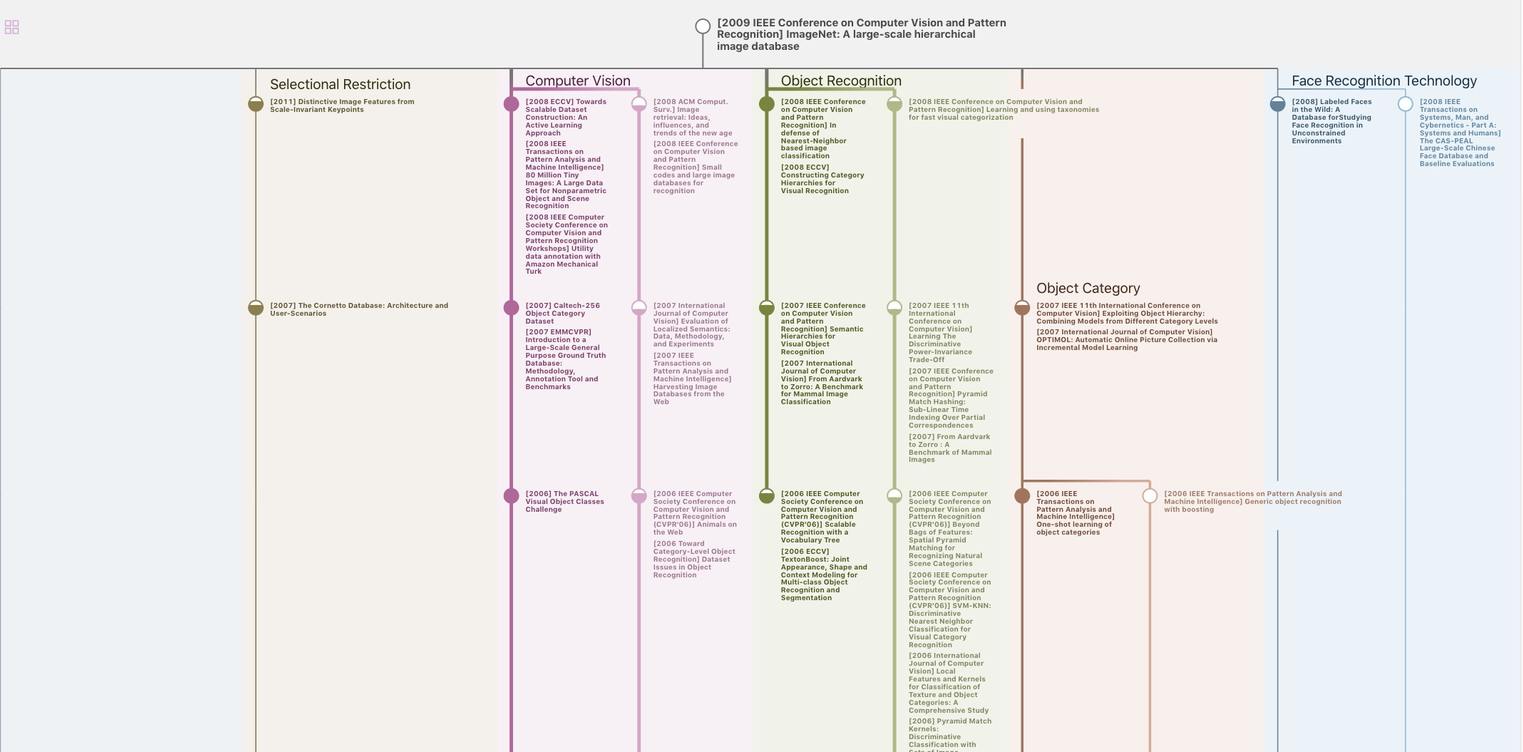
生成溯源树,研究论文发展脉络
Chat Paper
正在生成论文摘要